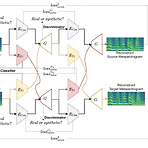
DRVC: A Framework of Any-to-Any Voice Conversion with Self-Supervised LearningAny-to-Any voice conversion은 training data에서 벗어난 source/target speaker에 대해 voice conversion을 수행하는 것을 목표로 함- BUT, 기존의 disentangle-based model은 speaker/content style information를 얻는 과정에서 untangle overlapping 문제가 발생함DRVC (Disentangled Representation Voice Conversion)Content encoder, timbre encoder, generator로 구성된 end-to-e..
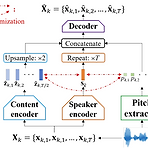
VQMIVC: Quantization and Mutual Information-based Unsupervised Speech Representation Disentanglement for One-Shot Voice ConversionOne-shot voice conversion은 speech representation disentanglement를 통해 효과적으로 수행될 수 있음- BUT, 기존 방식은 speech representation 간의 correlation을 무시하므로 content information이 leakage 될 수 있음VQMIVCContent encoding 과정에서 vector quantization을 사용하고 training 중에 correlation metric으로써 mutu..
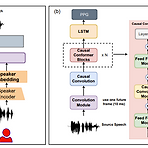
ALO-VC: Any-to-Any Low-Latency One-Shot Voice ConversionNon-parallel low-latency one-shot phonetic posteriorgrams-based voice conversion을 통해 빠른 합성이 가능함ALO-VCPre-trained speaker encoder, pitch predictor, positional encoding을 결합해 구성됨ALO-VC-R은 pre-trained d-vector speaker encoder를 활용하고 ALO-VC-E는 ECAPA-TDNN을 활용해 성능을 개선논문 (INTERSPEECH 2023) : Paper Link1. IntroductionVoice Conversion (VC)는 linguistic..
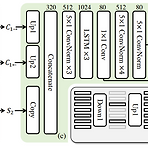
AutoVC: Zero-Shot Voice Style Transfer with Only Autoencoder LossMany-to-Many voice conversion, zero-shot conversion을 위해 Generative Adversarial Network나 conditional Variational AutoEncoder를 활용할 수 있음AutoVCBottleneck이 포함된 AutoEncoder에 기반한 style transfer 방식을 도입Self-reconstruction loss에 대해서만 training 함으로써 distribution-matching style transfer를 수행가능논문 (ICML 2019) : Paper Link1. IntroductionVoice Conve..
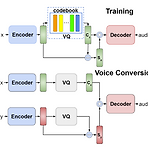
VQVC: One-Shot Voice Conversion by Vector QuantizationSpeaker label에 대한 supervision 없이 voice conversion을 수행할 수 있음VQVCContent embedding을 discrete code로 모델링하고 quantize-before/quantize-after vector 간의 차이를 speaker embedding으로 취급Vector quantization에 대한 reconstruction loss 만으로 content/speaker information에 대한 strong disentanglement를 달성논문 (ICASSP 2020) : Paper Link1. IntroductionVoice Conversion (VC)는 l..
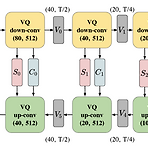
VQVC+: One-Shot Voice Conversion by Vector Quantization and U-Net ArchitectureAutoEncoder-based voice conversion은 speaker identity와 input speech content를 disentangle 하여 unseen speaker에 대해 generalize 됨- BUT, imperfect disentanglement로 인해 합성 품질의 한계가 있음VQVC+AutoEncoder-based system에 대해 U-Net architecture를 도입해 conversion 품질을 향상Strong information bottleneck을 위해 latent vector를 quantize 하는 vector quant..