티스토리 뷰
Paper/Conversion
[Paper 리뷰] ExVC: Leveraging Mixture of Experts Models for Efficient Zero-Shot Voice Conversion
feVeRin 2025. 3. 19. 21:18반응형
ExVC: Leveraging Mixture of Experts Models for Efficient Zero-Shot Voice Conversion
- Zero-shot voice conversion은 short target reference를 사용하는 경우 quality와 similarity를 balancing 하기 어려움
- ExVC
- Mixture of Experts layer와 Conformer module을 결합하여 zero-shot expressiveness를 향상
- Model을 speaker embedding에 효과적으로 conditioning 하기 위해 Feature-wise Linear Modulation을 도입
- 논문 (ICASSP 2025) : Paper Link
1. Introduction
- Voice Conversion (VC)는 speech content는 keeping 하면서 speaker identity를 target person의 vocal identity로 modify 하는 것을 목표로 함
- 이때 VC model은 speech signal을 timbre/linguistic content와 같은 distinct component로 disentangle 할 수 있어야 함
- 특히 zero-shot VC는 unseen speaker에 대해 short reference speech를 사용하여 VC task를 수행함 - BUT, zero-shot VC는 short reference speech와 Out-of-Domain generalization 문제가 존재함
- 이를 해결하기 위해 AutoVC는 bottleneck structure를 활용하여 speech feature를 disentangle 하고, YourTTS, SC-GlowTTS 등은 normalizing flow-based Text-to-Speech (TTS) system을 활용함
- 한편으로 FreeVC와 같이 large pre-trained model의 Self-Supervised Learning feature를 활용할 수도 있음
- BUT, 여전히 voice quality와 speaker similarity 측면에서 한계가 있음
- 이때 VC model은 speech signal을 timbre/linguistic content와 같은 distinct component로 disentangle 할 수 있어야 함
-> 그래서 Mixture of Experts를 활용하여 zero-shot expressiveness를 개선한 VC model인 ExVC를 제안
- ExVC
- Mixture of Experts (MoE)와 Conformer module을 결합하여 expressive capacity를 향상
- 추가적으로 model conditioning을 개선하기 위해 speaker embedding을 통해 model을 modulate 하는 Feature-wise Linear Modulation (FiLM)을 도입
- Mixture of Experts (MoE)와 Conformer module을 결합하여 expressive capacity를 향상
< Overall of ExVC >
- MoE와 FiLM을 결합한 zero-shot VC model
- 결과적으로 기존보다 뛰어난 conversion 성능을 달성
2. Method
- System Overview
- ExVC는 2개의 FiLM module 사이에 MoE-enhanced Coformer module stack을 sandwich 하고 HiFi-GAN vocoder를 추가하여 구성됨
- Vanilla Conformer module은 2개의 Feed-Forward Network (FFN), Multi-Head Attention (MHA), convolution layer로 구성됨
- 여기서 MoE layer를 integrate 하기 위해 second FFN layer를 MoE layer로 replace 함 - Training 중에 WavLM으로 추출한 speech SSL feature는 MoE-enhanced Conformer encoder로 전달되기 전에 FiLM module을 통해 speaker encoder의 speaker embedding에 따라 condition 됨
- Encoder의 latent representation은 speaker embedding에 따라 further condition 되고 HiFi-GAN vocoder로 전달되어 converted speech를 생성함
- 추론 시에는 source speech 대신 target reference가 사용되고, 1개의 Conv1D layer adapter가 SSL feature, Conformer module input 사이에 insert 되어 encoder dimension과 match 되도록 scale down 함
- Vanilla Conformer module은 2개의 Feed-Forward Network (FFN), Multi-Head Attention (MHA), convolution layer로 구성됨
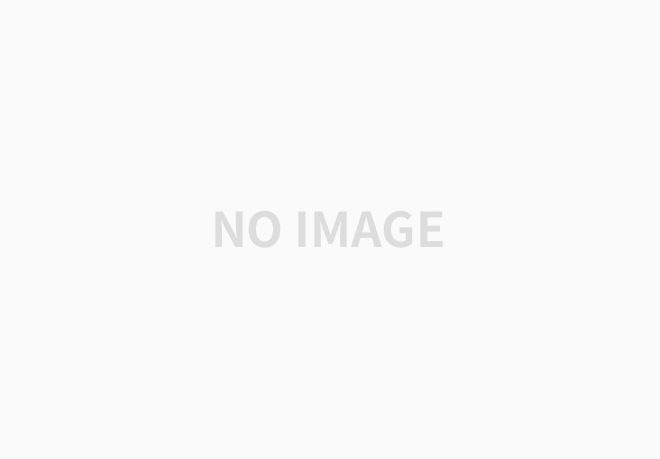
- Mixture of Experts Layer
- Mixture of Experts (MoE)는 input sample을 most appropriate 'experts' 에 route 하는 gating mechanism을 사용하여 modeling problem을 smaller subspace로 divide 하는 방식임
- 이때 MoE layer는 expert network set과 gating network로 구성됨
- 먼저
expert set 과 gating network가 주어지면, 모든 forward pass에서 gating network는 다음과 같이 expert weight를 추정함:
(Eq. 1)
- : -th expert의 weight, : gate network의 trainable weight, : input - 그러면 layer output은 weighted summation으로 얻어짐:
(Eq. 2)
- : total experts 수
- 먼저
- 구조적으로는 Conformer model의 second FFN layer를 4 experts로 구성된 dense MoE layer로 대체하여 사용함
- 이때 MoE layer는 expert network set과 gating network로 구성됨
- Feature-wise Linear Modulation
- ExVC는 Feature-wise Linear Modulation (FiLM)을 사용하여 speaker embedding을 통해 model을 condition 하는 방식으로 speaker similarity를 향상함
- 먼저 speaker embedding vector
가 주어지면 FiLM은 scale/shift parameter 를 생성하는 function 를 학습함 - 그러면 해당 parameter는 input을 다음과 같이 scale/shift 함:
(Eq. 3)
- : Hadamard product, : FiLM layer output - ExVC의 FiLM layer는 input으로 256-dimensional speaker embedding을 사용하고 384-dimension을 output 하는 Conv1D layer로 구성됨
- 해당 output은 각각 192-dimension을 갖는 로 split 되어 (Eq. 3)과 같이 input을 scale/shift 함
- 먼저 speaker embedding vector
- Voice Conversion-based Data Augmentation
- Same person의 semantic/speaker information으로 VC model을 training 하고 추론 시에는 다른 speaker information을 사용하는 경우 content/timbre의 proper disentanglement를 보장하기 어려움
- 따라서 논문은 Vec-Tok-VC+와 같이 pre-trained FreeVC model을 사용하여 VCTK, LibriTTS에서 derive 된 새로운 parallel dataset을 synthetically generate 함
- 즉, VCTK의 sample을 source speech로, LibriTTS의 distinct speaker에 대한 700 random waveform을 target speaker로 사용함
- 각 VCTK waveform은 다른 target speaker를 사용하여 7개 version으로 변환되어 semantically aligned waveform을 형성함
- Training 중에 synthetic sample은 input으로 사용되고 original VCTK sample은 ground-truth로 사용됨
- Testing은 training data에 포함되지 않은 speaker를 사용하여 수행됨
- Training Loss
- ExVC는 VITS의 adversarial training approach를 따라 end-to-end train 됨
- 그러면 overall generator loss는:
(Eq. 4)
- : generated/ground-truth mel-spectrogram 간의 reconstruction loss
- : discriminator의 intermediate feature에 대한 loss
- : adversarial training loss
- : loss scaling hyper-parameter
- 그러면 overall generator loss는:
3. Experiments
- Settings
- Results
- 전체적으로 ExVC의 성능이 가장 뛰어남

- CSIM, WER, CER 측면에서도 ExVC가 가장 우수함
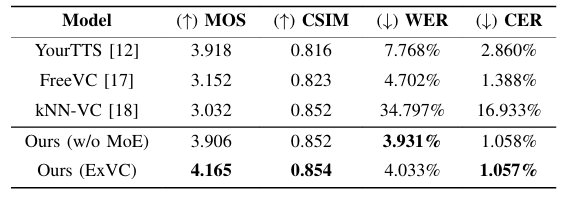
- ExVC는 FreeVC 보다 52% 적은 parameter 수를 가짐
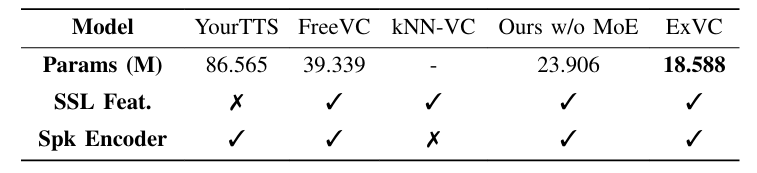
-SNE 측면에서 ExVC embedding은 다른 model에 비해 condensed cluster를 형성함

- Effect of Reference Speech Length
- MOS, CSIM은 reference speech length가 길어질수록 향상됨
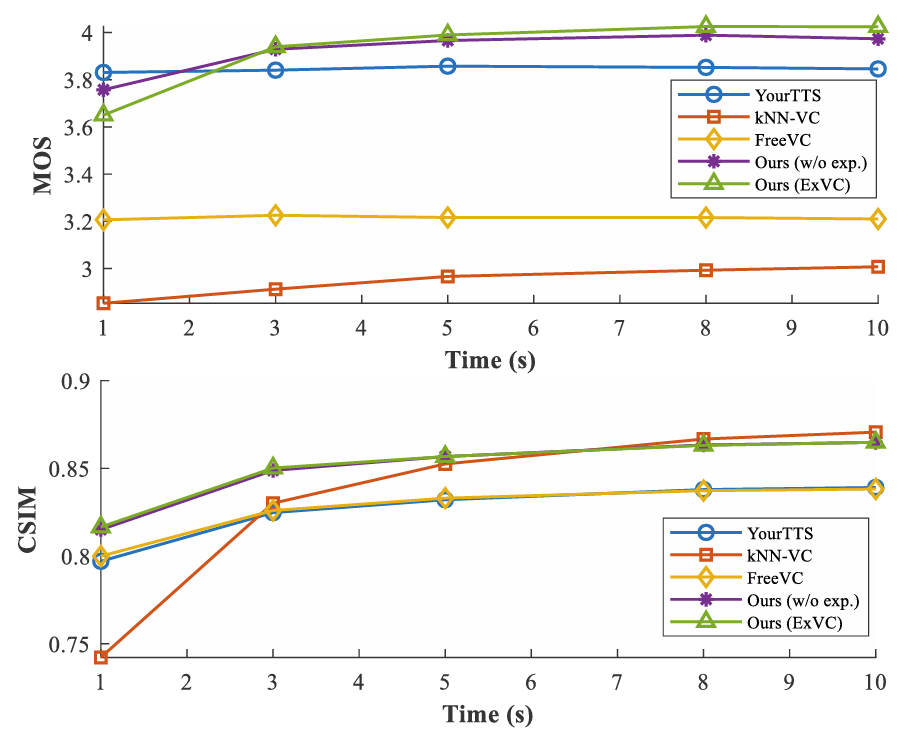
반응형
'Paper > Conversion' 카테고리의 다른 글
댓글