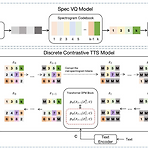
DCTTS: Discrete Diffusion Model with Contrastive Learning for Text-to-Speech Generation Text-to-Speech에서 latent diffusion model을 우수한 성능을 보이고 있지만, resource consumption이 크고 추론 속도가 느림 DCTTS Discrete diffusion model과 contrastive learning을 결합한 text-to-speech 모델 간단한 text encoder와 VQ model을 사용하여 raw data를 discrete space로 compress 한 다음, discrete space에서 diffusion model을 training 함 이때 diffusion step 수를 줄..
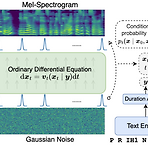
VoiceFlow: Efficient Text-to-Speech with Rectified Flow Matching Text-to-Speech에서 diffusion model은 우수한 성능을 보이고 있지만 sampling complexity로 인해 비효율적임 VoiceFlow 제한된 sampling step으로도 고품질의 합성을 수행할 수 있는 rectified flow matching을 활용 Text input을 condition으로 하여 mel-spectrogram을 ordinary differential equation을 통해 추정 Rectified flow는 효율적인 합성을 위해 sampling trajectory를 straighten 함 논문 (ICASSP 2024) : Paper Link 1...
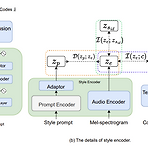
InstructTTS: Modelling Expressive TTS in Discrete Latent Space with Natural Language Style Prompt Expressive Text-to-Speech는 다양한 speech pattern을 반영하는 것을 목표로 하고, 이때 style을 control 하는 style prompt로 natural language를 활용할 수 있음 InstructTTS Self-supervised learning과 cross-modal metric learning을 활용하고 robust sentence embedding model을 얻기 위해 3-stage training을 제시 일반적인 mel-spectrogram 대신 vector-quantized ac..
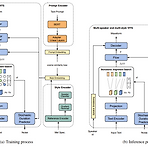
PromptStyle: Controllable Style Transfer for Text-to-Speech with Natural Language Descriptions Text-to-Speech에서 style control을 위해서는 개별적인 style category가 있는 expressive speech recording이 필요함 BUT, 실적용에서는 target style에 대한 referecne speech 없이 desired style에 대한 text description을 활용하는 것이 더 적합하다고 볼 수 있음 PromptStyle Text prompt-guided cross-speaker style transfer를 목표로 VITS와 cross-modal style encoder를 활용 ..
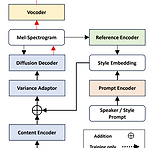
PromptTTS++: Controlling Speaker Identity in Prompt-based Text-to-Speech using Natural Language Descriptions Natural language description을 사용하여 speaker identity를 control 하는 prompt-based text-to-speech를 수행할 수 있음 PromptTTS++ Speaker identity를 control 하기 위해, speaking style과 independent 하도록 설계된 voice characteristic을 설명하는 speaker prompt를 도입 Diffusion-based acoustic model을 사용하여 다양한 speaker factor를 모델링..
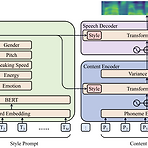
PromptTTS: Controllable Text-to-Speech with Text Descriptions Text description을 generation task를 guide 하는 데 사용할 수 있음 PromptTTS 음성 합성을 위해 style, content description이 포함된 prompt를 input으로 사용하는 text-to-speech 모델 Prompt에서 해당 representation을 추출하는 style encoder, content encoder를 활용하고, 추출된 style, content representation에 따라 음성을 합성하는 speech decoder로 구성됨 추가적으로 prompt가 포함된 dataset이 없으므로, 이에 해당하는 새로운 dataset..