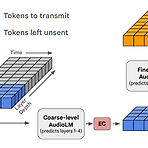
LMCodec: A Low Bitrate Speech Codec with Causal Transformer ModelsNeural codec은 낮은 bitrate로 고품질의 audio를 얻을 수 있어야 함LMCodecBackbone으로 residual vector quantization을 사용하여 audio를 coarse-to-fine token의 hierarchy로 encoding 하는 causal convolutional codec을 사용이때 generative 방식으로 coarse-to-fine token을 예측하도록 Transformer language model을 training 하여 더 적은 수의 code를 transmission 할 수 있음Second Transformer의 경우, past ..
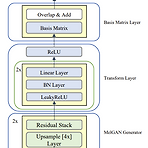
Basis-MelGAN: Efficient Neural Vocoder based on Audio DecompositionGenerative Adversarial Network (GAN) 기반의 vocoder는 autoregressive vocoder 보다는 빠른 합성이 가능하지만, 여전히 real-time으로 동작하는 것에는 한계가 있음- 특히 waveform length와 temporal resolution을 일치시키는 upsampling layer는 많은 시간을 소모함Basis-MelGANUpsampling layer의 계산량을 줄이기 위해 raw audio sample이 learned basis와 관련 weight로 decompose 함Prediction target을 raw audio sample..
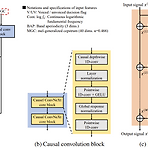
FIRNet: Fundamental Frequency Controllable Fast Neural Vocoder with Trainable Finite Impulse Response FilterFundamental frequency를 control 할 수 있는 neural vocoder는 우수한 합성 품질을 보이지만, 여전히 signal processing에 기반한 기존 vocoder들보다 느린 추론 속도를 가짐FIRNetTrainable time-variant Finite Impulse Response (FIR) filter를 갖춘 source-filter model을 활용Neural network를 통해 multiple FIR coefficient를 예측하고, mixed excitation signa..
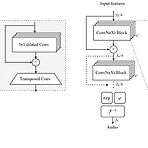
Vocos: Closing the Gap Between Time-domain and Fourier-based Neural Vocoders for High-Quality Audio Synthesis기존의 neural vocoder는 time-domain에서 동작하는 Generative Adversarial Network을 활용함BUT, 해당 방식은 time-frequency representation이 제공하는 inductive bias를 무시하므로 redundant, computationally-intense 한 upsampling operation이 요구됨Vocos더 빠른 계산과 human perception과의 align의 이점을 활용할 수 있는 Fourier-based time-frequency r..
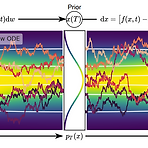
Score-based Generative Modeling through Stochastic Differential EquationsGenerative modeling은 noise로부터 data를 생성하는 것을 목표로 함Score-based Generative Modeling with SDENoise를 inject 하여 complex data distribution을 known prior distribution으로 변환하는 Stochastic Differential Equation (SDE)와 denoising을 통해 prior를 data distribution으로 변환하는 reverse-time SDE를 활용Score-based modeling을 활용하여 neural network를 통해 time-dep..
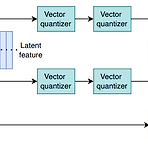
HiFi-Codec: Group-Residual Vector Quantization for High Fidelity Audio CodecAudio codec은 audio를 discrete representation으로 compress 하는 것으로써, 최근에는 생성 분야에서 intermediate representation으로 활용되고 있음BUT, audio codec은 large-scale dataset 부족과 reconstruction 성능 보장을 위한 codebook size의 부담으로 인한 어려움이 있음HiFi-Codec생성 모델의 부담을 완화하기 위해 Group-Residual Vector Quantization을 도입결과적으로 4개의 codebook 만으로도 high-fidelity의 audio..