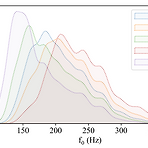
EdiTTS: Score-based Editing for Controllable Text-to-SpeechText-to-Speech를 위해 score-based modeling을 기반으로 speech editing method를 적용할 수 있음EdiTTSAdditional training이나 task-specific optimization 없이 content, pitch 측면에서 audio에 대한 targeted, granular editing을 허용Gaussian prior space에서 coarse, deliberate perturbation을 적용하여 diffusion model에서 desired behavior를 유도하고, mask와 softening kernel을 통해 target region에..
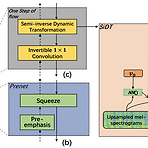
SiD-WaveFlow: A Low-Resource Vocoder Independent of Prior KnowledgeFlow-based nerual vocoder는 high-fidelity의 음성을 합성할 수 있지만, training에 많은 speech data가 필요하고 computationally heavy 함SiD-WaveFlowLow-resource 합성을 위한 flow-based neural vocoderWaveGlow의 Affine Coupling Layer의 계산 효율성을 개선하기 위해 Semi-inverse Dynamic Transformation module을 도입논문 (INTERSPEECH 2022) : Paper Link1. IntroductionVocoder는 mel-spectr..
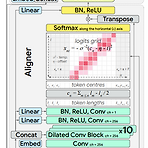
EATS: End-to-End Adversarial Text-to-SpeechText-to-Speech pipeline은 일반적으로 multiple stage 방식으로 구성됨EATSNormalized text나 phoneme에서 end-to-end 방식으로 음성을 합성하는 모델Feed-forward generator와 token length prediction에 기반한 differentiable alignment search를 통해 효과적인 training과 추론을 지원Adversarial feedback과 prediction loss를 조합하여 high-fidelity의 음성을 합성추가적으로 생성된 audio의 temporal variation을 capture 할 수 있는 dynamic time war..
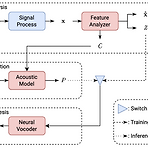
MSMC-TTS: Multi-Stage Multi-Codebook VQ-VAE based Neural TTSVector-quantized, compact speech representation을 도입하여 neural text-to-speech의 성능을 향상할 수 있음MSMC-TTSVector-Quantized Variational AutoEncoder based feature를 채택하여 acoustic feature를 서로 다른 time resolution의 sequence로 encoding 하고, 이를 multiple codebook으로 quantize 함Prediction 과정에서는 multi-stage predictor는 Euclidean distance와 triplet loss를 최소화하여 inp..
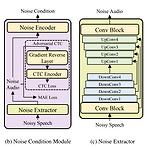
DenoiSpeech: Denoising Text to Speech with Frame-level Noise ModelingText-to-Speech 모델을 학습하기 위해서는 고품질의 speech data가 필요하지만, 대부분 noisy speech를 포함하고 있음DenoiSpeechNoisy speech data를 사용하여 clean speech를 합성할 수 있는 Text-to-Speech 모델모델과 jointly train 되는 noise condition module을 사용하여 fine-grained frame-level noise를 모델링하여 real-world noisy speech를 처리함논문 (ICASSP 2021) : Paper Link1. IntroductionText-to-Speech ..
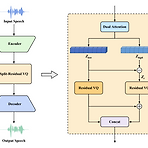
SRCodec: Split-Residual Vector Quantization for Neural Speech CodecEnd-to-End neural speech coding은 residual vector quantization을 통해 수행될 수 있지만, 가능한 적은 bit로 latent variable을 quantize 하는 것은 어려움SRCodecLatent representation을 동일한 dimension을 가지는 두 part로 split 하는 split-residual vector quantization을 채택한 fully convolutional encoder-decoder network- Low-dimensional feature와 high-dimensional feature 간의 res..