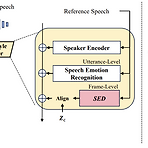
ED-TTS: Multi-Scale Emotion Modeling using Cross-Domain Emotion Diarization for Emotional Speech Synthesis기존의 emotional speech synthesis는 reference audio에서 추출된 utterance-level style embedding을 활용하기 때문에 speech prosody의 multi-scale property를 neglecting 하는 경우가 많음ED-TTSSpeech Emotion Diarization (SED)과 Speech Emotion Recognition (SER)을 활용하여 multi-scale에서 emotion을 모델링SER에서 추출한 utterance-level emotion..
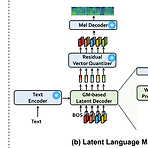
CLaM-TTS: Improving Neural Codec Language Modeling for Zero-Shot Text-to-SpeechZero-shot Text-to-Speech를 위해 audio의 discrete token에 대한 multiple stream을 encode 하는 neural audio codec을 활용할 수 있음이때 audio tokenization은 long sequence legnth와 multiple sequence modeling의 complexity로 인해 scalability의 한계가 있음CLaM-TTSToken length에 대한 뛰어난 compression을 달성하고, Language model이 한 번에 multiple token을 생성할 수 있도록 하는 prob..
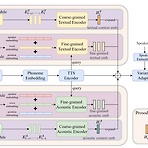
M2-CTTS: End-to-End Multi-Scale Multi-Modal Conversational Text-to-Speech SynthesisConversational text-to-speech는 historical conversation을 기반으로 적절한 prosody를 가진 음성을 합성하는 것을 목표로 함BUT, 기존 방식들은 대부분 global information 추출에만 초점을 맞추고 있으므로 keyword나 emphasis 같은 fine-grained information이 포함된 local prosody feature가 생략됨M2-CTTSHistorical conversation을 종합적으로 활용하고 prosodic expression을 향상하는 end-to-end multi-scal..
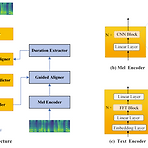
Eden-TTS: A Simple and Efficient Parallel Text-to-Speech Architecture with Collaborative Duration-Alignment LearningText-to-Speech에서 빠른 추론 속도는 non-autoregressive model를 통해 달성될 수 있고, 이때 parallel synthesis를 위해 text-speech alignment를 학습하는 것이 중요함- BUT, 기존 방식들은 복잡한 training procedure나 external aligner가 필요Eden-TTSSingle fully-differentiable model에서 duration prediction, text-speech alignment, speech ge..
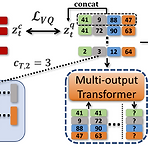
MQTTS: A Vector Quantized Approach for Text to Speech Synthesis on Real-World Spontaneous SpeechText-to-Speech에서 human-level diversity를 반영할 필요가 있음MQTTSMel-spectrogram based autoregressive model의 alignment mismatch 문제를 해결하기 위해 multiple code group으로 학습된 discrete code를 활용합성 품질 향상을 위해 clean silence prompt를 활용하고 multiple code generation과 monotonic alignment architecture를 도입논문 (AAAI 2023) : Paper Link..
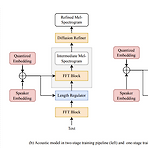
IST-TTS: Interpretable Style Transfer for Text-to-Speech with ControlVAE and Diffusion BridgeText-to-Speech에서 style transfer는 중요해지고 있음IST-TTSVariational autoencoder (VAE)와 diffusion refiner를 결합하여 refined mel-spectrogram을 얻음- 이때 audio 품질과 style transfer 성능을 향상하기 위해 two-stage, one-stage system을 각각 설계함Quantized VAE의 diffusion bridge를 통해 complex discrete style representation을 학습하고 transfer 성능을 향상더 나..