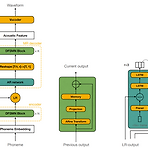
DeviceTTS: A Small-Footprint, Fast, Stable Network for On-device Text-to-Speech기존의 tex-to-speech 모델은 크고 복잡한 network로 구성되기 때문에, 원활한 배포를 지원할 수 있는 on-device text-to-speech에 적합한 모델이 필요함DeviceTTSDuration predictor를 통해 encoder, decoder 간의 bridge를 제공모델 size를 줄이기 위해 Deep Feedforward Sequential Memory Network (DFSMN)을 도입추가적으로 추론 속도를 높이기 위해, mix-resolution decoder를 채택논문 (ICASSP 2021) : Paper Link1. Introd..
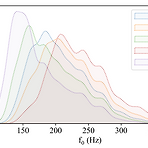
EdiTTS: Score-based Editing for Controllable Text-to-SpeechText-to-Speech를 위해 score-based modeling을 기반으로 speech editing method를 적용할 수 있음EdiTTSAdditional training이나 task-specific optimization 없이 content, pitch 측면에서 audio에 대한 targeted, granular editing을 허용Gaussian prior space에서 coarse, deliberate perturbation을 적용하여 diffusion model에서 desired behavior를 유도하고, mask와 softening kernel을 통해 target region에..
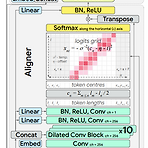
EATS: End-to-End Adversarial Text-to-SpeechText-to-Speech pipeline은 일반적으로 multiple stage 방식으로 구성됨EATSNormalized text나 phoneme에서 end-to-end 방식으로 음성을 합성하는 모델Feed-forward generator와 token length prediction에 기반한 differentiable alignment search를 통해 효과적인 training과 추론을 지원Adversarial feedback과 prediction loss를 조합하여 high-fidelity의 음성을 합성추가적으로 생성된 audio의 temporal variation을 capture 할 수 있는 dynamic time war..
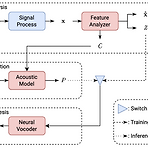
MSMC-TTS: Multi-Stage Multi-Codebook VQ-VAE based Neural TTSVector-quantized, compact speech representation을 도입하여 neural text-to-speech의 성능을 향상할 수 있음MSMC-TTSVector-Quantized Variational AutoEncoder based feature를 채택하여 acoustic feature를 서로 다른 time resolution의 sequence로 encoding 하고, 이를 multiple codebook으로 quantize 함Prediction 과정에서는 multi-stage predictor는 Euclidean distance와 triplet loss를 최소화하여 inp..
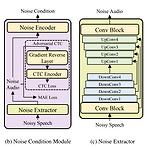
DenoiSpeech: Denoising Text to Speech with Frame-level Noise ModelingText-to-Speech 모델을 학습하기 위해서는 고품질의 speech data가 필요하지만, 대부분 noisy speech를 포함하고 있음DenoiSpeechNoisy speech data를 사용하여 clean speech를 합성할 수 있는 Text-to-Speech 모델모델과 jointly train 되는 noise condition module을 사용하여 fine-grained frame-level noise를 모델링하여 real-world noisy speech를 처리함논문 (ICASSP 2021) : Paper Link1. IntroductionText-to-Speech ..
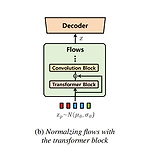
VITS2: Improving Quality and Efficiency of Single-Stage Text-to-Speech with Adversarial Learning and Architecture DesignSingle-stage text-to-speech model은 기존의 two-stage 방식보다 더 우수한 합성 품질을 보이고 있지만, phoneme conversion에 대한 dependency와 computational efficiency 측면에서 개선이 필요함VITS2기존의 VITS structure를 개선하여 보다 natural 한 음성 합성과 multi-speaker에서 더 나은 speaker similarity를 지원Fully end-to-end single-stage approac..