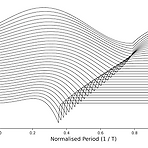
Singing Voice Synthesis Using Differentiable LPC and Glottal-Flow-Inspired Wavetables Singing Voice Synthesis를 위해 human voice의 physical characteristic을 활용할 수 있음 Glottal-Flow LPC Filter (GOLF) Harmonic source로써 glottal model을 사용하고, vocal tract를 simulate 하기 위해 IIR filter를 활용 GOLF는 더 적은 parameter와 memory를 사용함으로써 빠른 추론이 가능함 GOLF는 singing voice를 다양화할 수 있는 phase component를 modelling할 수 있음 논문 (ISMIR 20..
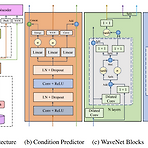
LiteSing: Towards Fast, Lightweight and Expressive Singing Voice Synthesis 경량화된 고품질의 Singing Voice Synthesis 시스템이 필요함 LiteSing Generative Adversarial Network architecture 하에서 악보의 full condition을 예측하고, 해당 condition에서 acoustic feature를 생성 Dynamic spectrogram energy, Voiced/Unvoiced decision, Dynamic pitch curve를 구성해 expressiveness를 향상 Pitch와 timbre를 개별적으로 예측하여 두 feature의 interdependence를 회피 논문 (IC..
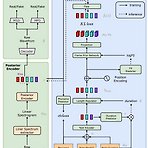
VISinger: Variational Inference with Adversarial Learning for End-to-End Singing Voice Synthesis 가사와 악보로부터 가창 음성을 직접 생성하는 End-to-End Singing Voice Synthesis (SVS) 모델 Normalizing flow 기반 VAE를 채택한 End-to-End Text-to-Speech (TTS) 모델인 VITS를 활용 VISinger Phoneme-level 평균, 분산 대신 Length regulator, Frame prior network를 사용하여 노래의 음향 변화를 모델링 F0 predictor를 통한 안정적인 가창 음성 합성 리듬감 향상을 위한 Duration predictor의 수정 논..
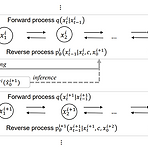
Hierarchical Diffusion Models for Singing Voice Neural Vocoder Pitch, loudness, pronunciation 같은 다양한 음악적 표현으로 인해 neural vocoder로 고품질의 가창 음성을 합성하는 것은 어려움 서로 다른 sampling rate에 대한 multiple diffusion model을 도입 HPG (Hierarchical Diffusion Model + PriorGrad) Lower sampling rate 모델은 pitch와 같은 저주파 요소를 합성 다른 모델은 lower sampling rate와 acoustic feature를 기반으로 higher sampling rate waveform을 점진적으로 합성 논문 (ICASS..
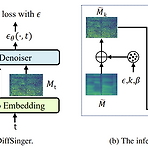
DiffSinger: Singing Voice Synthesis via Shallow Diffusion Mechanism Singing Voice Synthesis (SVS)는 음향 feature 재구성을 위해 간단한 Loss나 GAN을 활용함 각각의 방식은 over-smoothing 문제와 불안정한 학습과정으로 인해 부자연스러운 음성을 만들어냄 DiffSinger Diffusion probabilistic 모델 기반의 SVS용 음향 모델 조건부 분포 하에서 노이즈를 mel-spectrogram으로 반복적으로 변환하는 parameterized Markov chain Variational bound를 최적화함으로써 안정적이고 자연스러운 음성을 합성 논문 (AAAI 2022) : Paper Link 1. I..