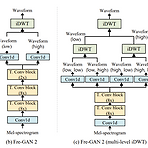
Fre-GAN 2: Fast and Efficient Frequency-Consistent Audio Synthesis 대규모의 TTS 모델은 resource가 제한된 device에 적용하기 어려우므로 neural vocoder는 효율적이면서도 고품질의 합성이 가능해야 함 Fre-GAN 2 Audio의 low/high-frequency에서 합성을 수행하고, inverse discrete wavelet transform을 통해 target-resolution audio를 reproduce 적은 수의 parameter 만으로 고품질의 audio를 합성할 수 있도록 adversarial periodic feature distillation을 도입 논문 (ICASSP 2022) : Paper Link 1. In..
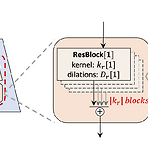
HiFi-GAN: Generative Adversarial Networks for Efficient and High Fidelity Speech Synthesis Generative Adversarial Network (GAN)을 활용한 음성 합성은 autoregressive에 비해 낮은 품질을 보임 하지만 GAN을 활용하면 sampling과 메모리 효율성을 향상할 수 있음 HiFi-GAN 다양한 period를 가지는 sinusoidal pattern을 모델링 Autoregressive 모델보다 더 빠르고 고품질의 음성을 합성 논문 (NeurIPS 2020) : Paper Link 1. Introduction 대부분의 음성 합성 모델은 two-stage 구조를 가짐 Text로 부터 mel-spectrog..
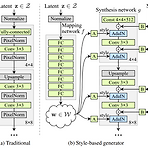
StyleGAN: A Style-Based Generator Architecture for Generative Adversarial Networks Style transfer의 개념을 빌린 Generative Adversarial Network (GAN)을 위한 generator architecture High level attributes와 stochastic variation에 대한 unsupervised separation을 학습하여 이미지 합성에 대한 scale-specific control을 제공 StyleGAN 기존의 distribution quality metric에 대해 SOTA 성능을 달성 더 나은 interpolation property 및 latent factor variation에 ..
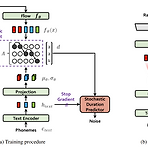
Conditional Variational Autoencoder with Adversarial Learning for End-to-End Text-to-Speech Single-stage 학습을 가능하게 하는 end-to-end 방식의 text-to-speech (TTS) 모델이 제안되었지만 여전히 two-stage TTS 모델들보다 음성 품질이 낮음 Two-stage TTS 모델보다 더 자연스러운 음성을 생성하는 병렬 end-to-end TTS 모델이 필요 VITS (Variational Inference with adversarial learning for end-to-end Text-to-Speech) Normalizing flow와 적대적 학습 방식을 사용한 variational 추론을 통한 생성..