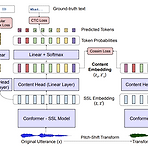
ACE-VC: Adaptive and Controllable Voice Conversion using Explicitly Disentangled Self-Supervised Speech RepresentationsVoice conversion을 위해 self-supervised learning으로 얻어진 speech representation을 활용할 수 있음ACE-VCContent/speaker representation을 disentangle 하기 위해 original과 pitch-shifted audio content representation 간의 similarity에 기반한 siamese network를 활용Decomposed representation으로부터 speech signal을 reco..
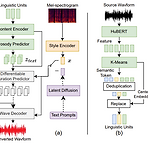
PromptVC: Flexible Stylistic Voice Conversion in Latent Space Driven by Natural Language Prompts기존의 voice conversion은 pre-defined label이나 reference speech에 의존적이므로 style의 한계가 있음PromptVCLatent diffusion model을 활용하여 natural language prompt에 의해 driven 된 style vector를 생성Style expressiveness를 향상하기 위해 HuBERT를 활용하여 discrete token을 추출하고, $k$-means center embedding을 적용하여 residual style information을 최소화추가적..
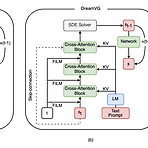
DreamVoice: Text-Guided Voice ConversionText-guided generation을 활용하면 user need에 따른 음성을 합성할 수 있음DreamVoiceEnd-to-End diffusion-based text-guided voice conversion을 위한 DreamVC와 text-to-voice generation을 위한 DreamVG를 제공추가적으로 VCTK, LibriTTS에 대한 voice timbre annotation을 가진 DreamVoiceDB dataset을 구축논문 (INTERSPEECH 2024) : Paper Link1. IntroductionVoice Conversion (VC)는 training/inference 중에 target voice의..
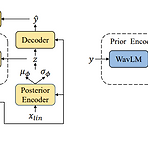
FreeVC: Towards High-Quality Text-Free One-Shot Voice Conversion기존의 voice conversion은 speaker information이 leak 되거나 많은 양의 annotated data가 필요함FreeVCVITS의 end-to-end framework를 채택하고 text annotation 없이 clean content information을 추출- 특히 WavLM feature에 information bottleneck을 impose 하여 content information을 disentangling추출된 content information의 purity를 향상하기 위해 spectrogram-resize based data augmentatio..
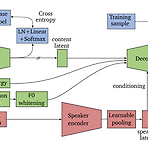
StreamVC: Real-Time Low-Latency Voice ConversionLightweight, high-quality conversion을 지원하는 streaming voice conversion 모델이 필요함StreamVCSoundStream의 neural audio codec architecture를 활용Soft speech unit을 causal 하게 학습하고 pitch stability를 향상하기 위해 whitened fundamental frequency information을 제공논문 (ICASSP 2024) : Paper Link1. IntroductionVoice Conversion (VC)는 linguistic content를 preserve 하면서 speech signal..
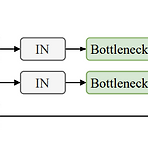
S2VC: A Framework for Any-to-Any Voice Conversion with Self-Supervised Pretrained RepresentationsAny-to-Any Voice Conversion은 seen/unseen speaker의 모든 utterance로 변환을 수행할 수 있어야 함S2VCSource/target feature로 self-supervised feature를 사용Speaker-independent 하고 content information을 추출할 수 있는 supervised phoneme posteriorgram을 baseline feature로 선정논문 (INTERSPEECH 2021) : Paper Link1. IntroductionSelf-Supervi..
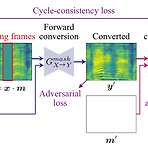
MaskCycleGAN-VC: Learning Non-Parallel Voice Conversion with Filling in FramesNon-parallel voice conversion을 위한 Cycle-Consistent Adversarial Network-based 방식은 time-frequency structure를 capture 하는 능력이 부족함MaskCycleGAN-VCCycleGAN-VC2의 확장으로써 Filling in Frames를 통해 training 하여 얻어짐Filling in Frames를 사용하여 input mel-spectrogram에 temporal mask를 적용하고 converter가 surrounding frame을 기반으로 missing frame을 fillin..
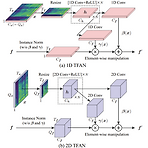
CycleGAN-VC3: Examining and Improving CycleGAN-VCs for Mel-Spectrogram ConversionNon-parallel voice conversion에서 CycleGAN-VC가 우수한 성능을 보임- BUT, mel-spectrogram conversion에 대한 ambiguity로 인해 time-frequency structure가 손상됨CycleGAN-VC3Time-Frequency Adaptive Normalization을 도입하여 time-frequency structure를 반영기존 CycleGAN의 mel-spectrogram conversion 성능을 향상논문 (INTERSPEECH 2020) : Paper Link1. IntroductionVo..
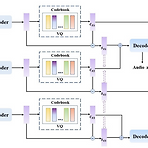
AVQVC: One-Shot Voice Conversion by Vector Quantization with Applying Contrastive LearningVoice conversion은 speech signal에서 timbre와 linguistic content를 disentangle 하여 수행될 수 있음AVQVCVQVC와 AutoVC를 결합한 one-shot voice conversion frameworkContent, timbre를 분리하기 위한 training method를 VQVC에 적용논문 (ICASSP 2022) : Paper Link1. IntroductionVoice Conversion (VC)는 original utterance의 content를 유지하면서 target speake..