티스토리 뷰
반응형
Denoising Diffusion Probabilistic Models
- Nonequilibrium thermodynamics에서 영감을 받은 latent variable model인 diffusion probabilistic model을 사용하여 고품질의 이미지 합성을 시도
- Denoising Diffusion Probabilistic Model (DDPM)
- Diffusion probabilistic model과 Langevin dynamics를 연결하는 denoising score matching을 활용
- Autoregressive decoding의 generalization으로 해석될 수 있는 progressive lossy decompression을 허용
- 논문 (NeurIPS 2020) : Paper Link
1. Introduction
- 다양한 생성 모델이 고품질의 이미지 합성 능력을 보여주고 있음
- Generative Adversarial Network (GAN), Autoregressive model, Flow, Variational AutoEncoder (VAE)가 대표적
- 특히 energy-based 모델링과 score matching은 GAN과 비교할만한 합성 품질을 보이고 있음 - 그중 논문은 diffusion probabilistic model을 활용함
- Diffusion model은 finite time 이후에 data와 일치하는 sample을 생성하기 위해, variational inference를 사용하여 학습되는 parameterized Markov chain
- 이때 chanin의 transition은 signal이 destory 될 때까지 sampling의 반대 방향으로 data에 점진적으로 noise를 추가하는 diffusion process를 reverse 하는 방식을 학습함
- Diffusion이 소량의 Gaussain noise로 구성된 경우, sampling chain transition을 conditional Gaussian으로 설정하여 간단한 neural network parameterization이 가능
- 위와 같이 diffusion model은 정의하기 쉽지만, 아직까지 고품질 sample을 합성할 수 있다는 보장이 없음
- 추가적으로 diffusion model의 특정한 parameterization은 여러 noise level에 대한 denoising score matching과 sampling 과정에서의 annealed Langevin dynamics와의 equivalence를 보임
- Generative Adversarial Network (GAN), Autoregressive model, Flow, Variational AutoEncoder (VAE)가 대표적
-> 그래서 denoising score matching과 Lagnevin dynamics를 연결하는 Denoising Diffusion Probabilistic Model을 제시
- Denoising Diffusion Probabilistic Model (DDPM)
- Denoising scroe matching과 Lagnevin dynamics를 연결하여 고품질의 이미지 합성 능력을 달성
- 특히 DDPM의 lossless codelength는 impreceptible image detail을 설명하는 데 사용되는 것으로 나타남
- Lossy compression을 통해 해당 특성에 대한 정교한 분석을 제시하고 diffusion model의 sampling 과정이 autoregressive decoding과 유사하게 generalize 될 수 있음을 보임
< Overall of DDPM >
- Diffusion probabilistic model과 Langevin dynamics를 연결하는 denoising score matching을 활용
- Autoregressive decoding의 generalization으로 해석될 수 있는 progressive lossy decompression을 허용
2. Background
- Diffusion model은
형식의 latent variable modelpθ(x0):= : data 와 동일한 dimensionality의 latents- Joint 분포
는 reverse process라고 하고, 에서 시작하는 learned Gaussian transition을 가지는 Markov chain으로 정의됨:
(Eq. 1) - Diffusion model과 다른 latent variable model 간의 차이점은,
- Forward/diffusion process라고 하는 근사 posterior 가 variance schedule 에 따라 data에 Gaussian noise를 점진적으로 추가하는 Markov chain으로 fix 된다는 것:
(Eq. 2) - Diffusion model의 training은 negative log-likelihood에 대한 variational bound를 최적화하는 것으로 수행됨:
(Eq. 3)
- Forward process variance 는 reparameterization을 통해 학습되거나 hyperparameter로 constant 하게 설정되고,
- Reverse process의 expressiveness는 에서 Gaussian conditional을 선택함으로써 보장됨
- 이는 가 작을 때, 두 process 모두 동일한 functional form을 가지기 때문 - Forward process의 주요한 property는 closed form의 arbitrary timestep
에서 의 sampling을 허용한다는 것:
(Eq. 4)
- , - 따라서 stochastic gradient descent를 사용하여
의 random term을 최적화함으로써 효율적인 training이 가능함
- 즉, (Eq. 3)의 을 아래와 같이 rewriting 하면, variance reduction이 가능:
(Eq. 5) - (Eq. 5)는 KL-divergence를 사용하여
를 에 대해 condition 할 때 tractable forward process posterior를 통해 직접적으로 비교됨:
(Eq. 6)
(Eq. 7) - 결과적으로, (Eq. 5)의 모든 KL-divergence는 Gaussian 간의 비교이므로 Monte Carlo 추정 대신, closed form을 사용하여 Rao-Blackwellized 방식으로 계산할 수 있음
3. Diffusion Models and Denoising Autoencoders
- Diffusion model은 latent variable model의 restricted class로 볼 수 있지만, 구현시 많은 자유도를 허용함
- 따라서 forward process의 variance
와 reverse process의 model architecture, Gaussian 분포 parameterization을 choice 해야 함 - 이러한 choice를 guide 하기 위해, diffusion model에 대한 simplified, weighted variational bound objective와 denoising score matching 간의 explicit 한 연결을 설정
- 이를 위해 (Eq. 5)의 각 term을 categorize 하여 이를 유도함
- 따라서 forward process의 variance
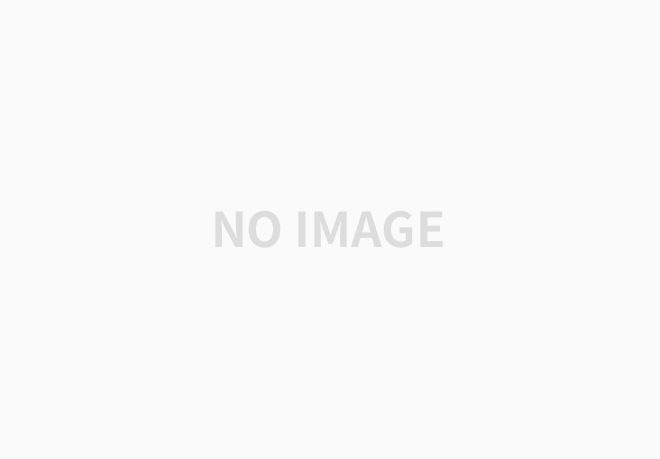
- Forward Process and
- DDPM은 forward process variance
가 reparameterization을 통해 learnable 하다는 점을 무시하고, constant로 fix 함- 따라서 근사 posterior
에는 learnable parameter가 존재하지 않고 는 constant 이므로, training 시 무시 가능함
- 따라서 근사 posterior
- Reverse Process and
- 이를 기반으로
에 대해 에서의 choice를 살펴보면, 를 untrained time dependent constant라고 했을 때, 와 모두 유사한 결과를 보임
- 이때 첫 번째 choice는 에 optimal 하고 두 번째 choice는 deterministic 한 하나의 point로 설정된 에 optimal 함
- 이는 coordinatewise unit variance가 있는 data에 대한 reverse process entropy의 uppper/lower bound에 해당- 따라서 평균
를 나타내기 위해 아래의 analysis를 기반으로 specific parameterization을 제안- 먼저
에 대해, 다음과 같이 나타낼 수 있음:
(Eq. 8)
- : 에 nondependent 한 constant
- 여기서 의 straightforward parameterization은 forward process posterior 평균인 를 예측하는 모델 - 이때 (Eq. 4)를
로 reparameterization 하고 forward process posterior formula (Eq. 7)을 적용하여 (Eq. 8)을 확장할 수 있음:
(Eq. 9)
(Eq. 10)
- (Eq. 10)은 가 주어진 에서 를 예측해야 하는 것을 나타냄 는 model input으로 사용할 수 있으므로, parameterization을 choice 할 수 있음:
(Eq. 11)
- : 로부터 을 예측하는 function approximator
- 이때 를 sampling 하는 것은, 에서 를 계산하는 것과 같음
- 먼저
- [Algorithm 2]의 전체 sampling 과정은 data density의 learned gradient로써
를 사용하는 Langevin dynamics와 같음- 추가적으로 (Eq. 11)의 reparameterization을 사용하면 (Eq. 10)은 아래와 같이 simplify 됨:
(Eq. 12) - 이는
로 index 된 여러 noise scale에 대한 denoising score matching과 유사함
- 다시 말해, (Eq. 12)는 Langevin-like reverse process (Eq. 11)에 대한 variational bound와 같음
- 따라서 denoising score matching과 유사한 objective를 최적화하는 것은, Langevin dynamics와 유사한 sampling chain의 finite-time margnial을 fit 하기 위해 variational inference를 사용하는 것과 equivalent 함
- 추가적으로 (Eq. 11)의 reparameterization을 사용하면 (Eq. 10)은 아래와 같이 simplify 됨:
- 요약하자면, reverse process mean function approximator
를 training 하여 를 예측하거나, parameterization을 수정하여 을 예측하도록 training 할 수 있음
- 이때 -prediction parameterization은 Langevin dynamics와 유사하므로, denoising score matching과 유사한 objective에 대해 diffusion model의 variational bound를 simplify 할 수 있음
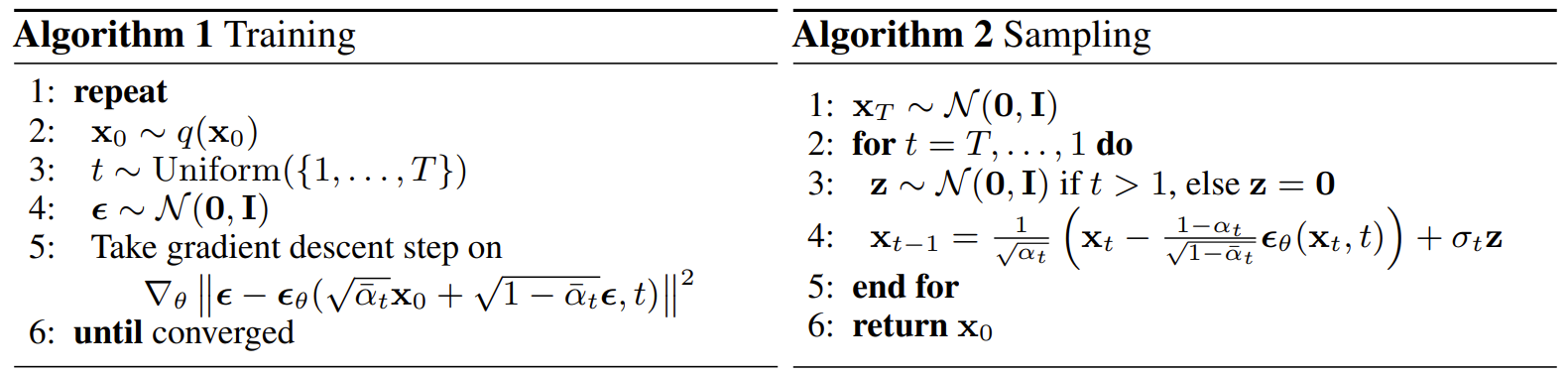
- Data Scaling, Reverse Process Decoder, and
- 이미지 data는
로 linearly scale 된 의 interger로 구성된다고 하자- 이는 neural network의 reverse process가 standard normal prior
에서 시작하여 consistently scaled input에서 동작하도록 함 - Discrete log-likelihood를 얻기 위해, reverse process의 마지막 term을 Gaussian
에서 파생된 independent discrete decoder로 설정:
(Eq. 13)
- ,
- : data dimensionality, : extraction of one coordinate - 위 방식은 data에 noise를 추가하거나 scaling의 Jacobian을 log-likelihood에 통합할 필요 없이, variational bound가 discrete data의 lossless codelength 임을 보장함
- 이는 VAE decoder나 Autoregressive model에서 사용되는 discrete continuous 분포와 유사함
- 결과적으로 sampling이 끝나면, 을 noiselessly 하게 나타낼 수 있음
- 이는 neural network의 reverse process가 standard normal prior
- Simplified Training Objective
- 앞서 정의된 reverse process와 decoder를 사용하면, (Eq. 12)와 (Eq. 13)에서 파생된 term으로 구성된 variational bound는
에 대해 differentiable 하므로 trainable 함- 이때 아래의 variational bound의 variant를 사용하는 것이 sample 품질에 더 유리하고 더 간단함:
(Eq. 14)
- 는 범위에 대해 uniform 함 인 경우, 과 edge effect를 무시하고 Gaussian probability density function과 bin width의 곱으로 근사된 discrete decoder (Eq. 13)의 적분을 가지는 에 해당함 인 경우, NCSN denoising score matching model에서 사용하는 loss weighting과 유사한 (Eq. 12)의 unweighted version에 해당함
- Forward process variance 가 fix 되어 있으므로 는 나타나지 않음
- 결과적으로 [Algorithm 1]은 이러한 simplified objective를 사용하여 training을 수행함
- 이때 아래의 variational bound의 variant를 사용하는 것이 sample 품질에 더 유리하고 더 간단함:
- Simplified objective (Eq. 14)는 (Eq. 12)의 weight를 제거하므로 standard variational bound와 비교하여 reconstruction의 다양성을 증가시킬 수 있음
- 특히 diffusion process 설정은 작은
에 해당하는 down-weight loss term에 대한 simplified objective를 제공 - 이러한 term은 아주 적은 양의 noise로 data를 denoising 하도록 network를 학습시키므로, network가 더 큰
term에서 더 어려운 denoising 작업에 집중할 수 있도록 down-weight 하는 것이 좋음 - 결과적으로 이러한 reweighting을 통해 DDPM은 더 우수한 sample 품질을 얻을 수 있음
- 특히 diffusion process 설정은 작은
4. Experiments
- Settings
- Dataset : CIFAR10, LSUN, CelebA-HQ
- Comparisons : EBM, JEM, BigGAN, StyleGAN, Gated PixelCNN, Sparse Transformer, PixelIQN, NCSN, SNGAN
- Results
- Sample Quality
- CIFAR10에 대한 Inception score, FID, negative log-likelihood (lossless codelength)를 비교해 보면
- DDPM이 가장 우수한 성능을 보이는 것으로 나타남
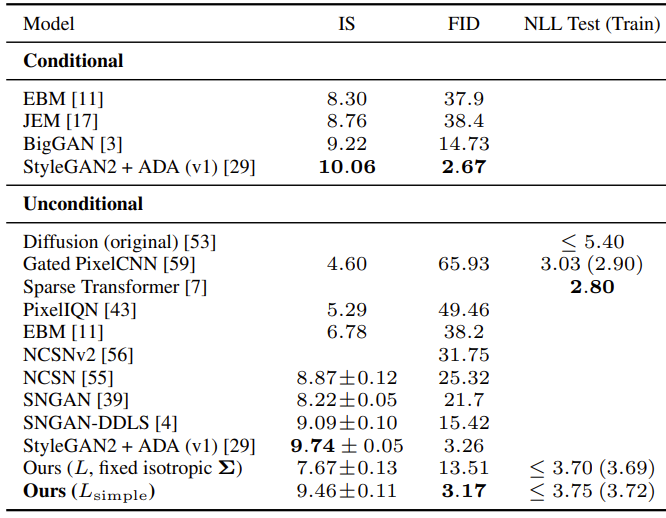
- 특히 simple objective
을 사용한 DDPM은 true variational bound에 대해 더 나은 codelength를 생성하고 더 우수한 합성 품질을 달성함
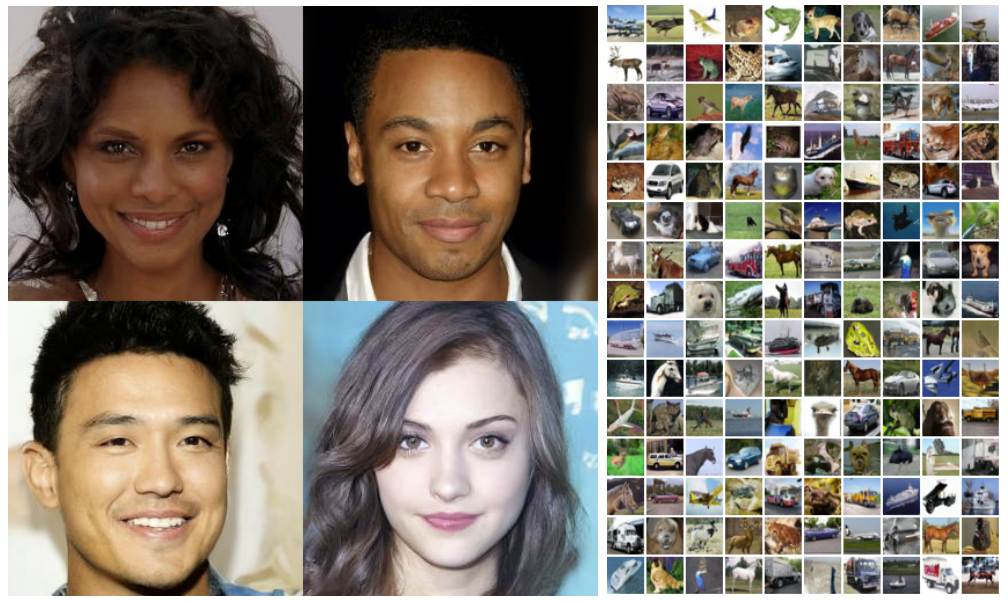
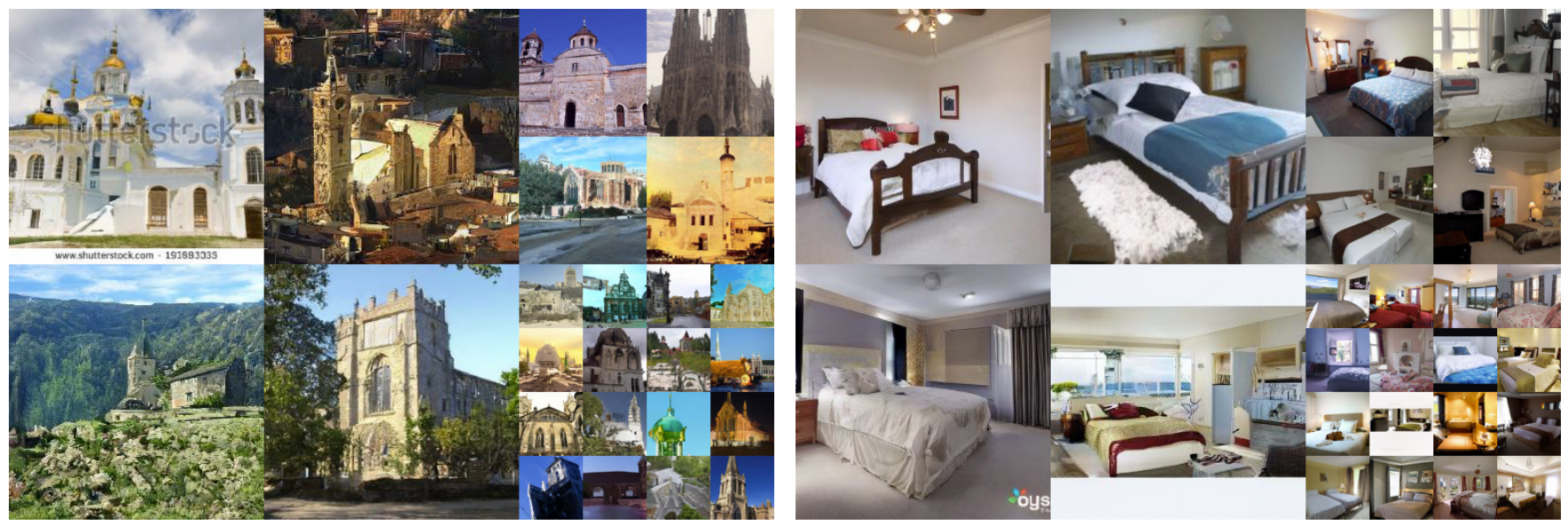
- Reverse Process Parameterization and Training Objective Ablation
의 예측은 (Eq. 14)의 unweighted mean squared error 대신 true variational bound에 대해 학습될 때만 잘 작동함- Reverse variance를 학습하면 fixed variance에 비해 학습이 unstable 하고 품질이 저하되는 것으로 나타남
- 따라서 논문에서 제안한 바와 같이, fixed variance와 simplified objective를 사용하면 더 우수한 성능을 얻을 수 있음
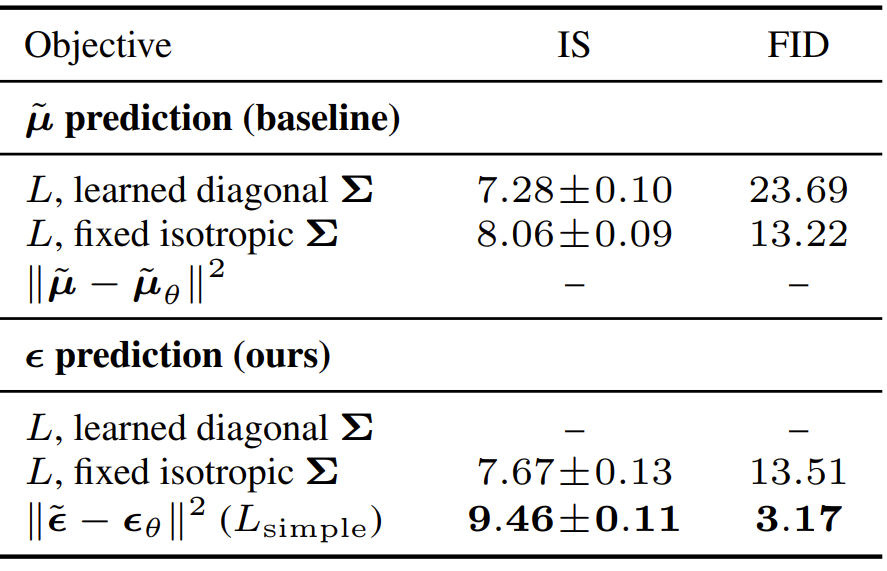
- Progressive Coding
- DDPM의 lossless codelength는 energy-based model과 annealed importance를 사용하는 score matching 방법들 보다는 좋은 결과를 보이지만, 다른 likelihood-based model 보다는 경쟁력이 떨어짐
- 그럼에도 불구하고 DDPM은 우수한 합성 품질을 보이기 때문에, diffusion model에는 lossy compressor를 만드는 inductive bias가 존재한다고 볼 수 있음 - Variational bound term
를 rate로 를 distortion으로 처리하면, DDPM은 CIFAR10에 대해 1.78 bits/dim을 보임
- 이는 0에서 255까지의 범위에서 0.95의 mean squared error에 해당함
- 다시 말해, lossless codelength의 절반 이상은 imperceptible distortion을 나타냄
- DDPM의 lossless codelength는 energy-based model과 annealed importance를 사용하는 score matching 방법들 보다는 좋은 결과를 보이지만, 다른 likelihood-based model 보다는 경쟁력이 떨어짐

- Progressive Lossy Compression
- (Eq. 5)를 반영하는 progressive lossy code를 DDPM에 도입하여 rate-distortion behavior를 조사
- 이때 receiver는 모든
에서 partial information 를 가지고 아래와 같이 점진적으로 추정할 수 있음:
(Eq. 15) - 결과적으로 CIFAR10에 대한 rate-distortion plot을 확인해 보면, distortion은 low-rate region에서 급격하게 감소하는 것으로 나타남
- 이는 대부분의 bit가 imperceptible distortion에 할당되었다는 것을 의미
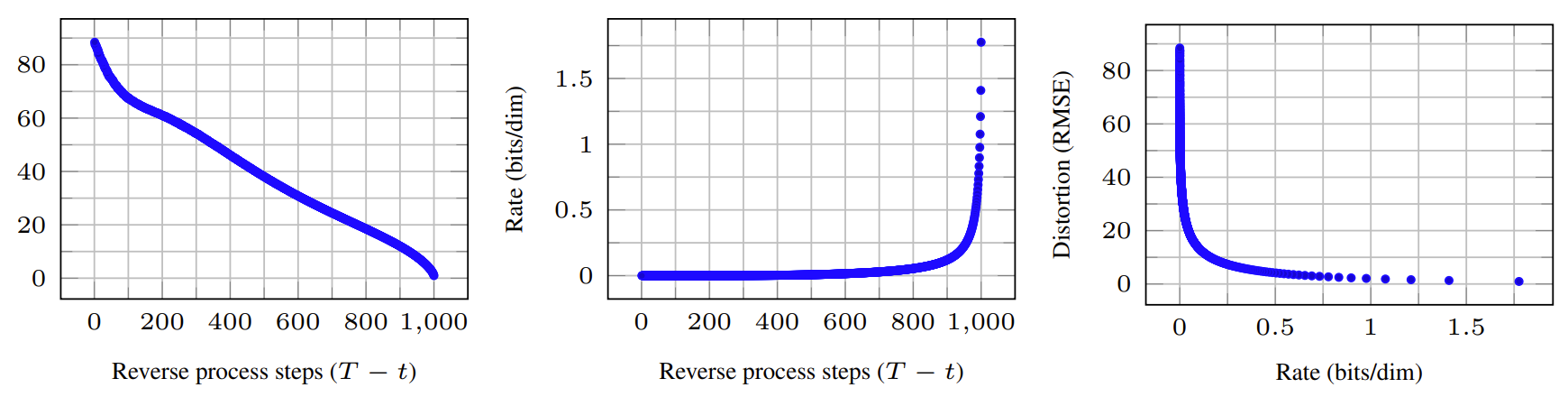
- Progressive Generation
- Random bit의 progressive decompression을 통한 unconditional generation process를 실험해 보면
- Large scale의 이미지 feature가 가장 먼저 나타나고 detailed feature는 나중에 나타남
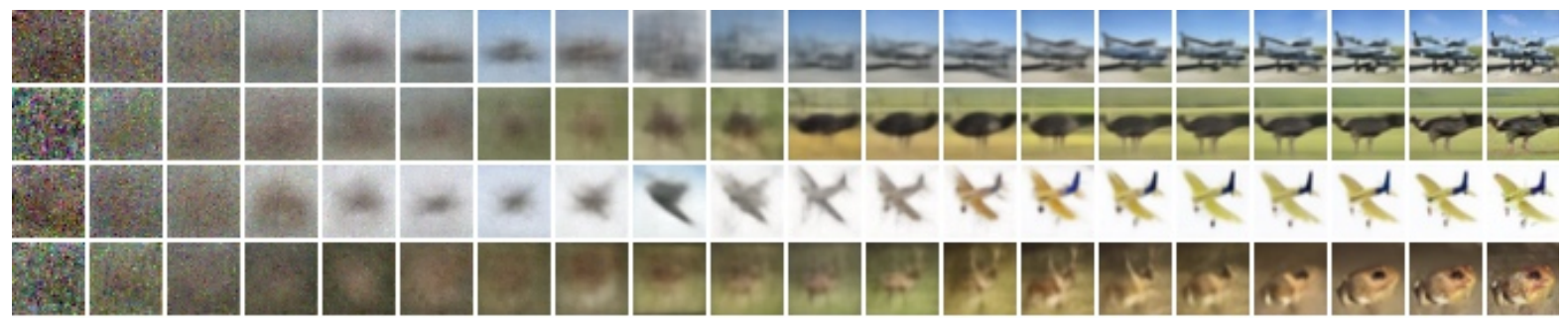
에 대해 를 frozen 했을 때 의 결과를 확인해 보면, 가 작으면 fine detail을 제외한 나머지 모든 detail이 preserve 되고, 가 크면 large sacel feature만 preserve 됨- 이는 conceptual compression으로도 볼 수 있음
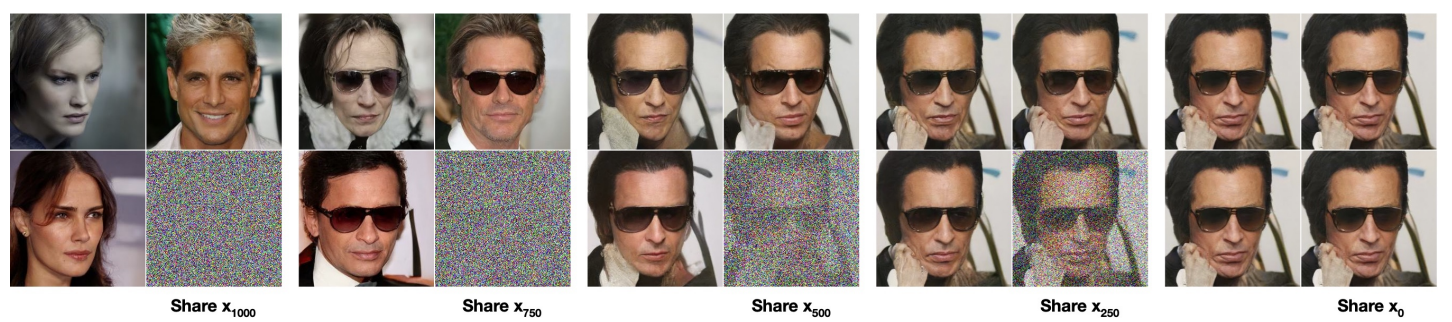
- Connection to Autoregressive Decoding
- (Eq. 5)의 variational bound는 다음과 같이 rewritten 할 수 있음:
(Eq. 16)
- 이 -th coordinate를 mask 하도록 하고, blank 이미지에 모든 mass를 place 하도록 를 설정하고, 를 취해 fully expressive conditional 분포로 표현할 수 있음 - 따라서 Gaussian diffusion model (Eq. 2)를 data coordinate의 reordering으로 표현할 수 없는, generalized bit ordering을 가진 autoregressive model로써 interpret 할 수 있음
- 이러한 reordering은 sample 품질에 영향을 주는 inductive bias를 제공하므로, DDPM의 Gaussian diffusion도 마찬가지로 유사한 목적을 가짐
- (Eq. 5)의 variational bound는 다음과 같이 rewritten 할 수 있음:
- Interpolation
를 stochastic encoder로 사용하여 latent space에서 source image 를 interpolate 할 수 있음- 결과적으로 reverse process를 통해 고품질의 reconstruction과 pose, skin tone, hairstyle, background 등을 부드럽게 변화시키는 plausible interpolation이 생성됨
- 다만 eyewear의 경우 이러한 interpolation이 나타나지 않음
- 추가적으로 가 클수록 더 coarse 하고 다양한 interpolation이 나타남

반응형
'Paper > ETC' 카테고리의 다른 글
댓글