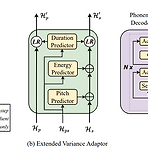
StyleSpeech: Self-Supervised Style Enhancing with VQ-VAE-based Pre-training for Expressive Audiobook Speech SynthesisAudiobook을 위한 음성 합성은 generalized architecture와 training data의 unbalanced style distribution으로 인해 한계가 있음StyleSpeechExpressive audiobook synthesis를 위해 VQ-VAE-based pre-training을 통한 self-supervised style enhancing method를 적용Text style encoder는 large-scale unlabeled text-only data로 p..
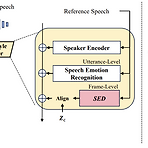
ED-TTS: Multi-Scale Emotion Modeling using Cross-Domain Emotion Diarization for Emotional Speech Synthesis기존의 emotional speech synthesis는 reference audio에서 추출된 utterance-level style embedding을 활용하기 때문에 speech prosody의 multi-scale property를 neglecting 하는 경우가 많음ED-TTSSpeech Emotion Diarization (SED)과 Speech Emotion Recognition (SER)을 활용하여 multi-scale에서 emotion을 모델링SER에서 추출한 utterance-level emotion..
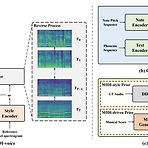
MIDI-Voice: Expressive Zero-Shot Singing Voice Synthesis via MIDI-Driven Priors기존의 Singing Voice Synthesis 모델은 unseen speaker와 fundamental frequency를 부정확하게 예측하므로 낮은 합성 품질을 보임MIDI-Voice더 나은 singing voice style adaptation을 위해 MIDI-based prior를 score-based diffusion model에 적용특히 MIDI-driven prior를 생성하여 note information을 반영하고 고품질의 style adaptation을 지원추가적으로 expressive synthesis를 위해 DDSP-based MIDI-sty..
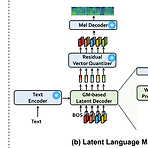
CLaM-TTS: Improving Neural Codec Language Modeling for Zero-Shot Text-to-SpeechZero-shot Text-to-Speech를 위해 audio의 discrete token에 대한 multiple stream을 encode 하는 neural audio codec을 활용할 수 있음이때 audio tokenization은 long sequence legnth와 multiple sequence modeling의 complexity로 인해 scalability의 한계가 있음CLaM-TTSToken length에 대한 뛰어난 compression을 달성하고, Language model이 한 번에 multiple token을 생성할 수 있도록 하는 prob..
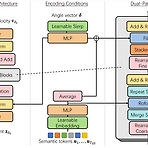
Efficient Neural Music GenerationMusicLM은 semantic, coarse acoustic, fine acoustic modeling을 통해 뛰어난 음악 생성 능력을 보여주고 있음BUT, MuiscLM은 fine-grained acoustic token을 얻기 위해 많은 계산 비용이 필요함MeLoDy고품질의 음악 생성이 가능하면서 forward pass의 효율성을 개선한 LM-guided diffusion modelSemantic modeling을 위해 MusicLM을 inherit 하고 dual-path diffusion과 audio VAE-GAN을 사용하여 conditioning semantic token을 waveform으로 decoding특히 dual-path dif..
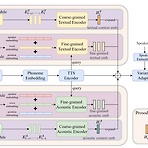
M2-CTTS: End-to-End Multi-Scale Multi-Modal Conversational Text-to-Speech SynthesisConversational text-to-speech는 historical conversation을 기반으로 적절한 prosody를 가진 음성을 합성하는 것을 목표로 함BUT, 기존 방식들은 대부분 global information 추출에만 초점을 맞추고 있으므로 keyword나 emphasis 같은 fine-grained information이 포함된 local prosody feature가 생략됨M2-CTTSHistorical conversation을 종합적으로 활용하고 prosodic expression을 향상하는 end-to-end multi-scal..
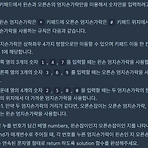
* Python을 기준으로 합니다구현 - 시뮬레이션 (Simulation)- 개념시뮬레이션 : 문제에서 주어진 과정을 하나씩 차례대로 직접 수행해 나가는 유형 시뮬레이션 문제 접근하기- 주어진 문제를 최대한으로 분리하기- 예외 처리는 독립적인 함수로 구분하기특히 시뮬레이션 문제는 2차원 배열을 활용하는 경우가 많으므로 행렬 연산을 응용해서 사용할 수도 있음- 문제가 길기 때문에 잘 분석해야함- 구현1. 행렬 연산행렬 덧셈, 뺄셈, 곱셈#행렬 덧셈def matrix_sum(a,b): result = [] for arow ,brow in zip(a, b): row = [] for aele, bele in zip(arow, brow): row.append..
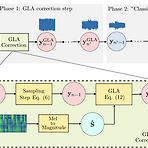
GLA-Grad: A Griffin-Lim Extended Waveform Generation Diffusion ModelDiffusion model은 diffusion process로 인한 비효율성이 존재하고 unseen speaker에 대한 고품질 합성이 어려움GLA-GradConditioning error를 최소화하면서 diffusion process의 효율성을 향상하기 위해 diffusion process의 각 step에 Griffin-Lim algorithm을 도입이를 통해 추가적인 training이나 fine-tuning 없이 already-trained waveform generation model에 직접 적용 가능논문 (ICASSP 2024) : Paper Link1. Introductio..
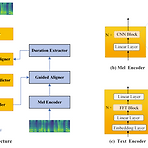
Eden-TTS: A Simple and Efficient Parallel Text-to-Speech Architecture with Collaborative Duration-Alignment LearningText-to-Speech에서 빠른 추론 속도는 non-autoregressive model를 통해 달성될 수 있고, 이때 parallel synthesis를 위해 text-speech alignment를 학습하는 것이 중요함- BUT, 기존 방식들은 복잡한 training procedure나 external aligner가 필요Eden-TTSSingle fully-differentiable model에서 duration prediction, text-speech alignment, speech ge..