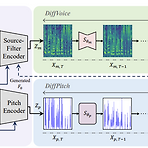
Diff-HierVC: Diffusion-based Hierarchical Voice Conversion with Robust Pitch Generation and Masked Prior for Zero-Shot Speaker AdaptationVoice Conversion은 여전히 inaccurate pitch와 low speaker adaptation 문제를 가지고 있음Diff-HierVC2가지 diffusion model을 기반으로 하는 hierarchical voice conversion model- Target voice style로 $F_{0}$를 효과적으로 생성할 수 있는 DiffPitch를 도입하고,- 이후 생성된 $F_{0}$를 DiffVoice에 전달하여 target voice styl..
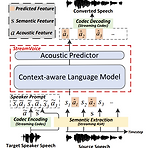
StreamVoice: Streamable Context-Aware Language Modeling for Real-time Zero-Shot Voice ConversionLanguage Model을 활용하여 zero-shot voice conversion 성능을 향상할 수 있음- BUT, 기존 방식은 offline conversion으로 인해 complete source speech 만을 요구하므로 real-time application에서 활용하기 어려움StreamVoiceStreaming capability를 위해 temporal independent acoustic predictor를 포함한 fully causal context-aware Language Model을 도입- 이를 통해 comple..
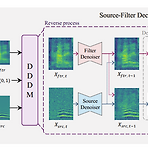
DDDM-VC: Decoupled Denoising Diffusion Models with Disentangled Representation and Prior Mixup for Verified Robust Voice ConversionDiffusion-based model은 data distribution에 많은 attribute가 존재하고 generation process에서 model parameter sharing에 대한 한계로 인해 각 attribute에 대한 specific style control이 어려움DDDM-VCDecoupled Denoising Diffusion Model을 도입하여 각 attribute에 대한 style transfer를 지원- 특히 voice conversion ta..
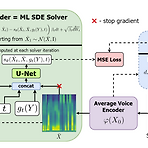
DiffVC: Diffusion-based Voice Conversion with Fast Maximum Likelihood Sampling SchemeOne-shot many-to-many voice conversion은 source/target speaker가 모두 training dataset에 속하지 않은 경우에 대해서 single reference utterance의 target voice를 copy 하는 것을 목표로 함DiffVCDiffusion probabilistic modeling을 기반으로 scalable one-shot voice conversion을 수행추가적으로 diffusion model을 가속할 수 있는 Stochastic Differential Equation solver를 ..
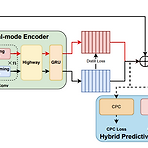
DualVC: Dual-mode Voice Conversion Using Intra-model Knowledge Distillation and Hybrid Predictive Coding일반적인 non-streaming voice conversion은 전체 utterance를 full context로 활용할 수 있지만, streaming voice conversion은 future information이 제공되지 않으므로 품질이 상당히 저하됨DualVCJointly trained separate network parameter를 활용하여 streaming/non-streaming mode를 지원하는 dual-mode conversion을 활용Streaming conversion의 성능을 향상하기 위해 i..
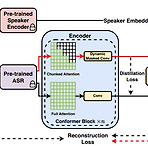
DualVC2: Dynamic Masked Convolution for Unified Streaming and Non-Streaming Voice Conversion기존의 DualVC는 streaming inference를 위해 streaming architecture, intra-model knowledge distillation, hybrid predictive coding을 활용함BUT, autoregressive decoder는 error accumulation의 문제가 있고 추론 속도가 제한적임- Causal convolution은 chunk 내의 future information을 효과적으로 사용할 수 없음- Unvoiced segment의 noise를 효과적으로 처리할 수 없어 음성 품질이 ..