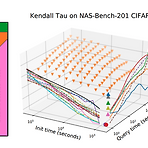
How Powerful Are Performance Predictors in Neural Architecture Search? Neural Architecture Search (NAS)의 계산 비용을 줄이기 위해서 neural architecture의 성능을 예측하는 방식이 제안됨 Performance predictor는 NAS에서 효과적이지만, 최적화된 Initialization time, Query time 설정과 합의된 평가 지표의 부족으로 다양한 Performance predictor들 간의 효과적인 비교가 어려움 여러 Performance predictor들에 대한 대규모 연구 결과 제시 Learning curve extrapolation, Weight-sharing, Supervised lea..
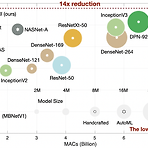
Once-For-All: Train One Network and Specialize it for Efficient Deployment 다양한 edge device에서 자원 제약을 만족하는 효율적인 inference의 어려움 Once-For-All (OFA) training / search를 분리하여 cost를 줄임 OFA에서 추가적인 training 없이 sub-network를 얻을 수 있음 Progressive Shrinking OFA network training을 위해 제안 -> depth/width/kernel/resolution pruning 논문 (ICRL 2020) : Paper Link OFA의 개선버전 : CompOFA 리뷰 1. Introduction model size의 증가는 har..
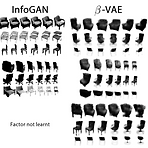
beta-VAE: Learning Basic Visual Concepts with a Constrained Variational Framework Supervision 없이 독립적인 data의 interpretable factorised representation을 학습하는 것이 중요 $\beta$-VAE (beta-VAE) Unsupervised 방식으로 raw image data에서 interpretable factorised latent representation을 발견 가능 adjustable parameter $\beta$ : latent channel capacity와 재구성 정확도의 independence 제약조건 조절 $\beta$-VAE는 train이 stable하고, data에 대한 가..