티스토리 뷰
Paper/TTS
[Paper 리뷰] FastSpeech: Fast, Robust and Controllable Text to Speech
feVeRin 2023. 7. 23. 11:56반응형
FastSpeech: Fast, Robust and Controllable Text to Speech
- 기존의 Text-to-Speech (TTS) 모델은 text에서 mel-spectrogram을 생성한 다음, WaveNet과 같은 vocoder를 사용해 mel-spectrogram에서 음성을 합성함
- End-to-end TTS 모델은 추론 속도가 느리고 합성된 음성이 robust 하지 않고, controllability (voice speed, prosody control)가 떨어짐
- FastSpeech
- Mel-spectrogram을 병렬로 생성하는 transformer 기반 feed-forward network
- Phoneme duration 예측을 위해 encoder-decoder 기반 teacher 모델에서 attention alignment를 추출
- 논문 (NeurIPS 2019) : Paper Link
- 해당 논문의 개선 버전 : FastSpeech2 리뷰
1. Introduction
- Tacotron, Deep Voice 등과 같은 기존 TTS 모델은 autoregressive 하게 mel-spectrogram을 생성한 다음, vocoder를 사용하여 mel-spectrogram에서 음성을 합성함
- 긴 mel-spectrogram 시퀀스와 autoregressive 특성으로 인해 기존 TTS 모델은 한계점이 존재
- Mel-spectrogram 생성으로 인한 느린 추론 속도
- CNN, Transformer 기반 모델은 RNN 기반 모델보다 추론 속도를 빠르게 할 수 있음
- 일반적으로 mel-spectrogram 시퀀스의 길이는 수백~수천이므로 여전히 추론 속도가 느림 - 합성된 음성은 robust하지 않음
- Autoregressive 생성 단계에서의 error propagation, 잘못된 attention alignment로 인해 생성된 mel-spectrogram은 word skipping, repeating 문제를 보임 - 합성된 음성은 controllability가 부족함
- Autoregressive 모델은 text와 음성 사이의 alignment를 명시적으로 활용하지 않고 mel-spectrogram을 하나씩 생성함
- Voice speed, prosody를 control 하기 어려움
- Mel-spectrogram 생성으로 인한 느린 추론 속도
-> 그래서 mel-spectrogram 생성 속도를 높이고, text와 음성 사이의 alignment를 고려한 non-autoregressive TTS 모델인 FastSpeech를 제안
- FastSpeech
- Transformer의 self-attention, 1D convolution에 기반한 feed-forward network
- Mel-spectrogram 시퀀스와 phoneme 시퀀스 간의 불일치 문제를 해결하는 length regulator
- Phoneme duration에 따라 phoneme 시퀀스를 upsampling 하여 mel-spectrogram 길이와 일치시킴
- 각 phoneme의 duration을 예측하는 duration predictor를 기반으로 함
< Overall of FastSpeech >
- 병렬 mel-spectrogram 생성을 통해 음성 합성 속도를 높임
- Phoneme과 그에 대응하는 mel-spectrogram 간의 hard alignment를 보장하는 phoneme duration predictor
- Voice speed와 prosody를 control 할 수 있는 length regulator
2. FastSpeech
- Feed-Forward Transformer
- Transformer의 self-attention, 1D convolution에 기반한 feed-forward 구조
- Feed-Forward Transformer (FFT)
- Phoneme에서 mel-spectrogram 변환을 위해 여러개의 FFT 블록을 stack 함
- Phoneme 쪽에
개의 블록, mel-spectrogram 쪽에N 개의 블록이 사용N
- Phoneme과 mel-spectrogram 시퀀스 사이의 length gap을 length regulator로 연결 - Self-attention network는 cross-position 정보를 추출하기 위한 multi-head attention으로 구성
- ReLU activation을 포함한 2-layer 1D convolution network의 사용
- 기존 transformer에서 2-layer Dense network를 대체
- Adjacent hidden state가 character / phoneme 및 mel-spectrogram 시퀀스에 더 밀접하게 관련되어 있다는 점을 이용
- Transformer에서 residual connection, layer normalization, dropuout이 self-attention 및 1D convolution network 다음에 추가됨
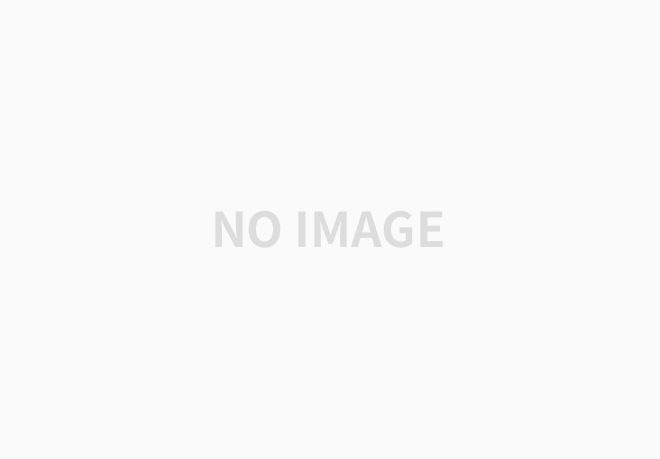
- Length Regulator
- FFT에서 phoneme과 spectrogram 시퀀스 간의 불일치 문제를 해결하고 voice speed와 prosody를 control 하는 역할
- Phoneme 시퀀스의 길이는 mel-spectrogram 시퀀스 보다 짧고 각 phoneme은 여러 mel-spectrogram에 해당할 수 있음
- Phoneme Duration : phoneme에 해당하는 mel-spectrogram의 길이
- Length Regulator
- Phoneme duration
를 기준으로 length regulator는 phoneme 시퀀스의 hidden state를d 배 확장하여 hidden state의 길이와 mel-spectrogram의 길이를 맞춤d - Phoneme 시퀀스의 hidden state :
Hpho=[h1,h2,...,hn]
- : 시퀀스 길이n - Phoneme duration 시퀀스 :
D=[d1,d2,...,dn]
-∑ni=1di=m
- : mel-spectrogram 시퀀스의 길이m - Length Regulator (
) :LR Hmel=LR(Hpho,D,α)
- : 확장된 시퀀스α 의 길이를 결정해 voice speed를 조절하는 hyperparameterHmel
- Phoneme duration
- For example,
, phoneme duration 시퀀스Hpho=[h1,h2,h3,h4] 인 경우,D=[2,2,3,1] - Noraml speed (
)α=1
: 확장 시퀀스Hmel=[h1,h1,h2,h2,h3,h3,h3,h4] - Slow speed (
)α=1.3
: duration 시퀀스Dα=1.3=[2.6,2.6,3.9,1.3]≈[3,3,4,1]
-> 확장 시퀀스Hmel=[h1,h1,h1,h2,h2,h2,h3,h3,h3,h3,h4] - Fast speed (
)α=0.5
: duration 시퀀스Dα=0.5=[1,1,1.5,0.5]≈[1,1,2,1]
-> 확장 시퀀스Hmel=[h1,h2,h3,h3,h4]
- Noraml speed (
- 합성된 음성의 prosody를 조정하기 위해 문장에서 space character의 duration을 조정하여 단어 사이의 끊김을 control 할 수 있음
- Duration Predictor
- Phoneme duration 예측은 length regulator에서 중요한 부분
- Length regulator는 ReLU activation이 있는 2-layer 1D convolution network로 구성됨
- 각 layer에는 layer noramlization, dropout이 사용되고 예측된 phoneme duration의 scalar output을 위한 linear layer가 존재 - Phoneme 쪽의 FFT 블록 위쪽에 stack 되어 각 phoneme에 대한 mel-spectrogram 길이 예측을 위해 FastSpeech 모델과 함께 Mean Squared Error (MSE) loss로 학습됨
- Logarithmic domain에서 길이를 예측하여 Gaussian처럼 만들어 쉽게 학습되도록 함 - 학습된 duration predictor는 TTS 추론 단계에서만 사용됨
- Autoregressive teacher 모델에서 추출한 phoneme duration을 사용할 수도 있음
- Length regulator는 ReLU activation이 있는 2-layer 1D convolution network로 구성됨
- Autoregressive teacher TTS 모델에서 ground-truth phoneme duration을 추출하여 duration predictor 학습하기
- Autoregressive encoder-attention-decoder 기반 Transformer TTS 모델 학습
- 각 training 시퀀스 pair에 대해 학습된 teacher 모델에서 decoder-to-encoder attention alignment 추출
- Multi-head attention으로 인해 multiple attention alignment가 존재하고, 모든 attention head가 diagonal property를 나타내지는 않음 (phoneme과 mel-spectrogram 시퀀스가 monotonic 하게 align 됨)
- Attention head가 diagonal 한 지 판별하기 위해 focus rate
를 통해 측정F
:F=1S∑Ss=1max1≤t≤T as,t
- : 각각 ground-truth spectrogram과 phoneme 길이S,T
- : attention matrix의as,t 번째 row와s 번째 column의 elementt - 각 head에 대해 focus rate를 계산하여 가장 큰
값을 가지는 head를 attention alignment로 사용F
- Duration extractor
에 따라 phoneme duration 시퀀스di=∑Ss=1[argmaxt as,t=i] 추출D=[d1,d2,...,dn]
- Phoneme duration은 선택한 attention head를 따라 attend 되는 mel-spectrogram의 개수
3. Experiments
- Settings
- Dataset : LJSpeech
- Comparisions : Tacotron 2, Transformer TTS, Merlin
- Results
- Audio Quality
- Transformer TTS, Tacotron 2와 비슷한 음성 합성 품질을 달성
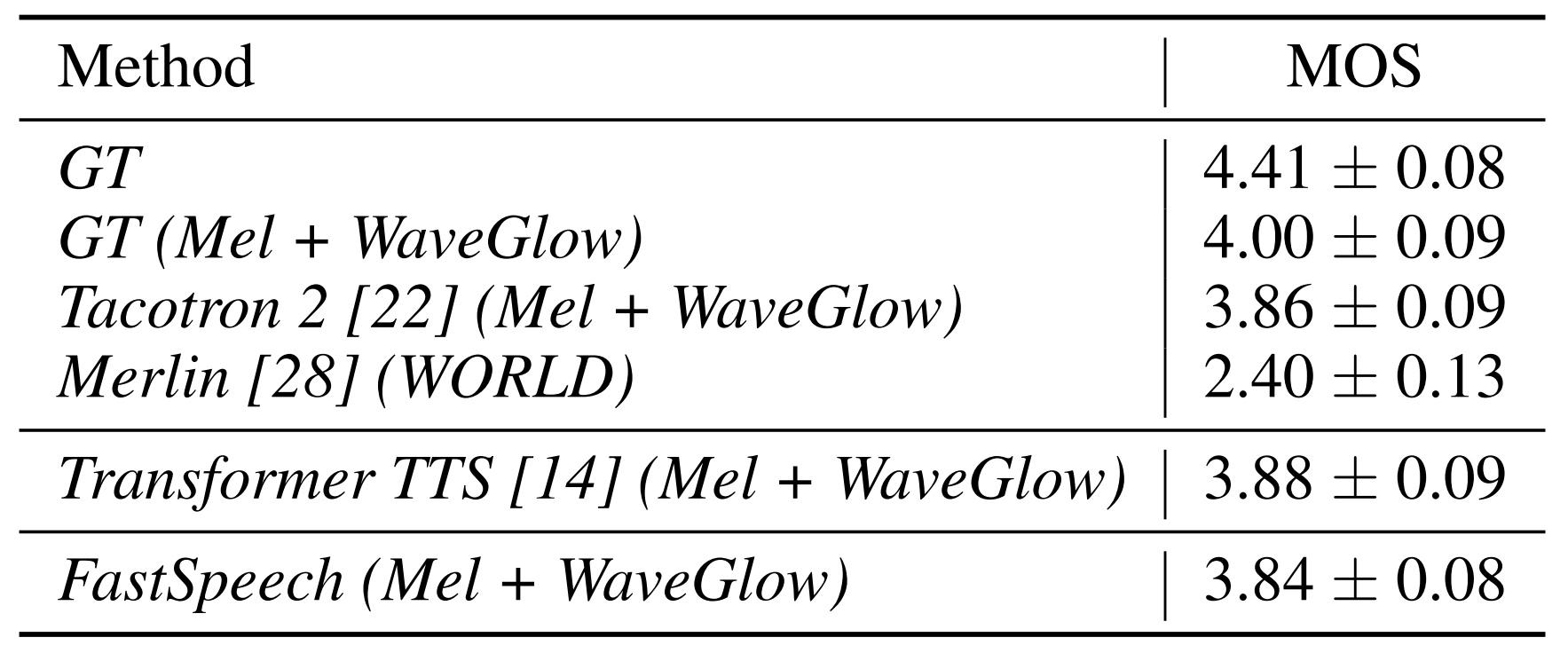
- Inference Speedup
- FastSpeech와 비슷한 크기의 parameter를 가지는 autoregressive Transformer TTS와 비교
- Mel-spectrogram 생성 속도 269.40배 향상
- 오디오 생성 속도 38.30배 향상
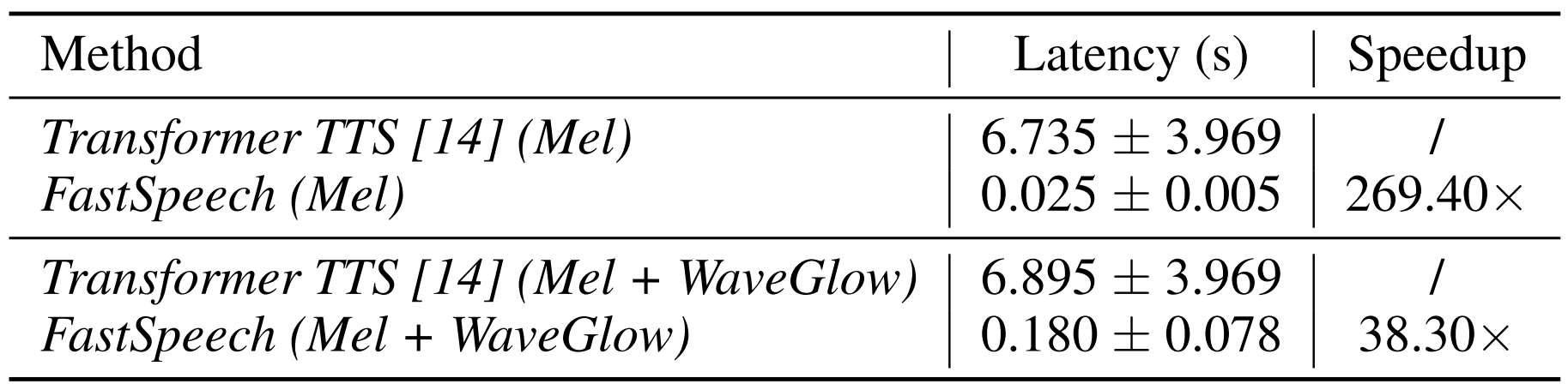
- 추론 latency와 예측된 mel-spectrogram 사이의 관계
- FastSpeech는 예측된 mel-spectrogram 길이에 따라 추론 latency가 거의 증가하지 않음
- Transformer TTS는 mel-spectrogram 길이가 길어질수록 latency도 같이 증가
-> FastSpeech는 생성된 오디오 길이에 민감하지 않음
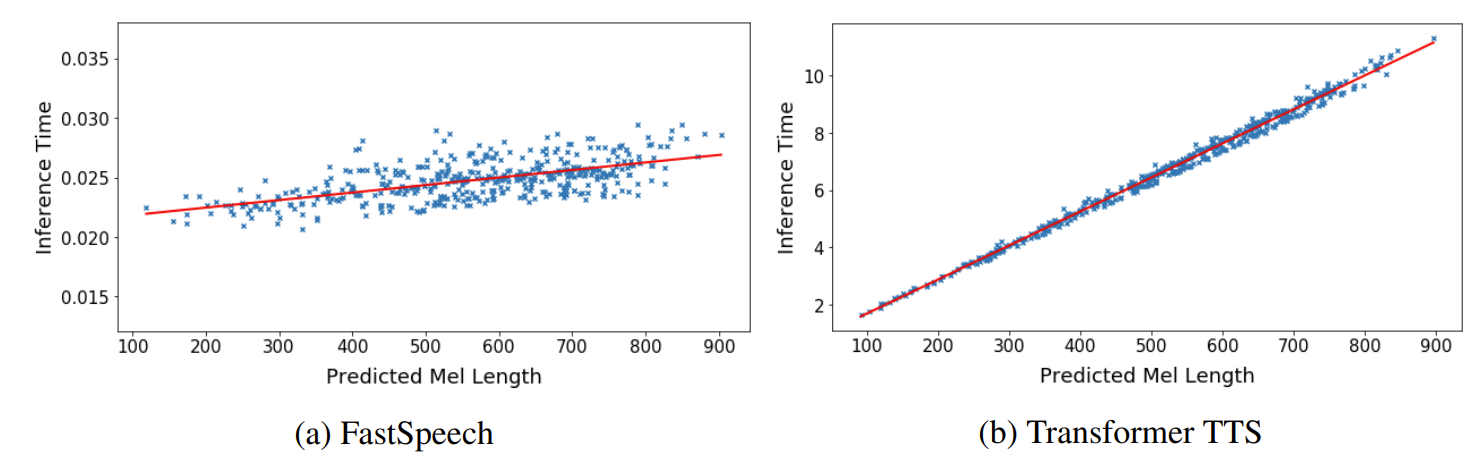
- Length Control
- FastSpeech는 phoneme duration을 조정하여 prosody와 voice speed를 조정할 수 있음
- Length 조절 전후의 mel-spectrogram을 비교 - Voice Speed
- FastSpeech는 voice speed를 0.5~1.5까지 안정적인 pitch로 변환할 수 있음 - Breaks Between Words
- FastSpeech는 문장에서 space character의 duration을 늘려 adjacent word 간의 break를 조절해 voice prosody를 개선할 수 있음
- FastSpeech는 phoneme duration을 조정하여 prosody와 voice speed를 조정할 수 있음
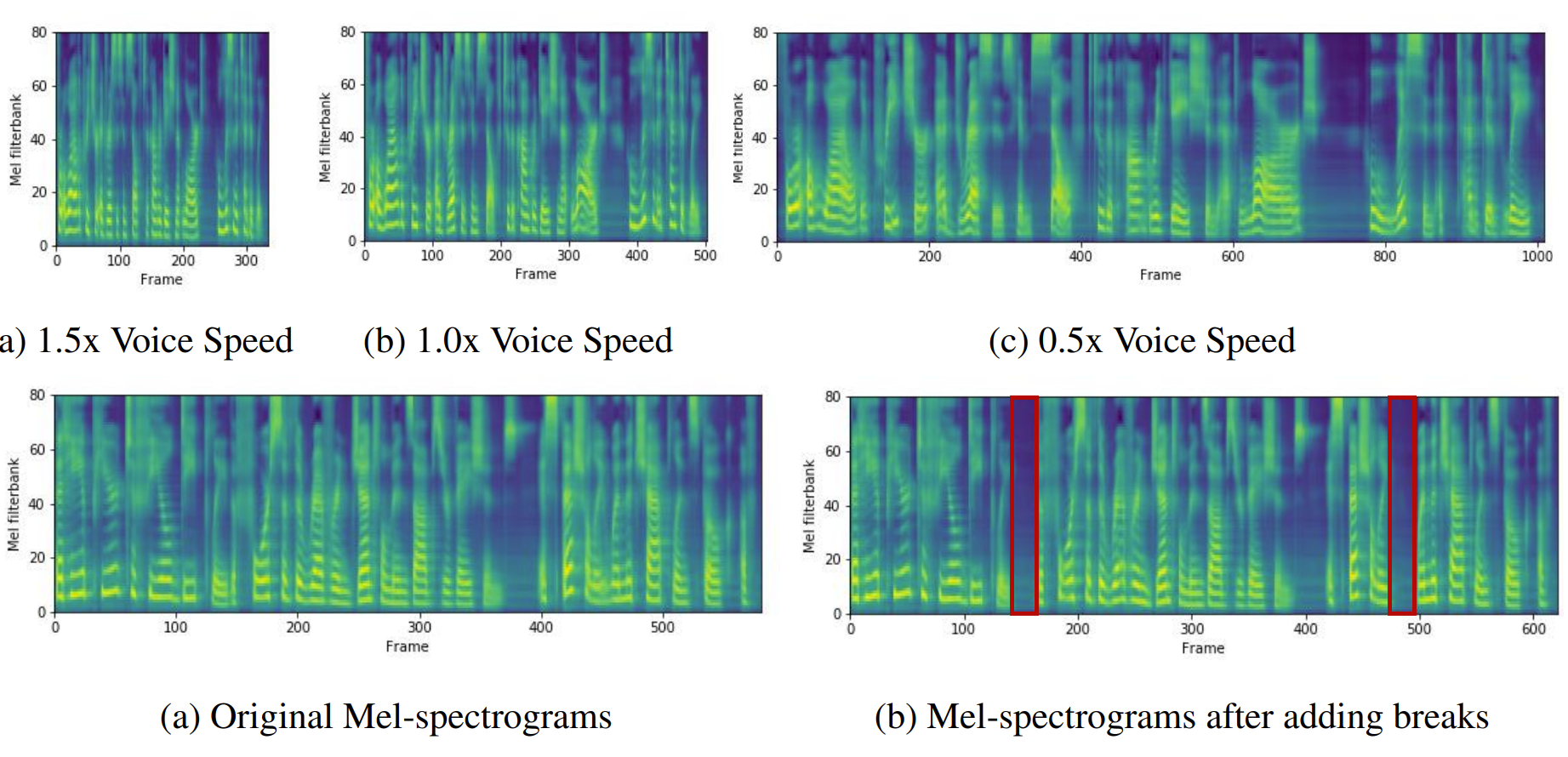
- Ablation Study
- FastSpeech에서 1D convolution과 Sequence-level Knowledge Distillation 효과 비교
- 1D convolution in FFT
- FFT는 기존의 fully-connected layer 대신 1D convolution을 사용함
- 1D convolution 대신 fully-connected layer를 사용한 경우, -0.113 CMOS를 달성해 성능 저하를 보임 - Sequence-level Knowledge Distillation
- Knowledge distillation을 사용하지 않은 경우, -0.325 CMOS를 달성해 성능 저하를 보임

반응형