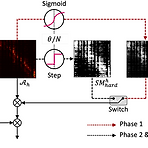
Pruning Self-Attention for Zero-Shot Multi-Speaker Text-to-Speech Personalized speech generation을 위해서는 target speaker의 limited data를 사용해서 Text-to-Speech를 수행해야 함 이를 위해 text-to-speech 모델은 out-of-domain data에 대해 amply generalize 되어야 함 Self-Attention Pruning Generalization을 위해 text-to-speech 모델의 transformer에 대해 spase attention을 통한 pruning을 적용 Attention weight가 threshold 보다 낮은 self-attention layer에서 ..
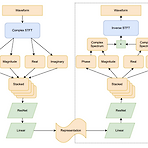
AutoVocoder: Fast Waveform Generation from a Learned Speech Representation Using Differentiable Digital Signal Processing Mel-spectrogram은 waveform으로부터 간단하게 추출될 수 있지만, mel-spectrogram에서 waveform을 생성하는 vocoder에는 많은 계산 비용이 필요함 AutoVocoder 기존 mel-spectrogram 방식에서 벗어나 inverse STFT의 differentiable implementation을 사용하여 waveform을 생성 결과적으로 기존 neural vocoder에 비해 14배 이상의 가속 효과를 달성 논문 (ICASSP 2023) : Paper..
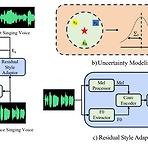
StyleSinger: Style Transfer for Out-of-Domain Singing Voice SynthesisSinging Voice Synthesis의 경우 높은 expressiveness를 요구하기 때문에 voice style을 모델링하는 것이 까다로움특히 기존의 모델들은 training 단계에서 target vocal attribute를 discernible 한다는 가정에 기반하기 때문에 out-of-domain 환경으로 확장이 어려움StyleSingerResidual quantization module을 통해 다양한 style을 capture 하는 Residual Style Adaptor의 적용Style attribute를 perturb 하여 generalization을 향상하는 U..
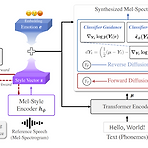
ZET-Speech: Zero-Shot Adaptive Emotion-Controllable Text-to-Speech Synthesis with Diffusion and Style-based Models Emotional Text-to-Speech는 natural 하고 emotional한 음성을 합성할 수 있음 BUT, 기존 방식들은 unseen speaker에 대한 generalization 없이 seen speaker만을 대상으로 함 ZET-Speech 짧은 speech segment와 target emotion label을 사용하여 any-speaker zero-shot adaptive text-to-speech 수행 Zero-shot adaptive model이 emotional speech를 ..
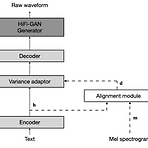
JETS: Jointly Training FastSpeech2 and HiFi-GAN for End to End Text to SpeechText-to-Speech는 2-stage 방식이나 개별적으로 training 된 모델의 cascade로 학습됨BUT, training pipeline은 최적의 성능을 위해서 fine-tuning이나 speech-text alignment를 요구함JETSSimplified pipeline을 구성해 개별적으로 학습된 모델들보다 뛰어난 성능을 발휘하는 end-to-end 모델을 제시Alignment module을 사용하여 FastSpeech2와 HiFi-GAN을 jointly trainingAlignment learning objective를 채택하여 external al..
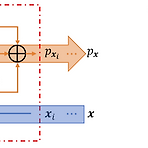
AutoNF: Automated Architecture Optimization of Normalizing Flows with Unconstrained Continuous Relaxtion Admitting Optimal Discrete Solution강력하면서도 계산 효율적인 flow model을 구축하는 것은 여전히 어려움이를 위해 Neural Architecture Search를 고려할 수 있지만, Normalizing Flow의 invertibility constraint로 인해 기존 방식들은 적용하기 어려움AutoNFNormalizing Flow에 대한 automated architectural optimization frameworkFlow model의 invertibility constrain..