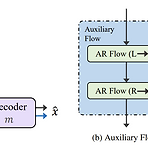
DFlow: A Generative Model Combining Denoising AutoEncoder and Normalizing Flow for High Fidelity Waveform GenerationHigh-fidelity의 waveform generation을 위한 vocoder가 필요함DFlow고품질 생성을 위해 Normalizing Flow와 Denoising AutoEncoder를 결합추가적으로 model size와 training set을 확장하여 DFlow를 large-scale universal vocoder로 scaling up논문 (ICML 2024) : Paper Link1. IntroductionDeep Generative Model (DGM)은 waveform generat..
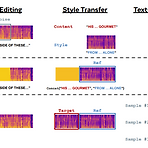
Voicebox: Text-Guided Multilingual Universal Speech Generation at ScaleLarge-scale generative model은 고품질의 output을 생성할 수 있지만, scale과 task generalization 측면에서 한계가 있음Voicebox주어진 audio context와 text를 기반으로 speech를 infill 하도록 train 된 non-autoregressive flow-matching modelIn-context learning을 통해 cross-lingual zero-shot synthesis, noise removal, content editing, style conversion 등의 다양한 task를 지원논문 (NeurI..
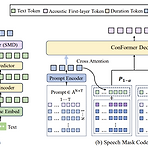
MobileSpeech: A Fast and High-Fidelity Framework for Mobile Zero-Shot Text-to-SpeechZero-shot Text-to-Speech는 few-second unseen speaker voice prompt로 강력한 voice cloning capability를 달성할 수 있음BUT, 대부분의 기존 방식들은 우수한 합성 품질에 비해 추론 속도, model size 측면의 한계가 있음MobileSpeechDiscrete codec를 기반으로 speech codec의 hierarchical information과 weight mechanism을 incorporate 하는 Speech Mask Decoder module을 도입- 특히 text와 spe..
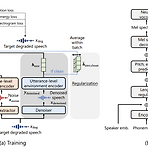
DRSpeech: Degradation-Robust Text-to-Speech Synthesis with Frame-Level and Utterance-Level Acoustic Representation Learning대부분의 text-to-speech system은 well-designed 환경에서 수집된 고품질 corpus를 활용하므로 데이터 수집 비용이 높음DRSpeechNoisy speech corpora를 training data로 활용할 수 있는 noise-robust text-to-speech 모델Frame-level encoder를 통해 time-variant additive noise를 represent 하고 utterance-level encoder를 사용하여 time-invarian..
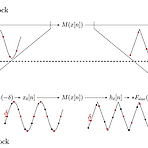
JenGAN: Stacked Shifted Filters in GAN-based Speech SynthesisNon-autoregressive GAN-based vocoder는 빠른 추론 속도와 우수한 품질을 지원하지만, audible artifact가 발생하는 경향이 있음JenGANShift-equivariant property를 보장하기 위해 shifted low-pass filter를 stack 하는 training strategy추론 시에 사용되는 model structure를 유지하면서 aliasing을 방지하고 artifact를 줄임논문 (INTERSPEECH 2024) : Paper Link1. IntroductionNeural vocoder는 mel-spectrogram과 같은 audio ..
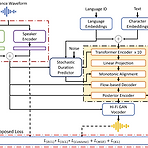
VECL-TTS: Voice Identity and Emotional Style Controllable Cross-Lingual Text-to-SpeechText-to-Speech는 여전히 voice identity와 emotional style 측면에서 합성의 한계가 있음VECL-TTSSource language의 reference speech에서 voice identity와 emotional style을 추출한 다음 cross-lingual technique을 사용해 target language로 transfer 함Multi-lingual speaker와 emotion embedding block을 도입하고 음성 품질을 향상하기 위해 content loss와 style consistency loss를..
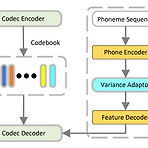
DelightfulTTS2: End-to-End Speech Synthesis with Adversarial Vector-Quantized Auto-Encoders일반적으로 text-to-speech는 mel-spectrogram을 intermediate representation으로 사용하는 cascaded pipeline을 활용함BUT, acoustic model과 vocoder는 개별적으로 training 되고, pre-designed mel-spectrogram은 sub-optimal 하다는 한계가 있음DelightfulTTS2Automatically learned speech representation과 joint optimization을 활용한 end-to-end text-to-speech 모..
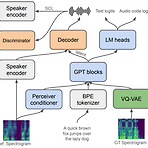
XTTS: A Massively Multilingual Zero-Shot Text-to-Speech Model대부분의 zero-shot multi-speaker text-to-speech 모델은 single language만 지원함XTTS16개의 다양한 low/medium resource language로 task를 확장Multilingual training을 지원하고 voice cloning을 개선하여 빠른 training/추론 속도를 달성논문 (INTERSPEECH 2024) : Paper Link1. IntroductionZero-shot multi-speaker Text-to-Speech (ZS-TTS)는 few-second speech를 기반으로 unseen speaker에 대한 음성 합성을 목..
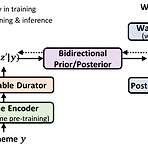
NaturalSpeech: End-to-End Text-to-Speech Synthesis with Human-Level QualityText-to-Speech에서 human-level quality를 판단하는 것은 어려움NaturalSpeechHuman-level quality를 달성하기 위해 variational auto-encoder를 활용한 end-to-end text-to-speech 모델Phoneme pre-training, differentiable duration modeling, bidirectional prior/posterior modeling, VAE memory mechanism을 포함논문 (PAMI 2024) : Paper Link1. IntroductionText-to-Spee..