티스토리 뷰
Paper/Language Model
[Paper 리뷰] SpeechX: Neural Codec Language Model as a Versatile Speech Transformer
feVeRin 2025. 1. 25. 12:26반응형
SpeechX: Neural Codec Language Model as a Versatile Speech Transformer
- Audio-text prompt 기반의 speech model은 text-to-speech 외의 다양한 task를 처리하는 데는 한계가 있음
- SpeechX
- Zero-shot Text-to-Speech, Speech Editing, Noise Suppression, Target Speaker Extraction 등의 다양한 task를 지원하는 speech model
- Neural codec language modeling과 task-dependent prompting에 기반한 multi-task learning을 도입
- 논문 (TASLP 2024) : Paper Link
1. Introduction
- Speech domain에서 audio-text input을 활용하는 대표적인 task에는 zero-shot Text-to-Speech (TTS)가 있음
- Zero-shot TTS는 주어진 text를 brief audio sample만을 사용하여 desired talker의 speaking style을 가진 speech로 변환하는 것을 목표로 함
- 이를 위해 YourTTS, SC-GlowTTS 등은 fixed-dimensional speaker embedding을 도입했음
- 반면 최근에는 VoiceBox와 같이 masked speech prediction을 사용하거나 VALL-E, SPEAR-TTS 등과 같이 neural codec language modeling을 도입하고 있음
- 해당 방식들을 fixed-dimensional representation으로 compressing 하지 않고 target audio를 직접 활용함 - 결과적으로 zero-shot TTS 뿐만 아니라 speech editing, voice conversion 등의 task도 수행할 수 있음
- BUT, 해당 model들은 여전히 한계점이 존재하고 다양한 audio-text-based speech generation task를 처리하지 못함
- Clean signal만 처리할 수 있거나, practical application에서 적용하기 어렵거나, target speaker extraction과 같은 task를 지원하지 못함 - 특히 이를 위한 unified audio-text-based speech model은 다음의 property를 만족해야 함:
- Versatility
- Unified audio-text-based generative model은 audio, text input에 대한 여러 task들을 처리할 수 있어야 함
- 즉, zero-shot TTS, speech enhancement, speech editing 등 다양한 task를 지원해야 함 - Robustness
- Acoustically challenging environment와 다양한 distrotion에 대해 robust 해야 함
- 즉, reliable performance를 보장하여 real-world scenario에 적용될 수 있어야 함 - Extensibility
- Flexible architecture를 기반으로 seamless extension이 가능해야 함
- 즉, 다양한 generation task에 efficiently adapt 해야 함
- Versatility
- Zero-shot TTS는 주어진 text를 brief audio sample만을 사용하여 desired talker의 speaking style을 가진 speech로 변환하는 것을 목표로 함
-> 그래서 앞선 property를 만족하면서 다양한 speech task를 처리할 수 있는 SpeechX를 제안
- SpeechX
- Textual/acoustic input을 기반으로 neural codec model의 code/acoustic token을 생성하는 language modeling 방식을 채택
- 추가적으로 다양한 task를 처리할 수 있도록 multi-task learning setup과 additional token을 지원
< Overall of SpeechX >
- Neural codec language modeling을 기반으로 다양한 generation task를 지원하는 audio-text-based speech model
- 결과적으로 zero-shot TTS, speech editing, speaker extraction 등 다양한 task에서 기존보다 뛰어난 성능을 달성
2. Method
- Overview
- SpeechX는 VALL-E의 principle을 따라 Transformer를 기반으로 하는 neural codec language model을 활용함
- 전체적으로 textual prompt
와 acoustic promptT 에 대한 2개의 input prompt를 기반으로 neural code sequenceA 에 대한 conditional generation을 학습함O
- 먼저 textual prompt
는 input text에 grapheme-to-phoneme conversion을 적용하여 얻어지는 phoneme sequence를 의미함T
- Textual prompt는 semantic information을 convey 하므로 semantic token으로 볼 수 있음 - Acoustic prompt
는 input speech signal의 acoustic information을 encapsulate 함A
- Neural codec encoder를 사용해 input audio를 acoustic token sequence로 변환하여 얻어짐 - 추가적으로 SpeechX는 task-specifying을 위해 acoustic prompt에 additional token을 incorporate 함
- 결과적으로 output
는 desired signal의 neural code가 되고 codec decoder를 통해 waveform으로 변환됨O
- 먼저 textual prompt
- 논문은 neural codec model로써 EnCodec을 채택함
- EnCodec은
quantization layer가 있는 encoder-decoder architecture를 기반으로 구성됨L
- SpeechX는 을 사용L=8 - 결과적으로 EnCodec의 각 layer는 75Hz sampling rate에서 1024 entry로 구성된 discrete code를 생성함
- EnCodec은
- SpeechX의 architecture는 neural language modeling approach의 end-to-end modeling capability를 극대화함
- 즉, 다른 zero-shot TTS model이나 speech generation model과 달리 speaker embedding model이나 duration model이 필요하지 않음
- 전체적으로 textual prompt
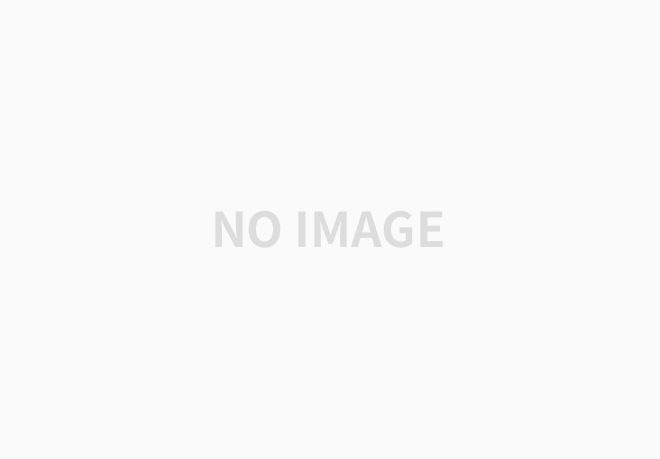
- Neural Codec Language Model
- VALL-E와 같이 SpeechX는 auto-regressive (AR)과 non-auto-regressive (NAR) Transformer를 활용함
- 구체적으로:
- AR model은 EnCodec의 first quantization layer에 해당하는 neural code를 추출하는 데 사용됨
- NAR model은 second layer부터 8th layer에 대한 neural code를 생성함
- Output
를 matrixO 로 represent 하자O=[ot,l]∈NT×L
- : time frameot,l 에서t -th codec layer의 code로써, 1024 value 중 하나를 취할 수 있음l
- : output sequence lengthT - 먼저 AR model은 transformer decoder layer의 stack으로 구성되고 desired output의 first layer code에 대한 negative log-likelihood를 minimizing 하여 최적화됨:
(Eq. 1)LAR=−∑Tt=1logP(ot,l|T,A,o<t,1;θAR)
- ,o<t,1=[o1,1,...,ot−1,1] : AR Transformer model parameterθAR
- Textual/acoustic token에는 서로 다른 embedding projection이 적용되고, sinusoidal positional embedding에 의해 superimpose 됨 - SpeechX의 AR model은 acoustic prompt
, textual promptA , past acoustic historyT 에 의해 결정됨o<t,1 - (Eq. 1)은 AR model이
에 의해서만 결정되고, 추론 시 audio prompt가T,o<t,1 의 일부로 represent 되는 VALL-E와는 다름o<t,1 - 구체적으로 VALL-E는 추론 시 audio prompt의 transcription과 textual prompt를 concatenate 하여
를 구성한 다음, audio prompt의 codec sequence를T 로 설정하여 audio를 생성함o<t,1
- BUT, SpeechX는 audio prompt의 transcription 없이도 codec sequence를 생성할 수 있음
- (Eq. 1)은 AR model이
- AR model을 통해 first layer code를 얻은 다음, NAR model을 기반으로 text, acoustic prompt와 생성된 first
layer의 output code를 활용하여l−1 -th layer code를 생성함l - 해당 model은
에 대해 repeatedly use 됨l=2,...,8 - 7개 layer에 동일한 NAR model을 사용하고, NAR model은 다음의 log-likelihood를 minimize 하도록 training 됨:
(Eq. 2)LNAR=−∑8l=2logP(o:,l|T,A,o:,<l;θNAR)
- : NAR model parameterθNAR
- :o:,l -th layer에 대한l 의 entire sequenceot,l
- :o:,<l [o:,1,...,o:,l−1] - Single NAR model이 7 layer 각각을 처리하기 위해, first부터
-th layer까지의 acoustic token(l−1) 이 embed 되고 summation 됨o:,<l
- 해당 model은
- 구체적으로:
- Task-based Prompting
- SpeechX는 하나의 model로 여러 task를 처리하기 위해 다음의 task-based prompting을 도입함
- Noise Suppression
- Noise Suppression은 noise-corrupted observation
에서 clean speech signals+n 를 추출하는 task를 의미함s - 해당 task의 경우, special token
를 포함한 acoustic prompt를 사용하여<ns> 을 만듦A=[<ns>,C(s+n)]
- : audio signal을 neural codec token sequence로 변환하는 데 사용되는 functionC(⋅) - Textual prompt
는 user가 reference transcription으로 제공할 수 있지만, human transcription이 unavailable 한 경우를 위해 textual prompt의 사용을 optional 하게 제공함T - 결과적으로 Noise Suppression task의 desired output은 clean audio의 acoustic token sequence
가 됨C(s)
- Noise Suppression은 noise-corrupted observation
- Speech Removal
- Speech Removal은 noisy speech signal에서 background noise를 preserving 하면서 speech를 removing 하는 것으로 목표로 함
- 즉, recording에서 unwanted speech를 remove 함 - 해당 task를 위해 SpeechX는
special token을 사용하여 acoustic prompt<sr> 을 구성함A=[<sr>,C(s+n)] - 결과적으로 Speech Removal task의 desired output은 noise signal의 acoustic token sequence
이 됨C(n)
- 이때 noise suppression과 마찬가지로 textual prompt를 omit 할 수 있음
- Speech Removal은 noisy speech signal에서 background noise를 preserving 하면서 speech를 removing 하는 것으로 목표로 함
- Target Speaker Extraction
- Target Speaker Extraction은
과 secondary speaker의 interfering speechs1 간의 mixture에서 target speaker의 clean speechs2 을 isolating 하는 것을 목표로 함s1
- 여기서 target speaker는 short enrollment audio 을 통해 identify 되고 3초의 enrollment를 가정s′1 - 해당 task의 경우 enrollment audio에서 추출한 acoustic token
과 mixed speech의 acoustic tokenC(s′1) 를 task-specifying tokenC(s1+s2) 와 concatenating 하여 acoustic prompt를 구성함<tse>
-A=[C(s′1),<tse>,C(s1+s2)] - 결과적으로 Target Speaker Extraction의 desired output은
이 됨C(s1)
- 마찬가지로 textual prompt는 optional 하게 선택할 수 있음
- Target Speaker Extraction은
- Zero-Shot TTS
- Zero-shot TTS는 input text와 enrollment speech
를 사용하여 speech signals 을 생성하는 것을 목표로 함s′
- 이때 의 speech characteristic은s′ 와 resemble 하면서 input text를 accurately reflecting 해야 함s - 해당 task의 경우, enrollment audio에서 추출한 acoustic token
를 acoustic prompt로 사용함C(s) - 결과적으로 SpeechX는 input text를 기반으로 synthesized speech에 대한 acoustic token
을 생성하고, 해당 acoustic token을 waveform으로 변환함C(s′)
- Zero-shot TTS는 input text와 enrollment speech
- Clean Speech Editing
- Clean Speech Editing은 input speech의 segment를 수정하여 input text에 align 하는 것을 목표로 함
- 먼저 edit 할 input speech signal을
라고 하자s
- 그러면 를s 의 3 부분으로 나누고,spre,smid,spost 를 editing을 위한 target segment로 사용함smid
- 여기서 는 empty일 수 있음spre,spost - 이를 기반으로 acoustic prompt를
와 같이 구성함[C(spre,<soe>,<mask>,<eoe>,C(spost)]
- Token 는 task와 editing segment를 지정하는 데 사용됨<soe>,<mask>,<eoe> - 결과적으로 desired output은 neural code sequence
가 되고,[C(spre),C(sedit),C(spost)] 의 spoken content는 input text와 match 되어야 함[spre,sedit,spost]
- 추가적으로 의 speaker characteristic은sedit 와 consistent 해야 함spre,spost
- Noisy Speech Editing
- Noisy Speech Editing은 noisy speech를 input으로 하여 background noise를 유지하면서 segment 내의 speech content를 수정하는 것을 목표로 함
- 즉, model은 editing 중에 speech와 noise를 distinguish 할 수 있어야 함 - 따라서 논문은
token으로 segment를 mask 하지 않고 model에 complete input speech signal을 제공함<mask> - 이를 기반으로 acoustic prompt는
와 같이 구성됨[C(spre+npre),<soe>,C(smid+nmid),<eoe>,C(spost+npost)] - 결과적으로 desired output은 neural code sequence
가 됨[C(spre+npre),C(sedit+nmid),C(spost+npost)]
- 해당 formulation은 model이 를 유지하면서 text input을 기반으로nmid 를smid 로 변환하는 것을 의미함sedit
- Noisy Speech Editing은 noisy speech를 input으로 하여 background noise를 유지하면서 segment 내의 speech content를 수정하는 것을 목표로 함
- Practical speech editing scenario에서 input text는 input speech에 Automatic Speech Recognition을 적용한 다음, transcription을 editing하여 수행됨
- 이 경우,
를 insert하는 position을 identify하는 것이 쉬움<soe>,<eoe> - 특히 clean speech editing에서
를 사용하면 model이 output speech length를 adaptively change하여 speaking speed 측면에서 natural sound를 만들 수 있음<mask>
- 이 경우,
- 결과적으로 이러한 task-based prompting은 SpeechX의 추론 중에 desired outptu을 uniquely decide할 수 있는 ability를 제공함
- 특히 해당 방식을 통해 additional task incorporating에 대한 flexibility를 제공할 수 있음
- 즉, 새로운 task를 추가하는 경우 해당 prompting shceme을 integrating한 다음 기존 checkpoint에서 training을 수행하면 됨
- 이는 model architecture의 변경 없이 새로 추가된 task-specific token에 대한 embedding만 randomly initialize하여 수행될 수 있음
- Noise Suppression

- Model Training
- Training 중에 각 model update에 대해 equal probability로 task를 random sampling하여 model이 특정 task를 unduly favor하지 않도록 함
- Noise suppression, speech removal, target speaker extraction의 경우, textual prompt를 50% 확률로 포함하므로 model은 text/text-less scenario를 equally experience할 수 있음
- Multi-task learning은 먼저 model을 zero-shot TTS에 대해 training한 다음 수행됨
- 즉, 기존 VALL-E checkpoint로 model을 initialize한 다음
- Multi-task training stage 시작 시 task-dependent prompt와 관련된 special token에 대해 randomly initialized embedding을 append함
- 해당 two-stage training strategy를 통해 SpeechX는 모든 task에 대한 성능을 크게 향상할 수 있음
3. Experiments
- Settings
- Dataset : LibriLight, DNS Challenge
- Comparisons
- Zero-shot TTS : VALL-E
- Speech Editing : A3T
- Speaker Extraction : VoiceFilter
- Noise Suppression : DCCRNN
- Results
- Result Overview
- 각 task별로 SpeechX가 가장 뛰어난 성능을 보임

- Speech Editing
- Spectrogram 측면에서 SpeechX는 speaker identity와 background noise를 maintain하면서 효과적인 noisy speech editing이 가능함
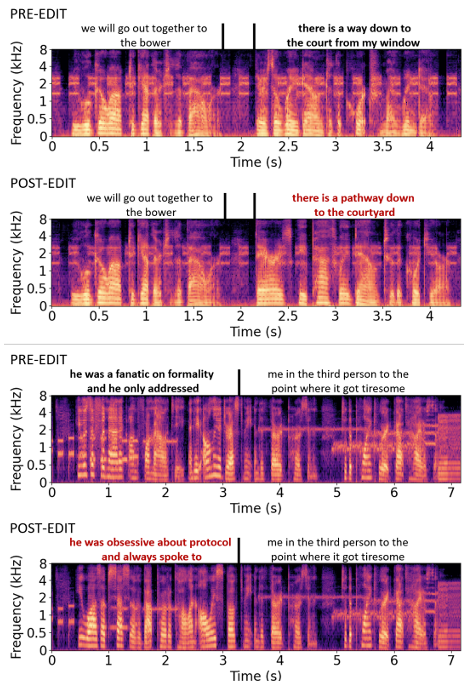
- Noise Suppression and Target Speaker Extraction
- Prompt를 추가하는 경우 noise suppression과 target speaker extraction의 성능을 향상할 수 있음
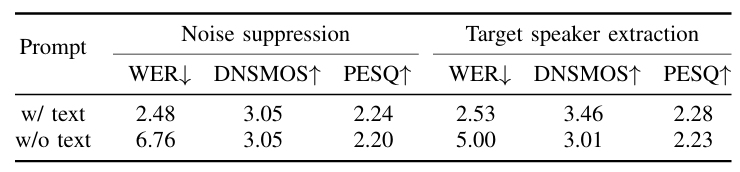
- Effect of Multi-Task Training
- Multi-task training은 zero-shot TTS에서 WER을 개선하고 additional task를 처리할 수 있는 ability를 제공함
- 즉, model을 다양한 data에 exposing하면 single task performance를 개선할 수 있음

- 추가적으로 task subset을 활용하여 다른 task 간의 potential interaction을 확인해보면
- Training 중에 speech editing을 포함하는 경우 zero-shot TTS에 대한 WER이 향상됨
- Training 중에 noise suppression과 speech removal을 포함하는 경우 noisy speech editing 성능이 개선됨

- Phoneme vs. Byte Pair Encoding (BPE)
- BPE-based SpeechX는 noise suppression, target speaker extraction에서 더 나은 성능을 보임
- Phoneme-based SpeechX는 zero-shot TTS, speech editing에서 더 나은 성능을 보임

- Limitation of Current Neural Codec Model
- SpeechX의 성능은 acoustic tokenization을 위해 사용되는 neural codec model의 accuracy에 의해 제한됨
- 따라서 neural codec model인 EnCodec의 영향을 추가적으로 확인해보면
- Codec model로 signal을 처리하는 경우 대부분의 task에서 약간의 성능 저하가 발생함
- 이는 PESQ metric과 EnCodec training objective 간의 mismatch 때문
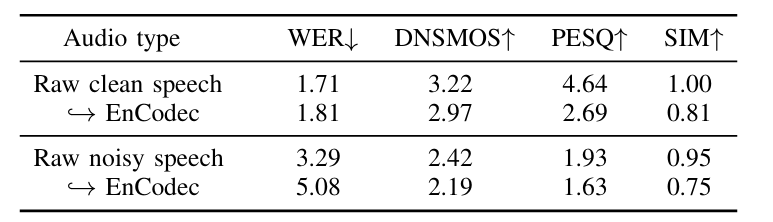
반응형