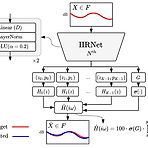
Direct Design of Biquad Filter Cascades with Deep Learning by Sampling Random Polynomials Arbitrary magnitude response와 match 하도록 Infinite Impulse Response filter를 설계하는 것은 어려움 - Yule-Walker method는 효율적이지만 high-order response를 정확하게 match 하지 못함 - Iterative optimization은 우수한 성능을 보이지만 initial condition에 민감 IIRNet 수백만개의 random filter에 대해 학습된 neural network를 사용하여 target magnitude response에서 filter coe..
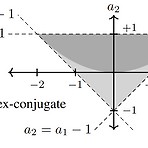
Lightweight and Interpretable Neural Modeling of an Audio Distortion Effect Using Hyperconditioned Differentiable Biquads Audio distortion effect를 모델링하기 위해 differentiable cacaded biquads를 사용할 수 있음 Hyperconditioned Differentiable Biquads Trainable Infinite Impulse Response (IIR) filter를 hyperconditioned case로 확장 Transformation은 distortion effect의 external parameter를 internal filter와 gain paramete..
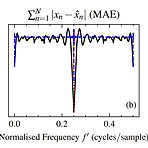
Sinusoidal Frequency Estimation by Gradient Descent Gradient descent를 사용하여 sinusoidal frequency parameter를 추정하는 것은 어려움 - Error function이 non-convex 하고 local minima에 densely populated 되어 있기 때문 Sinusoidal Frequency Estimation by Gradient Descent Complex exponential surrogate의 Wirtinger derivative와 first order gradient-based optimizer를 활용 Differentiable signal processing을 oscillatory component의 fre..
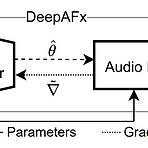
Differentiable Signal Processing with Black-Box Audio Effects Audio effect를 deep neural network로 통합하여 automate audio signal processing을 수행할 수 있음 DeepAFx Non-differentiable black-box effect layer를 학습시키기 위해 stochastic gradient approximation을 활용하여 end-to-end backpropagation을 생성 Tube amplifier emulation, automatic mastering, breath removal에 대한 audio production 작업에 적용 가능 논문 (ICASSP 2021) : Paper Link..
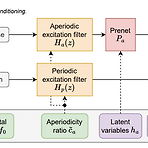
Embedding a Differentiable Mel-Cepstral Synthesis Filter to a Neural Speech Synthesis SystemEnd-to-End controllable speech synthesis를 위해 Mel-cepstral synthesis filter를 활용할 수 있음Differentiable Mel-Cepstral Synthesis FilterMel-cepstral synthesis filter를 통해 voice characteristics와 pitch는 각각 frequency warping parameter와 fundamental frequency를 통해 control 될 수 있음이때 End-to-End 방식으로 최적화할 수 있도록 diffetentiab..
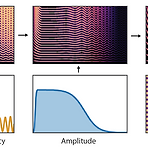
DDSP: Differentiable Digital Signal Processing대부분의 audio 생성 모델은 time 또는 frequency domain 중 하나에서 sampling을 생성함- Signal을 표현하는 데는 적합하지만 sound가 생성되고 인식되는 방식에 대한 knowledge를 활용하지 않음Vocoder의 경우 domain knowledge를 성공적으로 반영할 수 있지만 auto-differentiable-based 방식과는 통합하기 어려움Differentiable Digital Signal Processing (DDSP)기존의 signal processing 요소를 deep learning 방식과 통합Neural network의 expressive power를 잃지 않으면서 강력한..