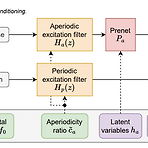
Embedding a Differentiable Mel-Cepstral Synthesis Filter to a Neural Speech Synthesis System End-to-End controllable speech synthesis를 위해 Mel-cepstral synthesis filter를 활용할 수 있음 Differentiable Mel-Cepstral Synthesis Filter Mel-cepstral synthesis filter를 통해 voice characteristics와 pitch는 각각 frequency warping parameter와 fundamental frequency를 통해 control 될 수 있음 이때 End-to-End 방식으로 최적화할 수 있도록 diffeten..
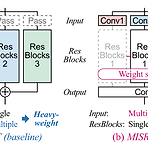
MISRNet: Lightweight Neural Vocoder Using Multi-Input Single Shared Residual Blocks HiFi-GAN의 Multi-Receptive field Fusion (MRF)은 branch 수에 따라 모델 size가 증가하는 단점이 있음 MISRNet MRF의 대안으로 Multi-Input Single Shared Residual block을 도입하여 residual block을 mutiple에서 single 단위로 변형 Residual block의 input convolution size를 줄임으로써 전체적인 모델을 경량화하고, tensor reshaping을 도입하여 처리 속도를 향상 논문 (INTERSPEECH 2022) : Paper Link..
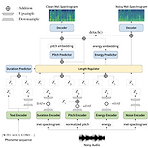
STYLER: Style Factor Modeling with Rapidity and Robustness via Speech Decomposition for Expressive and Controllable Neural Text to Speech Text-to-Speech는 어려운 합성 condition에 대한 robustness와 expressiveness, controllability를 요구함 STYLER Mel-Calibrator를 통한 audio-text aligning을 도입하여 unseen data에 대한 robust 한 추론을 가능하게 함 Supervision 하에서 disentangled style factor modeling을 통해 controllability를 향상 Domain adve..
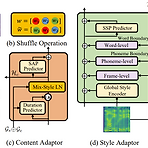
GenerSpeech: Towards Style Transfer for Generalizble Out-of-Domain Text-to-Speech Out-of-Domain 음성 합성을 위해 style transfer를 활용할 수 있지만 몇 가지 한계가 존재함 - Expressive voice의 dynamic style feature는 모델링과 transfer가 어려움 - Text-to-Speech 모델은 source data와 다른 Out-of-Domain condition을 handle 할 수 있을 만큼 robust 해야 함 GenerSpeech Out-of-Domain custom voice에 대해 high-fidelity zero-shot style transfer를 가능하게 하는 text-to-s..
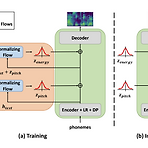
VarianceFlow: High-Quality and Controllable Text-to-Speech using Variance Information via Normalizing Flow Text와 speech 간의 one-to-many 관계를 학습하기 위해 두 가지 방식을 활용할 수 있음 - Normalizing Flow의 사용 - 합성 과정에서 pitch, energy 같은 variance information의 반영 VarianceFlow Normalizing Flow를 통해 variance를 모델링하여 더 정확하게 variance information을 예측 Normalizing Flow의 objective function은 variance와 text를 disentangle 하여 varianc..
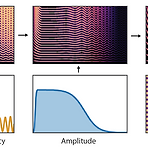
DDSP: Differentiable Digital Signal Processing 대부분의 audio 생성 모델은 time 또는 frequency domain 중 하나에서 sampling을 생성함 - Signal을 표현하는 데는 적합하지만 sound가 생성되고 인식되는 방식에 대한 knowledge를 활용하지 않음 Vocoder의 경우 domain knowledge를 성공적으로 반영할 수 있지만 auto-differentiable-based 방식과는 통합하기 어려움 Differentiable Digital Signal Processing (DDSP) 기존의 signal processing 요소를 deep learning 방식과 통합 Neural network의 expressive power를 잃지 않으..