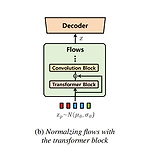
VITS2: Improving Quality and Efficiency of Single-Stage Text-to-Speech with Adversarial Learning and Architecture DesignSingle-stage text-to-speech model은 기존의 two-stage 방식보다 더 우수한 합성 품질을 보이고 있지만, phoneme conversion에 대한 dependency와 computational efficiency 측면에서 개선이 필요함VITS2기존의 VITS structure를 개선하여 보다 natural 한 음성 합성과 multi-speaker에서 더 나은 speaker similarity를 지원Fully end-to-end single-stage approac..
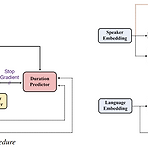
SANE-TTS: Stable and Natural End-to-End Multilingual Text-to-SpeechStable 하고 natural 한 end-to-end multilingual text-to-speech 모델이 필요함SANE-TTSMultilingual synthesis의 naturalness를 향상하기 위해 domain adversarial training을 도입추가적으로 speaker regularization loss를 적용하여 duration predictor의 speaker embedding을 zero-vector로 대체해 cross-lingual synthesis를 stablize 함논문 (INTERSPEECH 2021) : Paper Link1. IntroductionM..
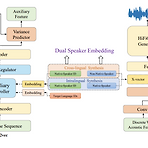
DSE-TTS: Dual Speaker Embedding for Cross-Lingual Text-to-SpeechCross-lingual text-to-speech는 speaker timbre를 정확하게 retain 하면서 nativeness를 반영하는 것이 어려움DSE-TTSMel-spectrogram 보다 더 적은 speaker information을 포함하는 vector-quantized acoustic feature를 활용해당 acoustic feature를 기반으로 speaking style을 반영하는 Dual Speaker Embedding을 도입- 한 embedding은 linguistic speaking stlye을 학습하기 위해 acoustic model에 전달되고,- 다른 embedd..
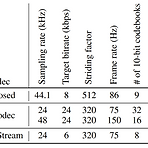
High-Fidelity Audio Compression with Improved RVQGANLanguage model의 핵심 component는 high-dimensional natural signal을 low-dimensional discrete token으로 compress 하는 neural codec임Improved RVQGANAdversarial, reconstruction loss와 vector quantization technique을 도입하여 high-fidelity의 audio compression을 보장추가적으로 speech, environment, music 등의 다양한 domain에 대한 universal compression을 지원논문 (NeruIPS 2023) : Paper Li..
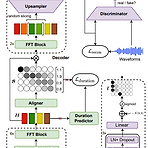
AutoTTS: End-to-End Text-to-Speech through Differentiable Duration ModelingText-to-Speech 모델은 일반적으로 external aligner가 필요하고, decoder와 jointly train 되지 않으므로 최적화의 한계가 있음AutoTTSInput, output sequence 간의 monotonic alignment를 학습하기 위해 differentiable duration method를 도입Expectation에서 stochastic process를 최적화하는 soft-duration mechanism을 기반으로 하여 direct text-to-waveform synthesis 모델을 구축추가적으로 adversarial train..
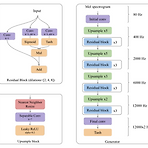
nVOC-22: A Low Cost Mel Spectrogram Vocoder for Mobile DevicesMobile CPU/GPU에서 동작할 수 있는 fully convolutional, non-autoregressive neural vocoder가 필요함nVOC-22Nearest neighbor resize와 separable convolution의 조합을 upsampling block에 적용하여 checkerboarding artifact를 최소화하고 빠른 upsampling을 지원추가적으로 Generative Adversarial Network를 기반으로 training 하여 안정적인 성능을 달성논문 (ICASSP 2023) : Paper Link1. Introduction음성 합성은 nav..