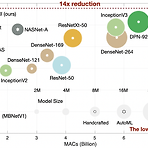
Once-For-All: Train One Network and Specialize it for Efficient Deployment 다양한 edge device에서 자원 제약을 만족하는 효율적인 inference의 어려움 Once-For-All (OFA) training / search를 분리하여 cost를 줄임 OFA에서 추가적인 training 없이 sub-network를 얻을 수 있음 Progressive Shrinking OFA network training을 위해 제안 -> depth/width/kernel/resolution pruning 논문 (ICRL 2020) : Paper Link OFA의 개선버전 : CompOFA 리뷰 1. Introduction model size의 증가는 har..
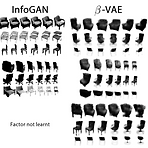
beta-VAE: Learning Basic Visual Concepts with a Constrained Variational Framework Supervision 없이 독립적인 data의 interpretable factorised representation을 학습하는 것이 중요 $\beta$-VAE (beta-VAE) Unsupervised 방식으로 raw image data에서 interpretable factorised latent representation을 발견 가능 adjustable parameter $\beta$ : latent channel capacity와 재구성 정확도의 independence 제약조건 조절 $\beta$-VAE는 train이 stable하고, data에 대한 가..