티스토리 뷰
Paper/Vocoder
[Paper 리뷰] WaveFM: A High-Fidelity and Efficient Vocoder based on Flow Matching
feVeRin 2025. 3. 30. 12:44반응형
WaveFM: A High-Fidelity and Efficient Vocoder based on Flow Matching
- Flow Matching은 diffusion model에 대한 robust training을 제공하지만 neural vocoder에 directly applying 하면 audio quality가 저하됨
- WaveFM
- Standard Gaussian prior 대신 mel-conditioned prior distribution을 채택하여 transportation cost를 minimize
- Refined multi-resolution STFT loss를 결합하여 audio quality를 향상
- 추가적으로 inference speed 향상을 위해 consistency distillation method를 적용
- 논문 (NAACL 2025) : Paper Link
1. Introduction
- Real-time high-fidelity speech synthesis를 위해 Flow-based, Generative Adversarial Network (GAN)-based, Diffusion-based model과 같은 non-autoregressive model이 주로 활용됨
- Flow-based model은 WaveFlow, WaveGlow와 같이 selected prior distribution에서 waveform을 생성하기 위해 invertible nerual network를 활용하여 log-likelihood를 estimate 하는 방식을 사용함
- BUT, invertibility와 determinant evaluation으로 인해 flexibility의 한계가 있음 - MelGAN, HiFi-GAN, BigVGAN과 같은 GAN-based model은 large receptive field와 다양한 discriminator를 활용해 high-fidelity speech를 생성할 수 있음
- 한편으로 DiffWave, BDDM, FreGrad 등은 Denoising Diffusion Probabilistic Model (DDPM)에 기반한 parameter-free noise-adding diffusion process를 활용하여 high-fidelity speech를 생성함
- BUT, diffusion model은 training 중에 discriminator와 같은 auxiliary network가 필요하지 않다는 장점이 있지만 inference phase가 상당히 time-consuming 하다는 단점이 있음
- 한편으로 Consistency Model은 각 step에서 Probability Flow-Ordinary Differential Equation (PF-ODE)의 endpoint를 directly predict 하는 방식으로 single-step inference를 지원할 수 있음
- 즉, ODE trajectory를 따라 align 하여 higher distillation efficiency를 달성할 수 있으므로 entire ODE를 numerically solve 할 필요가 없음
- Flow-based model은 WaveFlow, WaveGlow와 같이 selected prior distribution에서 waveform을 생성하기 위해 invertible nerual network를 활용하여 log-likelihood를 estimate 하는 방식을 사용함
-> 그래서 consistency distillation을 통해 efficient diffusion-based speech synthesis를 지원하는 WaveFM을 제안
- WaveFM
- Mel-spectrogram에 대한 appropiratly conditioned distribution을 활용하여 sample quality를 개선
- Waveform을 directly predict 하는 reparameterized flow matching method를 채택하고 auxiliary loss를 적용해 model 성능을 향상
- 특히 phase angle에 대한 model output을 supervise 하는 multi-resolution phase loss를 integrate 함
- Gradient, Laplacian operator를 real/generated spectrogram에 적용해 spectrogram에서 edge detail과 structural pattern을 효과적으로 반영함
- 추가적으로 tailored consistency distillation method를 통해 audio quality는 유지하면서 inference speed를 further accelerate
< Overall of WaveFM >
- Consistency Distillation과 Flow Matching을 활용한 efficient, high-fidelity vocoder
- 결과적으로 기존 diffusion-based model 보다 더 우수한 합성 품질과 빠른 inference speed를 달성
2. Background
- Flow Matching and Rectified Flow Models
- Flow Matching과 Rectified Flow는 similar training objective를 share 하고 diffusion model도 해당 framework 내에서 interpret 될 수 있음
- [Theorem 1]
를xt 에 대한 continuously differentiable random process,t∈[0,1] 를 probability density function이라고 하자. Prior distribution을p(x,t) , ground-truth를x0 이라고 할 때, conditional expectationx1 이 locally Lipschitz라면:E[dxtdt|xt=x]
(Eq. 1)v(x,t)=E[dxtdt|xt=x]
이다. - 그러면 data distribution
의 sample은 prior distributionx1 에서 sampling 한 다음, timex0 에서 initial valuet=0 를 사용하여 다음의 ODE를 solve 하여 얻어짐:x0
(Eq. 2)dxdt=v(x,t) - 여기서 conditional expectation은 simple Mean Square training objective로 나타낼 수 있음:
(Eq. 3)minvE||dxtdt−v(xt,t)||2 - 일반적으로 straight trajectory는 lower transportation cost를 의미하므로, neural network
에 대한 다음 objective로 이어짐:v(x,t)
(Eq. 4)xt=tx1+(1−t)x0,t∈[0,1] - Training process 이후 data sample은 [Theorem 1]에 따라 ODE를 numerically solve 하여 얻어짐:
(Eq. 5)minvE||x1−x0−v(xt,t)||2
- [Theorem 1]
- Consistency Distillation
- Consistency Distillation (CD)는 diffusion model을 distillating 하여 one-step generation을 지원함
- 기존에는 다음의 forward Stochastic Differential Equation (SDE)를 통해 data를 diffuse 함:
(Eq. 6)dx=√2tdw,t∈[ϵ,T]
- ,ϵ=0.002 T=80 - 이때 backward SDE와 PF-ODE는:
(Eq. 7)dx=−2t∇xlogp(x,t)dt+√2tdw
(Eq. 8)dx=−t∇xlogp(x,t)dt - 한편으로 CD에서 time step은 다음과 같이 discretize 됨:
(Eq. 9)ti=ϵ1/ρ+i−1N−1(T1/ρ−ϵ1/ρ)
- : total discretization step 수,N ,ρ=7 i∈{1,...,N} - Forward SDE에 따라,
- CD는
를 sample 하고 pre-trained teacher score network를 사용하여 PF-ODE를 numerically solve 해n∈U{1,2,...,N−1},xtn+1∼N(xclean,t2n+1I) 을 얻음ˆxtn
- 이를 위해 ODE solver를 채택할 수 있음 - CD student network는 any position/time에서 PF-ODE trajectory의 time
에서 endpoint를 predict 하는 것을 목표로 함:t=ϵ
(Eq. 10)fθ(x,t)=cskip(t)x+cout(t)Fθ(x,t)
-cskip(t)=σ2data(t−ϵ)2+σ2data,cout=σdata(t−ϵ)√σ2data+t2
- ,σdata=0.5 : neural networkFθ(x,t) - 그러면 CD의 loss function은:
(Eq. 11)λ(tn)d(fθ(xtn+1,tn+1),fθ−(ˆxtn,tn))
- : scale function,λ(tn) :d(⋅,⋅) distance와 같은 distance functionL2 - Parameter
는 Exponential Moving Average (EMA)를 통해 update 됨:θ−
(Eq. 12)θ−←stopgrad(μθ−+(1−μ)θ)
- : EMA decay rateμ
- CD는
- 기존에는 다음의 forward Stochastic Differential Equation (SDE)를 통해 data를 diffuse 함:
3. Method
- Mel-Conditioned Prior Distribution
- PriorGrad, SpecGrad와 같이 mel-conditioned prior distribution은 diffusion model에서 자주 활용되지만, 실제로는 diffusion training objective stabilizing으로 인해 audio distribution에 approximate 하지 못함
- 대표적으로 PriorGrad는
를 diffusion prior distribution으로 사용하고, 이때 training objective는:N(μ,Σ)
(Eq. 13)xt=√ˉαt(x0−μ)+√1−ˉα2tϵ
(Eq. 14)minϵ(ϵ−ϵ(xt,t))⊤Σ−1(ϵ−ϵθ(xt,t))
- ,x0∼pdata ϵ∼N(0,Σ)
- ,μ=0 : mel-spectrogram에서 derive 된 diagonal matrixΣ - 해당 training process를 stabilize 하기 위해서는 standard deviation을
로 clamp 해야 하므로, prior와 audio distribution 간의 distance가 증가함[0.1,1]
- BUT, [Theorem 1]에 따라 flow matching model training은 analytical form 없이 2개의 marginal distribution에서 sampling을 수행하기만 하면 됨 - 즉, WaveFM은 training stability를 compromise 하지 않고도 smaller variance의 prior distribution을 활용할 수 있음
- 먼저 diagonal
를 가지는 prior distributionΣ 를 choice 하자N(0,Σ) - 이때 raw value가
의 wide range에 span 되어 있으므로 neural network의 input으로 logarithmic mel-spectrogram을 사용함[0,32768]
- Mel-spectrogram은 audio signal의 energy를 capture 하므로 frequency dimension에 대한 summation의 square root는 prior distribution의 standard deviation으로 적합함 - 다음으로
내에 위치시키기 위해[0,1] 로 divide 하여 normalize 하고 linear interpolation을 적용하여 audio shape에 align 함√mel-bands×32768
- Minimum value는 으로 clamp 됨10−3
- 먼저 diagonal
- Mel-spectrogram value는 일반적으로 potential maximum value보다 훨씬 smaller 하므로 standard deviation은 nearly silent region에서
에 approach 할 수 있음10−3
- 결과적으로 이는 WaveFM의 prior distribution이 audio distribution과 closely align 되어 있음을 의미함
- 대표적으로 PriorGrad는
- Training Objective
- (Eq. 5)의 original objective는 random derivative를 estimate 하는 것을 목표로 하므로, mel-spectrogram loss와 같은 auxiliary loss의 incorporation을 어렵게 함
- 나아가 random noise의 periodic pattern distrubing으로 인해 periodic inductive bias를 포함하는 neural network를 사용하지 못함
- 특히 경험적으로 해당 original objective는 sample quality를 저해함 - 반면 WaveFM은 neural network가 random derivative를 predict 하는 대신 noise로부터 audio를 directly generate 하는 것을 목표로 함
- 따라서 논문은 original Mean Square obejctive를 다음과 같이 reparameterize 함:
(Eq. 15)x1−x0=x1−xt1−t,t∈[0,1)
(Eq. 16)v(xt,t)=E[x1−x0|xt]=E[x1|xt]−x−t1−t
(Eq. 17)⇔minv′E||x1−v′(xt,t)||21−t,t∈[0,1) - 이를 기반으로 neural network는 GAN과 유사하게 mel-spectrogram에서 clean audio를 predict 할 수 있고, 다음의 auxiliary loss를 straight-forward add 할 수 있음:
(Eq. 18)minv′(11−tE||x1−v′(xt,t)||2+λ0E[STFTLoss(x1,v′(xt,t))]+λ1E||mel(x1)−mel(v′(xt,t))||1M)
- ,λ0=0.02,λ1=0.02 : mel-spectrogram의 element 수M
- 에서 infinity approaching을 방지하기 위해 coefficient를11−t 에 대해 10으로 설정t∈[0.9,1)
- 따라서 논문은 original Mean Square obejctive를 다음과 같이 reparameterize 함:
- First auxiliary loss는 Parallel WaveGAN의 multi-resolution STFT loss로써, clean/generated audio의 3가지 resolution에 대해 STFT를 적용하여 얻어짐
- 여기서 FFT, hop, window size는 각각
(1024,2048,512),(120,240,50),(600,1200,240) - 한편으로 spectral convergence loss
와 log-STFT magnitude lossLsc 는:Lmag
(Eq. 19)Lsc(x,ˆx)=|||STFT(x)|−|STFT(ˆx)|||F|||STFT(x)|||F
(Eq. 20)Lmag(x,ˆx)=1N||log|STFT(x)||STFT(ˆx)|||1
- : clean/generated audio,x,ˆx : STFT spectrogram의 element 수N
- : 각각 Frobenius norm,||⋅||F,||⋅||1 normL1
- Norm 내의 STFT-shaped matrix에 대한 operator는 element-wise로 동작함 - 결과적으로 total loss function은:
(Eq. 21)STFTLoss(x,ˆx)=13∑3m=1(L(m)sc+L(m)mag)(x,ˆx) - 여기서 original multi-resolution STFT loss는 STFT spectrogram의 magnitude information 만을 사용하므로,
를 phase angle lossLsc 로 replace 함:Lpha
(Eq. 22)ΔP=Phase(STFT(x))−Phase(STFT(ˆx))
(Eq. 23)Lpha(x,ˆx)=||atan2(sinΔP,cosΔP)||1N
- : phase difference를atan2 로 wrapping 하는 역할(−π,π] - 이때 phase angle은 excessively large gradient를 생성할 수 있으므로, squared magnitude가
보다 작은 phase angle loss는 compute 하지 않음1×10−6
- 추가적으로 를 compute 하기 위해 squared magnitude에L(m)mag 의 small constant를 add 하고, FFT, hop, window size를 각각1×10−6 으로 adjust 함(1024,2048,512),(128,256,64),(512,1024,256)
- 여기서 FFT, hop, window size는 각각
- 최종적으로 multi-resolution STFT loss를 더욱 향상하기 위해 clean/generated spectrogram magnitude에 temporal gradient, frequency gradient, Laplacian operator를 적용함:
(Eq. 24)14[−11−22−11],14[−1−2−1121],18[−1−1−1−18−1−1−1−1]
- 이후 각각 로 scale 된 mean squared error를 compute 하여 model이 spectrogram 내에서 regular pattern을 학습하도록 함4,4,2
- 결과적으로 해당 operator를 통해 spectrogram의 edge information에 대한 visibility를 향상하여 finer detail을 효과적으로 capture 할 수 있음 - 실제로 앞선 multi-resolution STFT loss를 적용하면 WaveFM의 one-step generation quality를 개선할 수 있음
- 특히 아래 그림과 같이 original loss를 사용하여 생성된 spectrogram은 WaveFM의 spectrogram에 비해 lower accuracy를 보임 - 추가적으로 second auxiliary loss function으로 mel-spectrogram의 average
loss를 채택해 quality를 향상함L1
- 나아가 random noise의 periodic pattern distrubing으로 인해 periodic inductive bias를 포함하는 neural network를 사용하지 못함
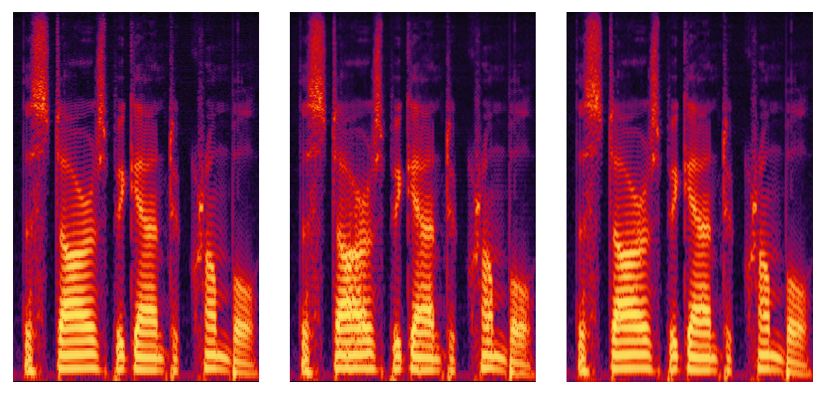
- 한편으로 WaveFM의 reparameterization은 time
에서 diverge 함t=1 - 따라서 논문은 distillation 과정에서
의 range를t 로 restrict 함[0,0.99]
- Waveform이 already sufficiently clean 하므로 large 는 효과적이지 않기 때문t - 결과적으로 WaveFM training은 [Algorithm 1]과 같이 수행됨
- : mel-spectrogramprior(m) 에서 derive 된 diagonal covariance matrixm
- : reparameterizedvθ v′
- 따라서 논문은 distillation 과정에서
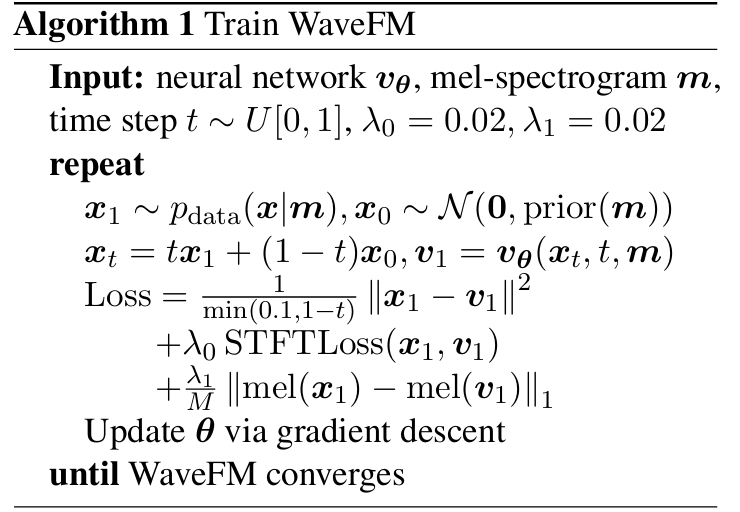
- Distillation Objective
- Numerical ODE solver를 사용하는 기존 inference method는 waveform 생성을 위해 상당한 step이 필요함
- 따라서 논문은 [Algorithm 2]와 같이 SDE에 대한 Consistency Distillation을 도입함
- 먼저 student network는 pre-trained model로 initialize 되고 student network parameter의 EMA를 계산하여 distillation procedure를 stabilizing 하는데 필요한 consistency training target을 생성함
- 이때 distillation process는
가 아닌U(0,0.99) range 내의 truncated[0,0.99] 에서N(0,0.332) 를 sampling 함t
- One-step generation에서 near 의 time step error가 더 critical 하기 때문t=0
- 먼저 student network는 pre-trained model로 initialize 되고 student network parameter의 EMA를 계산하여 distillation procedure를 stabilizing 하는데 필요한 consistency training target을 생성함
- 한편으로 [Algorithm 2]에서 distance function
은 network output과 target 간의 training loss를 의미하고, 앞선 결과와 같이 4개의 term으로 구성됨d(⋅,⋅) 의 경우, any numerical solver를 사용할 수 있음ODESOLVE
- 논문은 Euler method를 채택- 이때 original objective를 reparameterize 하므로 numerical ODE solver에 대한 original function을 reconstruct 할 수 있어야 함
- 논문은 consistency function을 continuously differentiable manner로 parameterizing 하지 않고,
에 close 한 time point에서 clean audio를 target으로 설정한 다음 neural network를 통해 smaller time point의 결과를 predict 함1
- 이는 기존 consistency model과 같이 parameterize 하면 generated audio quality가 degraded 되기 때문 - 결과적으로 WaveFM의 consistency distillation은 dynamic prior distribution과 compatible 하므로, fixed prior distribution에 의존하는 기존 consistency model과는 달리 prior sampling만 필요함
- 따라서 논문은 [Algorithm 2]와 같이 SDE에 대한 Consistency Distillation을 도입함
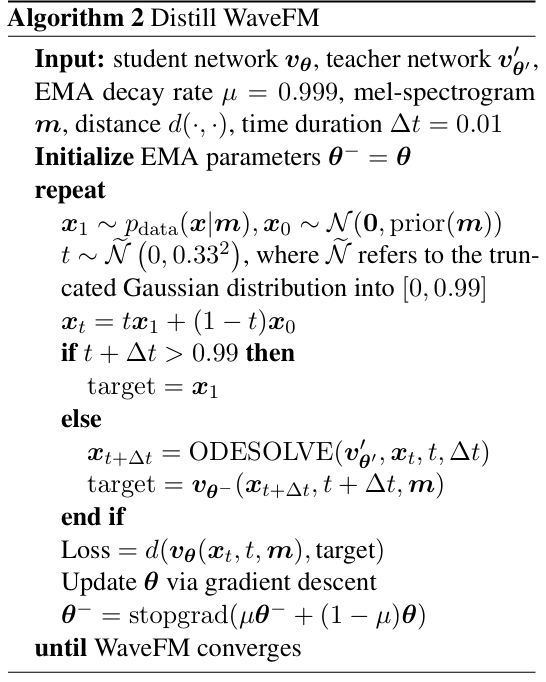
- Network Architecture
- Function
는 19.5M parameter의 asymmetric U-Net model을 기반으로 HiFi-GAN의 multi-receptive filed module을 채택함v(x,t) - ResBlock에서 Conv1D는 'same' padding을 사용하고, 각 ResLayer는 kernel list, dilation list로 정의됨
- 여기서 kernel list, dilation list의 outer product는 ResBlock matrix와 각 ResBlock의 convolutional layer에 대한 kernel width, dilation을 정의함 - Mel-spectrogram은 detailed condition을 제공하므로 neural network는 mel-spectrogram을 upsampling 하고 waveform을 step-by-step refine 해야 함
- 따라서 WaveFM은 U-Net upsampling process를 활용하고 larger kernel size를 가지는 dilated convolutional layer를 채택하여 mel-spectrogram으로부터 audio를 reconstrcut 함
- 반면 downsampling process는 simpler convolutional layer로 구성됨 - 결과적으로 아래 그림과 같이
ResBlock matrix를 포함하는 downsampling ResLayer와4×1 ResBlock matrix를 포함하는 upsampling ResLayer를 구축함3×3
- 따라서 WaveFM은 U-Net upsampling process를 활용하고 larger kernel size를 가지는 dilated convolutional layer를 채택하여 mel-spectrogram으로부터 audio를 reconstrcut 함
- Multi-receptive field module에서 channel-wise log-scale parameter
로 정의된 snake-beta activation을 사용함:α,β
(Eq. 25)snake(x)=x+1eβ+ϵsin2(eαx)
- (Eq. 25)는 BigVGAN을 따름
- : numerical stability를 위해 사용되는 valueϵ=10−8 - Down/upsampling process는 각각 strided/transposed convolution을 사용함
- 해당 architecture를 통해 mel-spectrogram으로부터 waveform을 directly generate 함 - Time representation의 경우
을 100으로 scale 하여, diffusion model과 magnitude를 align 한 다음, 128-dimensional positional encoding vector에 embedding 함:t∈[0,1]
(Eq. 26)[sin(100t⋅100×463),...,sin(100t⋅1063×463),cos(100t⋅100×463),...,cos(100t⋅1063×463)]
- (Eq. 26)은 DiffWave를 따름
- 해당 128-dimensional time embedding은 2개의 linear-SiLU layer를 통해 512-dimension으로 expand 된 다음, 각 resolution을 desired shape로 reshape 하고, downsampling process에서 hidden layer에 add 됨
- ResBlock에서 Conv1D는 'same' padding을 사용하고, 각 ResLayer는 kernel list, dilation list로 정의됨
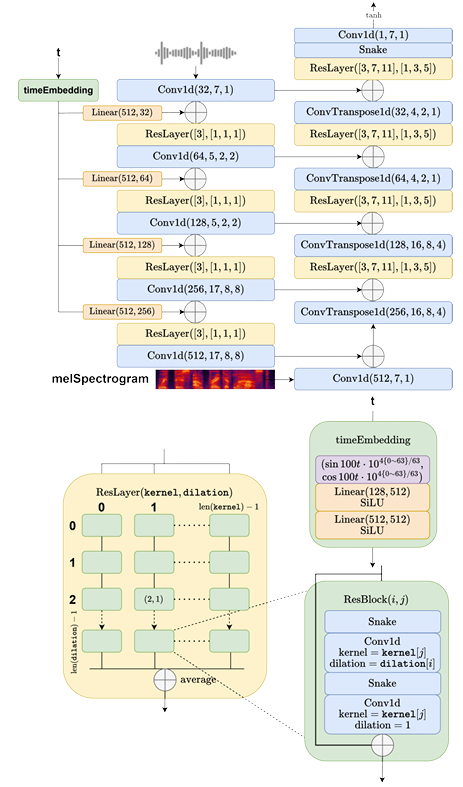
4. Experiments
- Settings
- Dataset : LibriTTS, MUSDB18-HQ
- Comparisons : HiFi-GAN, BigVGAN, DiffWave, PriorGrad, FreGrad, FastDiff
- Results
- LibriTTS dataset에 대해 WaveFM이 가장 뛰어난 성능을 보임
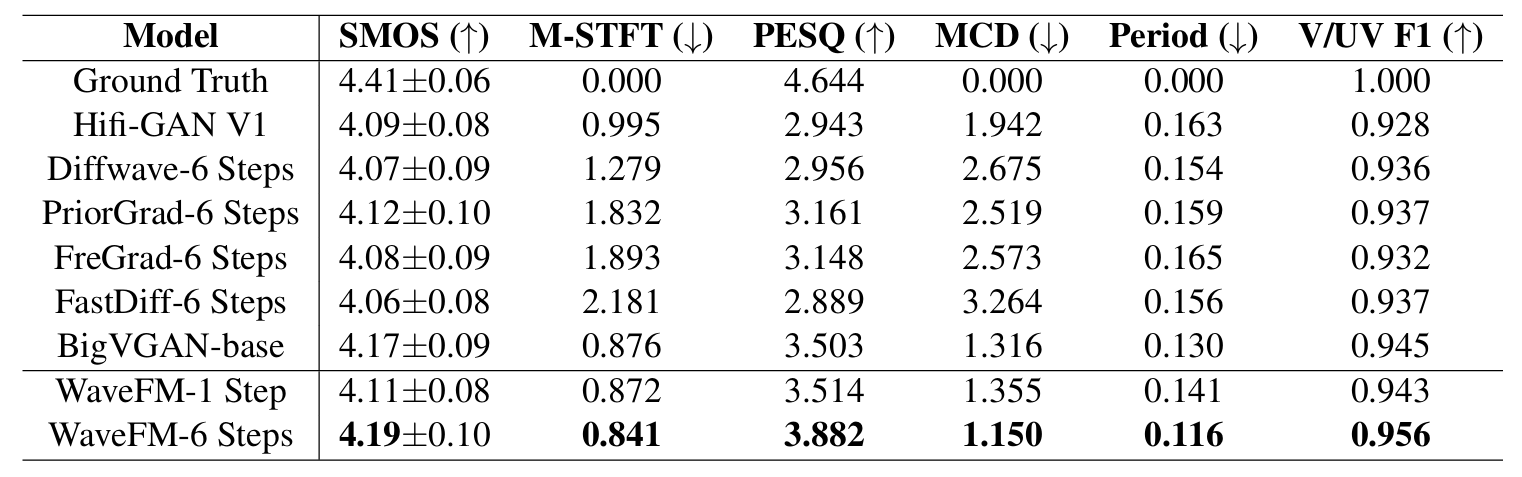
- MUSDB18-HQ dataset에 대해서도 WaveFM이 가장 우수함
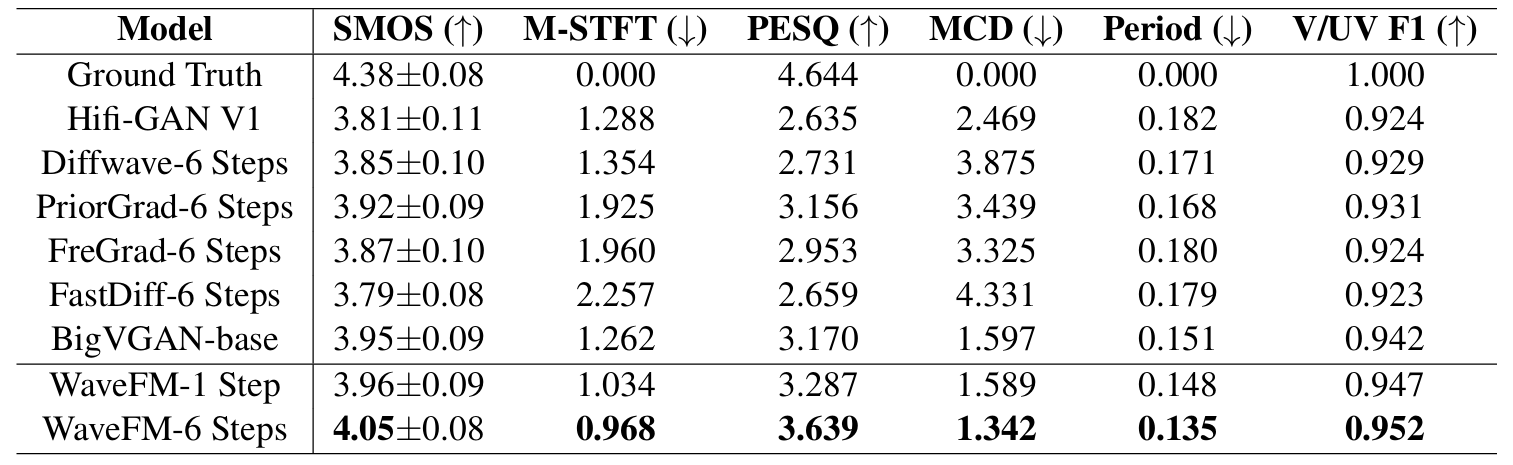
- WaveFM은 HiFi-GAN 수준의 Real Time Factor (RTF)를 달성할 수 있음
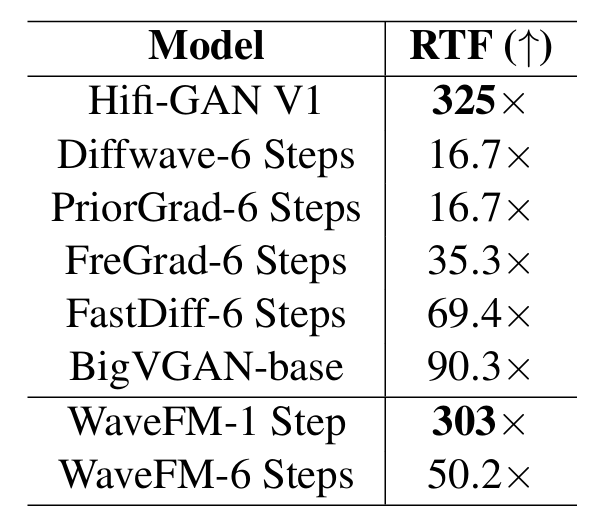
- Out-of-Distribution Situation
- WaveFM의 mel-spectrogram은 ground-truth와 더 closer 함
- 특히 PriorGrad는 low-frequency component를 overestimate 하고 BigVGAN은 clean spectrogram에서 regular component를 generate 하지 못함
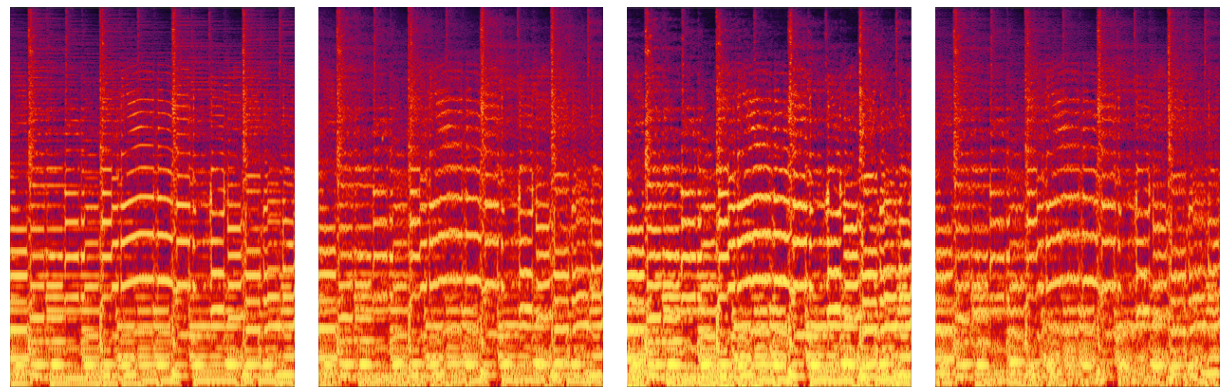
- Ablation Study
- WaveFM의 각 component를 제거하는 경우 성능 저하가 발생함
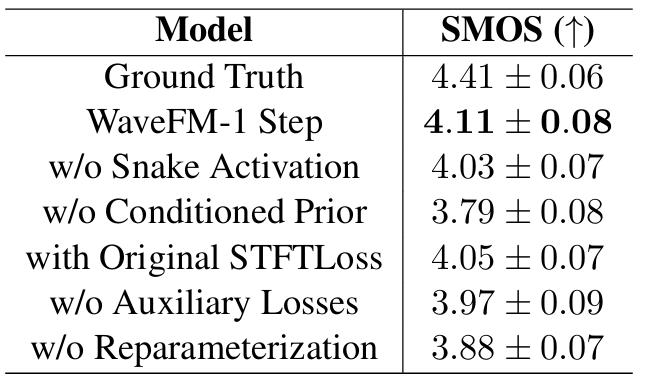
반응형