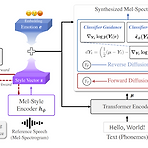
ZET-Speech: Zero-Shot Adaptive Emotion-Controllable Text-to-Speech Synthesis with Diffusion and Style-based Models Emotional Text-to-Speech는 natural 하고 emotional한 음성을 합성할 수 있음 BUT, 기존 방식들은 unseen speaker에 대한 generalization 없이 seen speaker만을 대상으로 함 ZET-Speech 짧은 speech segment와 target emotion label을 사용하여 any-speaker zero-shot adaptive text-to-speech 수행 Zero-shot adaptive model이 emotional speech를 ..
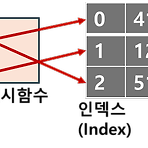
* Python을 기준으로 합니다 해시 테이블 (Hash Table) - 개념 해시 테이블 : 키와 값을 매핑해 데이터 양에 상관없이 빠른 탐색을 가능하는 자료구조 Python에서는 일반적으로 dictionary 자료형으로 해시 테이블을 활용함 해시 테이블의 탐색은 $O(1)$의 time complexity를 가진다는 장점이 있음 - 키 자체가 해시 함수에 의해 값이 있는 index가 되기 때문 따라서 특정 값을 기준으로 조회를 여러번 해야 하거나, 특정 값과 매핑되는 값의 관계를 활용하는 경우 사용 - BUT, 완전 탐색이나 정렬, 최대/최소 등이 필요한 경우는 배열이 더 유용함 Time Complexity $O(1)$ : 조회, 값 할당, 키 가져오기 `new_dict.keys()`, 딕셔너리 초기화..
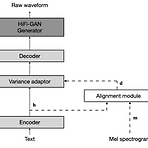
JETS: Jointly Training FastSpeech2 and HiFi-GAN for End to End Text to SpeechText-to-Speech는 2-stage 방식이나 개별적으로 training 된 모델의 cascade로 학습됨BUT, training pipeline은 최적의 성능을 위해서 fine-tuning이나 speech-text alignment를 요구함JETSSimplified pipeline을 구성해 개별적으로 학습된 모델들보다 뛰어난 성능을 발휘하는 end-to-end 모델을 제시Alignment module을 사용하여 FastSpeech2와 HiFi-GAN을 jointly trainingAlignment learning objective를 채택하여 external al..
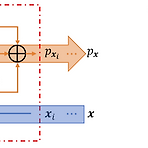
AutoNF: Automated Architecture Optimization of Normalizing Flows with Unconstrained Continuous Relaxtion Admitting Optimal Discrete Solution 강력하면서도 계산 효율적인 flow model을 구축하는 것은 여전히 어려움 이를 위해 Neural Architecture Search를 고려할 수 있지만, Normalizing Flow의 invertibility constraint로 인해 기존 방식들은 적용하기 어려움 AutoNF Normalizing Flow에 대한 automated architectural optimization framework Flow model의 invertibility cons..
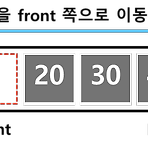
* Python을 기준으로 합니다 큐 (Queue), 덱 (Deque) - 개념 큐 : FIFO (First In First Out) 형태의 자료구조 처리해야 하는 작업들을 큐에 쌓음으로써 쉽게 처리할 수 있음 스택과 마찬가지로 삽입과 삭제를 핵심적인 연산으로 가짐 - 배열에서 `append()`나 `pop(0)`를 통해 삽입/삭제를 수행할 수 있지만, `pop(0)`는 $O(n)$을 소모함 - 따라서 큐는 연결 리스트를 통해 구현하는 것이 좋음 큐의 주요 연산 `enqueue()` : 큐의 맨 마지막에 새로운 요소를 추가 `dequeue()` : 큐의 첫 번째 요소를 pop() `isEmpty()` : 큐가 비어있으면 `True` 아니면 `False` 원형 큐 (Circular Queue) 선형 큐(L..
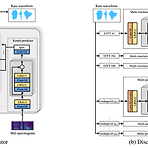
UnivNet: A Neural Vocoder with Multi-Resolution Spectrogram Discriminators for High-Fidelity Waveform Generation Full-band spectral feature를 사용하면 vocoder에 많은 acoustic information을 제공할 수 있음 - BUT, full-band mel-spectrogram 사용 시 over-smoothing 문제가 발생할 수 있음 UnivNet Full-band over-smoothing 문제를 해결하는 고품질 neural vocoder Multiple linear spectrogram magnitude를 사용하는 multi-resolution spectrogram discrimin..