티스토리 뷰
Paper/Representation
[Paper 리뷰] Data2Vec 2.0: Efficient Self-Supervised Learning with Contextualized Target Representations for Vision, Speech and Language
feVeRin 2025. 4. 6. 09:44반응형
Data2Vec 2.0: Efficient Self-Supervised Learning with Contextualized Target Representations for Vision, Speech and Language
- Self-supervised learning을 위해서는 상당한 computational resource가 필요함
- Data2Vec 2.0
- Data2Vec을 기반으로 rich contextualized target representation을 얻고,
- Fast convolutional decoder를 통해 teacher representation을 build 하는데 필요한 effort를 amortize 함
- 논문 (ICML 2023) : Paper Link
1. Introduction
- Self-supervised learning은 computer vision, Natural Language Processing (NLP), speech processing 등의 다양한 task에서 활용되고 있음
- BUT, 대부분의 approach는 single-modality만을 고려하므로 동일한 mechanism을 여러 modality에 대해 generalize 할 수 없음
- 따라서 Data2Vec은 다양한 modality에서도 동일하게 동작할 수 있는 self-supervised framework를 도입함 - 한편으로 self-supervised model은 large model capacity/training dataset을 활용하여 few-shot learner로써 기능할 수 있음
- BUT, 이를 위해서는 수천억 parameters를 가지는 model이 필요하므로 computationally unfeasible 하고 self-supervised learning stage의 efficiency가 저해됨
- BUT, 대부분의 approach는 single-modality만을 고려하므로 동일한 mechanism을 여러 modality에 대해 generalize 할 수 없음
-> 그래서 self-supervised learning의 efficiency를 향상한 Data2Vec 2.0을 제안
- Data2Vec 2.0
- Self-supervised learning의 efficiency를 향상하기 위해 Data2Vec을 기반으로 efficient data encoding, fast convolutional decoder, target representation reuse를 도입
- 각 modality에 대해 동일한 learning objective를 사용하는 대신, input modality에 따라 서로 다른 feature encoder를 가지는 Transformer architecture를 활용하여 각 modality에 대한 separate model을 학습
- 추가적으로 teacher model을 기반으로 unmasked training example에 대한 latent contextualized representation을 생성하여, masked sample을 input으로 하는 student model을 통한 regress를 지원
< Overall of Data2Vec 2.0 >
- Data2Vec을 기반으로 learning efficiency를 개선한 self-supervised framework
- 결과적으로 다양한 modality에 대해 기존보다 더 뛰어난 성능을 달성
2. Method
- Data2Vec을 기반으로 Masked AutoEncoder (MAE)와 비슷하게, sample의 non-masked portion만 encoding 하고 decoder model을 사용하여 masked portion에 대한 target representation을 predict 함
- 이때 Transformer-based decoder 대신 easier/faster training이 가능한 smaller convolutional decoder를 도입함
- Contextualized target representation을 얻는데 필요한 computational overhead를 줄이기 위해, 각 target을 training sample의 multiple masked version에 reuse 함
- 추가적으로 inverse block masking strategy를 통해 student prediction에 대한 더 많은 context를 제공함
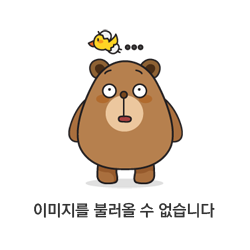
- Contextualized Target Prediction
- 논문은 raw input data의 local window를 reconstruct 하거나 discrete representation을 predict 하는 대신, enitre input sample의 information을 incorporate 한 teacher network를 구성함
- 이를 통해 target이 특정 training sample에 specify 된 richer training task를 얻을 수 있음
- 결과적으로 contetualized target은 unmasked training sample을 encoding 하는 Transformer-based teacher model의 self-attention mechanism을 통해 얻어짐
- 이때 training target은 sample의 all feature의 weighted sum과 같음 - Target Representation and Learning Objective
- Training target은 teacher의 top-
FFN block의 averaging을 기반으로 함K
- Averaging 이전에 activation은 instance normalization을 통해 normalize 됨 - Training objective는 student network가 sample의 masked version을 사용하여 해당 target을 regress 하는 것을 목표로 함
- Training target은 teacher의 top-
- Teacher Weights
- Teacher weight
는 student encoder weightΔ 에 대한 exponentially moving averageθ Δ←τΔ+(1−τ)θ - 여기서
는 starting valueτ 에서 final valueτ0 까지τe update를 통해 linearly increase 하는 schedule을 따르고, 이후에는 constant value로 keeping 됨τn
- Teacher weight
- Learning Objective
- Teacher network
의 target representation과 student network predictiony 에 대한f(x) loss를 사용함L2 - 이는 기존 Data2Vec에서 사용된 Smooth
loss에 비해 simplify 된 것으로, 다양한 modality에 대해 효과적으로 동작함L1
- Teacher network
- Model Architecture
- Data2Vec 2.0은 modality-specific feature encoder와 Transformer architecture를 사용함
- Computer vision의 경우,
pixel의 patch matching을 feature encoder로 사용하고, speech의 경우 multi-layer convolutional network, text의 경우 Byte-Pair Encoding에 기반한 embedding을 사용함16×16 - Asymmetric Encoder/Decoder Architecture
- 먼저 teacher network를 사용하여 unmasked training sample의 all part를 encode 하여 training target을 만든 다음, sample의 일부를 mask 하고 student encoder에 embed 함
- 이때 efficiency를 향상하기 위해 training example의 unmasked patch/time-step만 encoding 함 - 이후 student encoder output은 masked portion에 대한 fixed representation과 merge 되어 decoder network에 전달됨
- Masked token을 represent 하기 위해 learned representation 대신 random Gaussian noise를 사용함 - 최종적으로 decoder network는 student input에서 masked time-step에 대한 teacher network의 contextualized target representation을 reconstruct 함
- 먼저 teacher network를 사용하여 unmasked training sample의 all part를 encode 하여 training target을 만든 다음, sample의 일부를 mask 하고 student encoder에 embed 함
- Convolutional Decoder Network
- 논문은
convolution으로 구성된 lightweight decoder를 사용함D
- 각각은 layer normalization, GELU activation, residual connection을 포함함 - Speech/text와 같은 sequential data의 경우 1D convolution을 사용하고 image의 경우 2D convolution을 사용함
- 이때 각각은 efficiency를 향상하기 위해 group별로 parameterize 됨
- 논문은
- Computer vision의 경우,
- Multi-Mask Training
- 기존 Data2Vec의 teacher-student setup은 각 sample을 twice processing 해야 한다는 단점이 있음
- 즉, teacher model로 target을 얻은 다음 student로 prediction을 얻어야 함
- 특히 teacher model은 full unmasked input을 process 해야 하므로 student model에 비해 less efficient 함 - 따라서 Data2Vec 2.0은 teacher model computation을 amortize 하기 위해 training sample의 multiple masked version에 대한 teacher representation을 reuse 함
- 이를 위해 training sample의
different masked version을 사용하여 same target representation에 대한 loss를 compute 함M
- 이는 target representation이 sample의 full unmasked version에 기반하기 때문 - 결과적으로
이 증가함에 따라 target representation에 대한 computational overhead를 negligible 할 수 있음M
- 즉, 기존 self-supervised method에 비해 small batch size로도 training이 가능함
- 이를 위해 training sample의
- 추가적으로 논문은 redundant computation을 avoid 하기 위해 training example의 different masked version에서 feature encoder output을 share 함
- 이를 통해 feature encoder가 computation에 많은 부분을 차지하는 speech와 같은 dense modality에서 speed up 효과를 얻을 수 있음
- 즉, teacher model로 target을 얻은 다음 student로 prediction을 얻어야 함
- Inverse Block Masking
- Masked AutoEncoder (MAE)-style sample encoding은 masked time-step에 information을 store 할 수 있는 ability를 제거하므로 training task를 어렵게 만듦
- Random masking은 MAE에서 효과적으로 동작할 수 있지만, 생성된 mask에 대한 structure가 없으므로 semantic representation을 build 하는 것이 어려움
- Block masking의 경우 time-step/patch의 entire block을 masking 하여 structured 할 수 있지만 training sample의 large continguous portion을 unmask 한다는 보장이 없음 - 따라서 논문은 student model이 sample의 local region에 대해 semantically rich representation을 build 할 수 있도록 Inverse Block Masking을 도입함
- 이때 어떤 patch를 mask 할지를 choice 하는 대신, block-wise fashion으로 어떤 patch를 preserve 할지를 choice 함
- Block size는 patch 수/time-step 에 따라 결정됨B - 먼저 각 block의 starting point를 sampling 하고,
- Speech/text의 경우, block width가 가 될 때까지 symmetrically exapnd 함B
- Image의 경우, 가 될 때까지 expand 함√B - 이후 다음 starting point를 replacement 없이 sampling 하여, modality에 따라 width
/widthB 의 quadratic block으로 expand 함:√B
(Eq. 1)L×(1−R)+AB
- : training sample의 total time-step/patch 수L
- : mask ratio,R : mask ratio를 adjust 하는 hyperparameterA
- 이때 어떤 patch를 mask 할지를 choice 하는 대신, block-wise fashion으로 어떤 patch를 preserve 할지를 choice 함
- Block이 overlap 되는 경우, over-masking과 각 sample에 대한 actually masked time-step 수의 variance가 발생함
- 따라서 Unmasked time-step만 encoding 하기 위해, batch의 all sample에 대한 unmasked time-step 수를 assimilate 하는 strategy를 도입함
- 즉, 각 sample에 대해 mask/unmask 할 individual time-step을 randomly choice 하고, desired unmask time-step 수
에 reach 할 때까지 반복함L×(1−R)
- Random masking은 MAE에서 효과적으로 동작할 수 있지만, 생성된 mask에 대한 structure가 없으므로 semantic representation을 build 하는 것이 어려움
3. Experiments
- Settings
- Dataset
- Vision : ImageNet
- Speech : LibriSpeech
- NLP : Books, English Wikipedia - Comparisons : Data2Vec
- Vision : BEiT, PeCo, BEiT-2, TEC, MoCo, DINO, MAE, SimMIM, iBOT, MaskFeat
- Speech : Wav2Vec 2.0, HuBERT, WavLM
- NLP : BERT
- Results
- Efficiency
- Data2Vec 2.0은 각 modality에 대해 더 나은 speed, accuracy trade-off를 가짐
- Vision의 경우, 50.7 hours의 MAE 보다
더 빠른 3 hours training 만으로도 83.7%의 accuracy를 달성할 수 있음16.4× - Speech의 경우 Wav2Vec 2.0 보다
더 빠르고 NLP는10.6× 더 적은 training time을 소모함2.9×
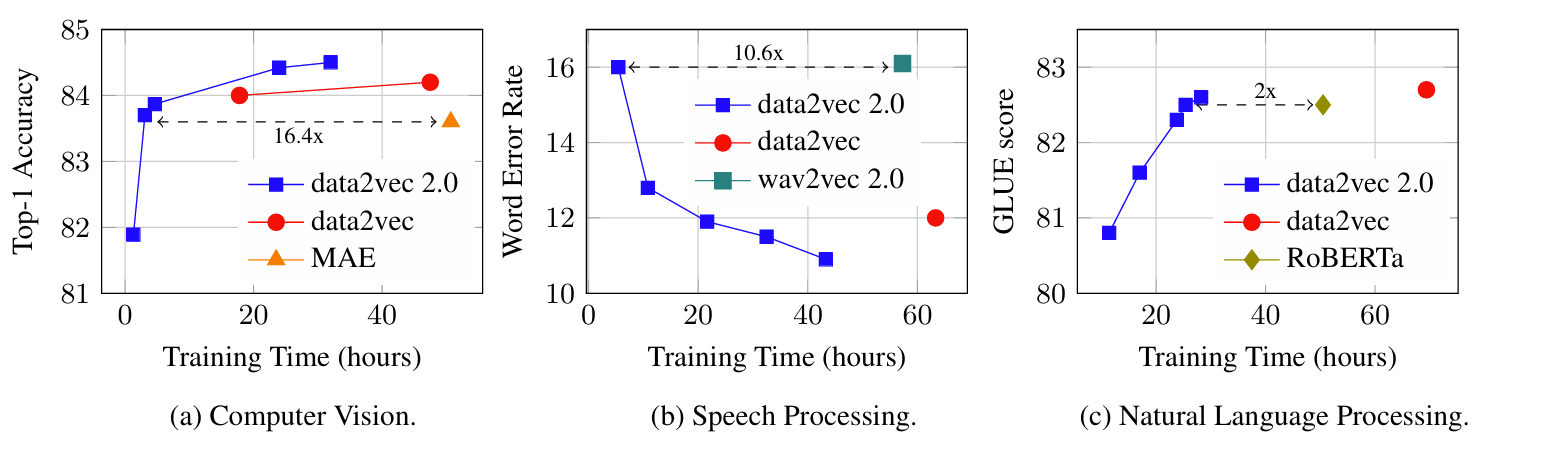
- Computer Vision
- ViT-B, ViT-L 측면에서 Data2Vec 2.0이 가장 우수한 성능을 달성함
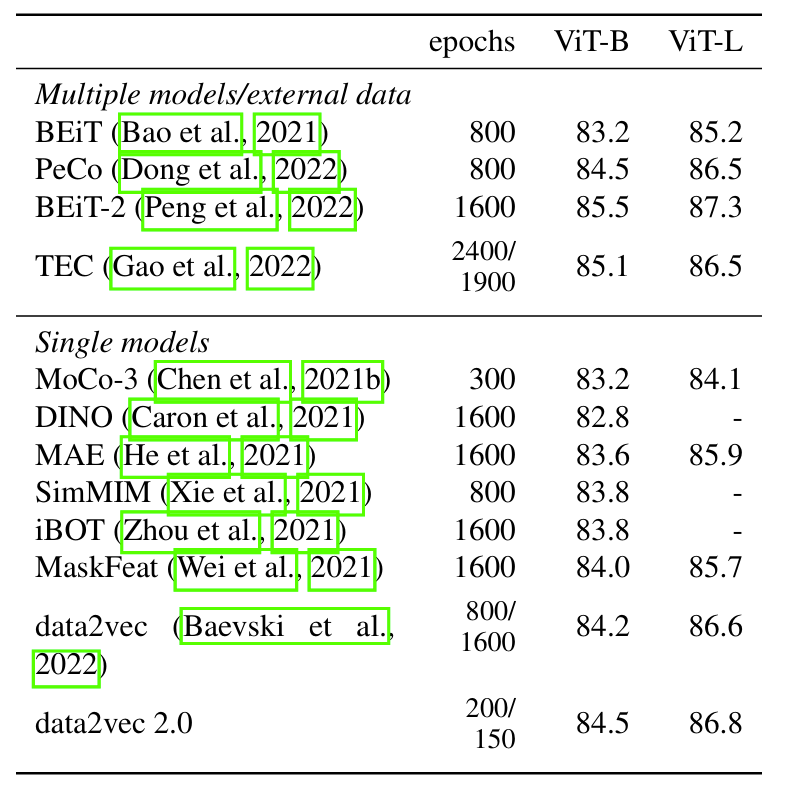
- Data2Vec 2.0은 MAE 보다 더 적은 epoch과 training time으로 더 나은 성능을 달성할 수 있음
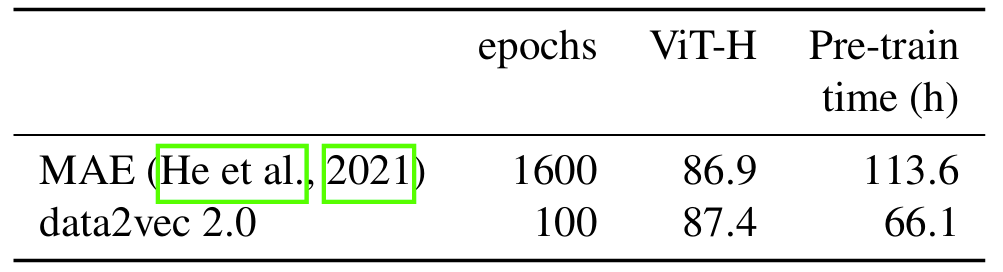
- Speech Processing
- Speech processing 측면에서도 Data2Vec 2.0이 가장 뛰어남
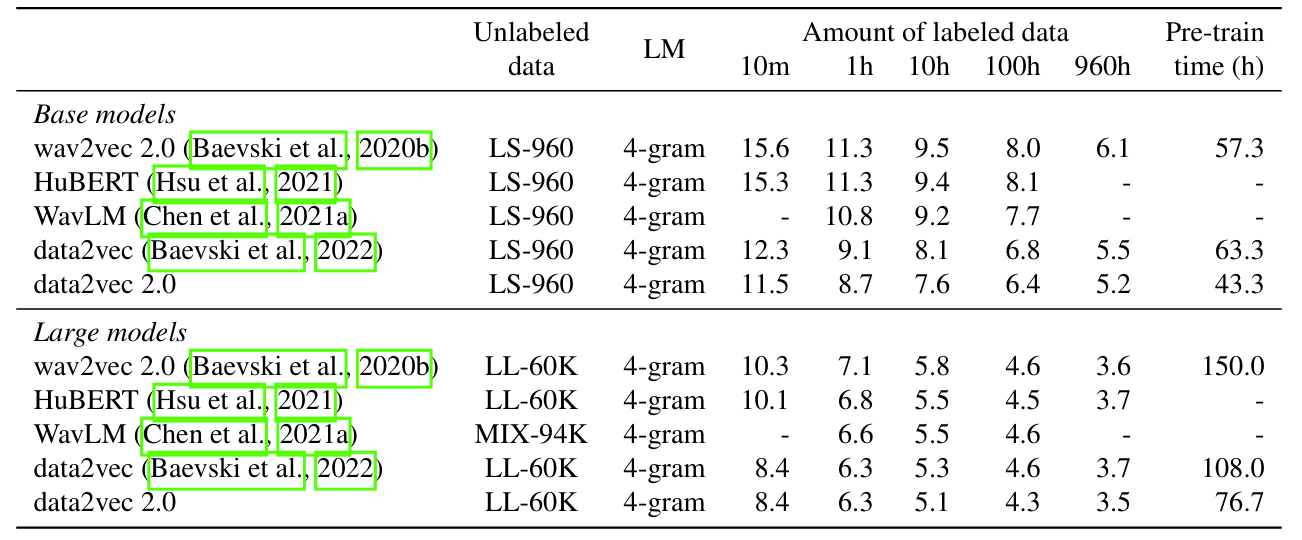
- Natural Language Processing
- NLP 측면에서도 Data2Vec 2.0이 가장 우수한 성능을 보임

- Multi-Mask Training
- Training sample 당 multiple mask를 사용할 때 accuracy가 크게 향상됨
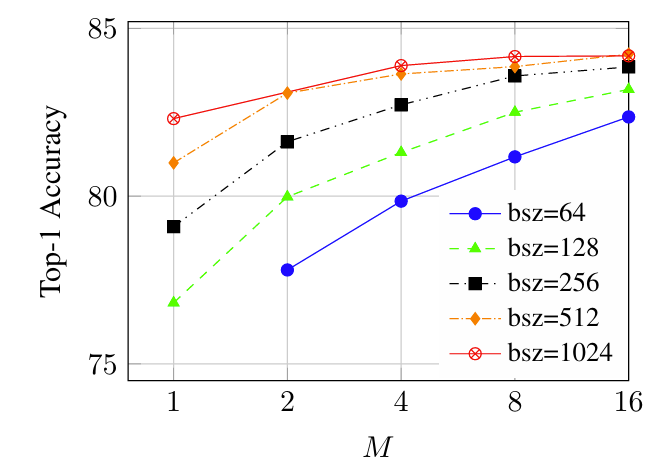
- Training Loss
- CLS loss component는 computer vision task의 accuracy를 향상할 수 있음
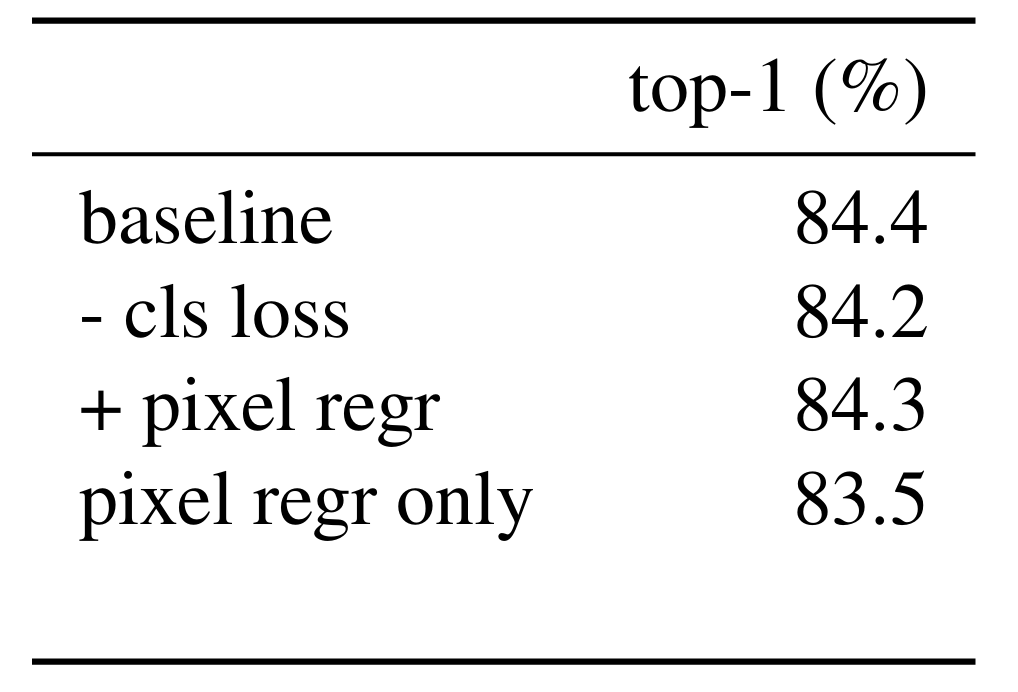
- Masking Strategy
일 때 inverse block masking은 최적의 성능을 달성할 수 있고,B=3 인 경우 오히려 random masking 보다 낮은 성능을 보임B=1
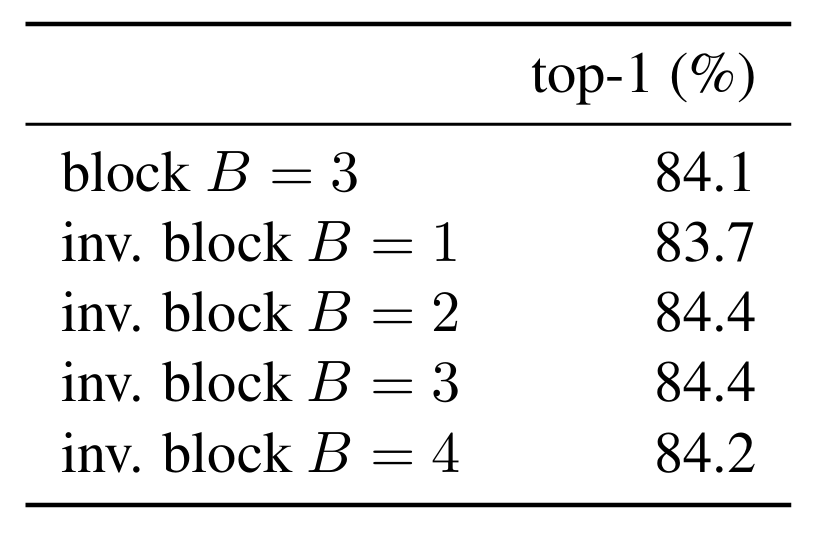
- Speech Alibi Embedding
- Alibi Embedding을 제거하는 경우 성능 저하가 발생함
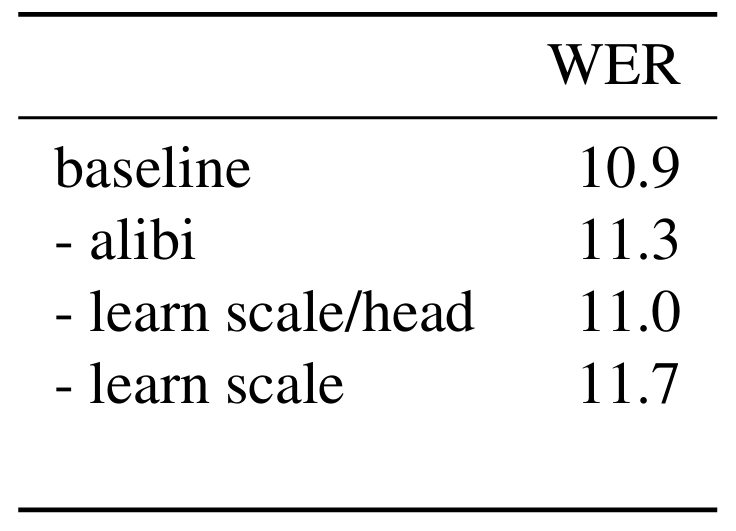
반응형