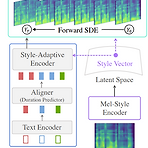
Grad-StyleSpeech: Any-Speaker Adaptive Text-to-Speech Synthesis with Diffusion Models Any-speaker adaptive Text-to-Speech 작업은 여전히 target speaker의 style을 모방하기에 만족스럽지 못함 Grad-StyleSpeech Diffusion model을 기반으로 하는 any-speaker adaptive Text-to-Speech model Few-second reference speech가 주어지면 target speaker와 유사한 음성을 생성하는 것을 목표로 함 논문 (ICASSP 2023) : Paper Link 1. Introduction Text-to-Speech (TTS)는 single..
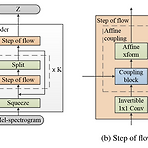
Flow-TTS: A Non-Autoregressive Network for Text to Speech Based on Flow Non-autoregressive Text-to-Speech를 위해 generative flow를 활용할 수 있음 Flow-TTS Single feed-forward network 만을 사용하여 고품질의 음성을 합성 Spectrum 생성을 위해 flow를 활용하고 single network를 통해 alignment와 spectrogram 생성을 jointly learn 논문 (ICASSP 2020) : Paper Link 1. Introduction Text-to-Speech (TTS)는 input text sequence $\{ x_{1}, x_{2}, ..., x_{N}\}..
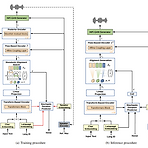
YourTTS: Towards Zero-Shot Multi-Speaker TTS and Zero-Shot Voice Conversion for Everyone Zero-Shot multi-speaker Text-to-Speech를 위해 multilingual approach가 필요 YourTTS VITS를 기반으로 multi-speaker, multilingual task로 확장 Low-resource zero-shot 환경에서 우수한 합성 품질을 달성하고 1분 미만으로 fine-tuning이 가능 논문 (ICML 2022) : Paper Link 1. Introduction 대부분의 Text-to-Speech (TTS) 모델은 single speaker의 음성에만 특화되어 있음 이때 Zero-Shot ..
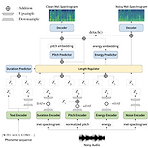
STYLER: Style Factor Modeling with Rapidity and Robustness via Speech Decomposition for Expressive and Controllable Neural Text to Speech Text-to-Speech는 어려운 합성 condition에 대한 robustness와 expressiveness, controllability를 요구함 STYLER Mel-Calibrator를 통한 audio-text aligning을 도입하여 unseen data에 대한 robust 한 추론을 가능하게 함 Supervision 하에서 disentangled style factor modeling을 통해 controllability를 향상 Domain adve..
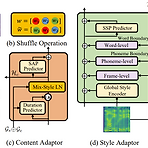
GenerSpeech: Towards Style Transfer for Generalizble Out-of-Domain Text-to-Speech Out-of-Domain 음성 합성을 위해 style transfer를 활용할 수 있지만 몇 가지 한계가 존재함 - Expressive voice의 dynamic style feature는 모델링과 transfer가 어려움 - Text-to-Speech 모델은 source data와 다른 Out-of-Domain condition을 handle 할 수 있을 만큼 robust 해야 함 GenerSpeech Out-of-Domain custom voice에 대해 high-fidelity zero-shot style transfer를 가능하게 하는 text-to-s..
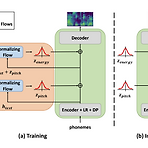
VarianceFlow: High-Quality and Controllable Text-to-Speech using Variance Information via Normalizing Flow Text와 speech 간의 one-to-many 관계를 학습하기 위해 두 가지 방식을 활용할 수 있음 - Normalizing Flow의 사용 - 합성 과정에서 pitch, energy 같은 variance information의 반영 VarianceFlow Normalizing Flow를 통해 variance를 모델링하여 더 정확하게 variance information을 예측 Normalizing Flow의 objective function은 variance와 text를 disentangle 하여 varianc..